Tao Liu, Hao Li, Tao He, Cunzheng Fan, Zhijun Yan, Deming Liu, Qizhen Sun. Ultra-high resolution strain sensor network assisted with an LS-SVM based hysteresis model[J]. Opto-Electronic Advances, 2021, 4(5): 200037-1

Search by keywords or author
- Opto-Electronic Advances
- Vol. 4, Issue 5, 200037-1 (2021)
Abstract
(1) |
View in Article
(2) |
View in Article
(3) |
View in Article
(4) |
View in Article
(5) |
View in Article
(6) |
View in Article
(7) |
View in Article
(8) |
View in Article
(9) |
View in Article
(10) |
View in Article
(11) |
View in Article
(12) |
View in Article
(13) |
View in Article
(14) |
View in Article
(15) |
View in Article
(16) |
View in Article
(17) |
View in Article
(18) |
View in Article
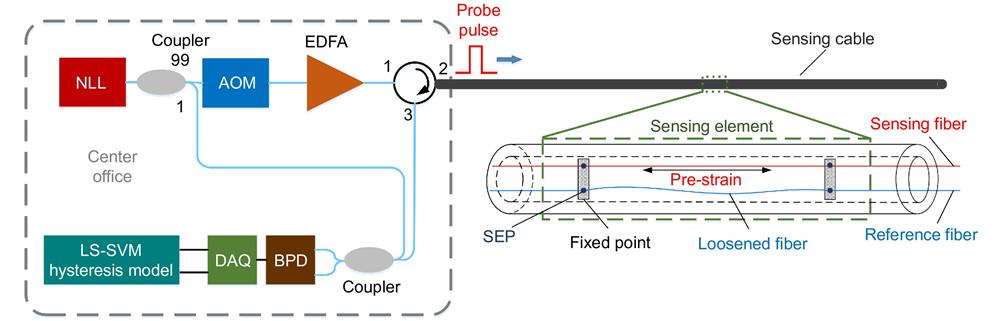
Set citation alerts for the article
Please enter your email address