
- Photonics Research
- Vol. 10, Issue 1, 120 (2022)
Abstract
1. INTRODUCTION
Histopathological examination of formalin-fixed paraffin-embedded (FFPE) section remains the gold standard in evaluating neoplasms and other diseases. However, the current clinical workflow often requires hours or even days to provide a reliable diagnosis [1]. A series of time-consuming and laborious tissue processing steps are necessary to prepare high-quality thin tissue slices. Although frozen section is the current intraoperative histology examination alternative (20–30 min), its freezing artefacts resulting from sectioning frozen tissue, especially in adipose tissue [2], are still highly unsatisfactory. This affects the reliability of the frozen section. In conventional brightfield microscopy, which has been widely adopted in histopathology laboratories, thick tissue imaging remains a challenge since light scattering from biomolecules at multiple depths significantly degrades image contrast. Therefore, sectioning thick tissue specimens into thin tissue slices physically is necessary. However, getting thin tissue slices not only requires the use of costly and specialized machines but also prolongs the assessment, potentially delaying treatments for patients. Thus, there is a great demand to develop a rapid, slide-free, and reliable imaging technique for intraoperative histology.
Various techniques have been proposed to achieve rapid diagnosis on unprocessed thick tissues. For instance, optical coherence tomography and confocal reflectance microscopy have been demonstrated as the label-free imaging techniques for the diagnosis of breast [3] and skin cancer [4], respectively. Yet, their intrinsic scattering contrast is not suitable for probing specific molecular targets.
Other fluorescent labeling imaging alternatives, for example, confocal fluorescence microscopy [5] and multiphoton microscopy [6], have also been demonstrated in histopathology applications. However, their inherent point-scanning mechanism requires a high-repetition-rate laser and complex scanning system to achieve high imaging speed. Moreover, the associated high cost of a high-peak-power laser for the generation of the nonlinear effect further makes multiphoton microscopy less favorable in clinical settings [7].
Sign up for Photonics Research TOC. Get the latest issue of Photonics Research delivered right to you!Sign up now
Microscopy with ultraviolet surface excitation (MUSE) [8] has recently been demonstrated as a simple and cost-effective surface imaging technique for biological tissues. MUSE utilizes the short penetration depth of deep ultraviolet (UV) light and the limited diffusion of fluorescent stains to confine the excitation of fluorophores only on the tissue surface. Sharing the advantage of wide-field microscopy, MUSE only requires a UV light-emitting diode (LED) as a light source to provide high imaging speed, eliminating the need of any high-repetition-rate laser as in point scanning approaches, which is highly desirable for clinical applications. MUSE also has its strength in providing a broad color palette for studying specific structural identities by utilizing both UV-excitable endogenous and exogenous fluorophores. However, one limiting factor of UV surface imaging is the tissue-dependent property of UV penetration depth, which defines the optical sectioning thickness. It has been shown that the UV penetration depth is
In histopathology, although 2×–4× magnifications are sometimes sufficient for making a decision in surgical margin analysis [12], 20×–40× magnifications [with a numerical aperture
To address the aforementioned challenges of short DOF, we first incorporate MUSE with speckle illumination, termed MUSES, which allows previously missed high-frequency components to fall into the passband of the imaging system in the Fourier domain. Followed by an iterative reconstruction algorithm, the high-spatial-frequency components can be retrieved and synthesize a larger passband, thus improving the lateral resolution. The problem of short DOF is addressed in two aspects: (1) preserving the long DOF with the use of a low-NA objective lens and (2) simultaneously reducing the optical sectioning thickness by oblique illumination. In MUSES, we also implement a color transformation algorithm via deep learning to demonstrate the effectiveness of MUSES in histological imaging. With these implementations, we aim at providing a better imaging contrast for UV-surface excitation in highly scattering organs, relieving the constraint in tissue flatness and encouraging the use of a common blade to obviate the lengthy thin tissue slice preparation, thus accelerating the clinical histological workflow.
2. METHODS
A. Super-Resolution Fluorescence Imaging by Pattern Illumination
In diffraction-limited microscopes, there is a fundamental trade-off between DOF and lateral resolution. To circumvent this trade-off, we employ a pattern illumination scheme using a low-NA objective lens to achieve resolution beyond the diffraction limit, while preserving the long DOF for sharp thick tissue imaging. Structured illumination with different patterns (e.g., sinusoidal stripe [14] and speckle pattern [15]) have been reported to improve spatial resolution by synthesizing a large effective aperture. By illuminating with a high-spatial-frequency pattern, intensity modulation is introduced to the fluorescence sample. This modulation allows high-spatial-frequency information beyond the diffraction limit to be encoded into low-spatial-frequency information, which can then be captured. The effective NA (
In a typical implementation of linear structured illumination microscopy, the resolution gain is limited by a factor of 2 with an epi-illumination configuration that uses the same objective lens for illumination and detection. To go beyond this limit, we have adopted an oblique pattern illumination configuration [Fig. 1(a)] for two reasons: (1) the illumination and detection paths can be separated and hence achieve a resolution gain
Figure 1.(a) System configuration of MUSES. (b) An iterative algorithm for the R, G, and B channels.
A condenser lens with a focal length of 50 mm (LA4148-UV, Thorlabs Inc.) was used to focus the speckle pattern on the sample plane with a sufficiently large illumination area and working distance to be accommodated in the system. Images were acquired under an inverted microscope configuration which consists of a 4× plan achromat objective lens (RMS4X,
To reconstruct a color high-resolution (HR)-MUSES image, the raw sequence of the speckle-illuminated MUSES images was split into R, G, and B channels. The reconstruction was performed in each channel, respectively, based on the framework in Ref. [19]. A momentum-accelerated ptychographical iterative engine [20] was adopted, which allows quick update and regularization when the acquired image is susceptible to noise, enabling high robustness to changes in frame intensities due to photobleaching. The reconstructed images for each channel were then merged back into a color HR-MUSES image. In the following, the performance of HR-MUSES using a 4× objective lens (termed 4X MUSES hereafter) is compared with MUSE images acquired using 4× and 10× objective lenses (termed 4X MUSE and 10X MUSE, respectively, hereafter) under UV-LED illumination (M265L4, Thorlabs Inc.).
B. Color Transformation via Deep Learning
To show the effectiveness of MUSES in histological imaging, we have employed an unsupervised deep-learning method to transform the color style of MUSES images into standard hematoxylin and eosin (H&E)-stained images virtually. There has been a growing use of deep-learning approaches in different areas of computational imaging, such as super-resolution, denoising, and color transformation [21–23]. While model-based pseudocolor approaches [24,25] have been commonly applied to simulate H&E-stained images, we adopted a data-driven deep-learning approach for color transformation since it can also potentially improve resolution and contrast through deconvolution [26], which can further improve the image quality. An unpaired image-to-image translation method called cycle-consistent generative adversarial network (CycleGAN) [27] was adopted. Its unpaired nature is particularly important for thick tissue image style transformation, in which paired MUSES and H&E-stained images are impossible to obtain. To better preserve the histological features and perceptual quality, including nuclear size, number of nuclei, and H&E color style, during transformation, cycle-consistency and structural similarity losses were added to the final objective loss function together with the common GAN and L1 losses to train the thick MUSES image with the adjacent FFPE H&E-stained images based on the framework in Ref. [28]. 1368 10X MUSE image patches and 2575 H&E-stained image patches with a patch size of
3. RESULTS
A. Imaging Performance of MUSES Verified by Fluorescent Beads
The MUSES system performance in resolution improvement was verified by imaging blue fluorescence beads with a diameter of 500 nm (B500, excitation/emission: 365/445 nm, Thermo Fisher Scientific Inc.). 4X MUSE, 4X MUSES, and 10X MUSE images of the fluorescent beads are compared in Figs. 2(a)–2(c). By measuring the full width at half-maximum of the Gaussian-fitted line profiles of the beads, the achievable lateral resolutions of the 4X MUSES system in G- and B-channels are 1.02 μm and 1.01 μm, respectively. An average of 2.4 times resolution gain was demonstrated by 4X MUSES over 4X MUSE images (averaged line profiles of 10 fluorescent beads). Due to the random nature of the speckle, a speckle pattern with speckle size ranging from 1.4 to 2.8 μm (validated by measuring line profiles of several speckles on a fluorescent plate) was generated, corresponding to an illumination NA
Figure 2.(a)–(c) Comparison of 4X MUSE, 4X MUSES, and 10X MUSE images of blue fluorescent beads with a diameter of 500 nm. (d) and (e), (f) and (g), (h) and (i) Zoomed-in images of the bead inside the yellow dashed boxes in G- and B-channels under 4X MUSE, 4X MUSES, and 10X MUSE images, respectively. (j), (k) The corresponding line profiles in G- and B-channels of this bead under 4X MUSE (orange line) and 4X MUSES (purple line).
B. Histological Images of FFPE Slides Provided by MUSES
To evaluate the performance of MUSES on biological samples, we first tested on a 7 μm FFPE thin slice of a mouse brain that had been stained with a mixture of Rhodamine B (500 μg/mL) and Hoechst 33342 (500 μg/mL) in phosphate-buffered saline for 10 s, which was then washed with water and mounted on a UV-transparent quartz slide before MUSES imaging [Fig. 3(a)]. In the hippocampus region with dense cell nuclei, resolution improvement is clearly observed in 4X MUSES images [Figs. 3(d), 3(g), and 3(j)] when compared with their corresponding 4X MUSE images [Figs. 3(c), 3(f), and 3(i)]. After MUSES imaging, the same tissue slice was destained with deionized water, followed by a few drops of acid-alcohol solution, and subsequently stained with H&E. A whole-slide scanner with a 20× objective lens (
Figure 3.(a) 4X MUSE image of an FFPE mouse brain tissue slice that is stained with Rhodamine B and Hoechst 33342. (b) Corresponding H&E-stained FFPE slice. (c)–(e) Zoomed-in images of 4X MUSE, 4X MUSES, and corresponding H&E slice of the hippocampus region marked with an orange solid box in (a) and (b). (f)–(h) Zoomed-in images that correspond to the yellow dashed box regions marked in (c), (d), and (e), respectively. (i)–(k) Zoomed-in images that correspond to the blue dotted box regions marked in (c), (d), and (e), respectively.
C. Histological Images of Fixed Thick Tissue Provided by MUSES
Then we further tested a 3 mm thick mouse brain tissue with prior formalin fixation (Fig. 4). An adjacent FFPE thin slice was prepared for validation. Resolution improvement is also observed when comparing 4X MUSE [Figs. 4(b), 4(e), 4(h)] with 4X MUSES [Figs. 4(c), 4(f), and 4(i)]. Comparable nuclear contrast and distribution are noted between slide-free images provided by MUSES [Figs. 4(c), 4(f), 4(i)] and standard H&E [Figs. 4(d), 4(g), and 4(j)].
Figure 4.(a) 4X MUSE image of formalin-fixed mouse brain tissue stained with Rhodamine B and Hoechst 33342. (b)–(d) Zoomed-in 4X MUSE, 4X MUSES, and its standard H&E (from adjacent layer) images of the orange solid box marked in (a), respectively. (e)–(g) Zoomed-in images that correspond to the green dashed box regions marked in (b), (c), and (d), respectively. (h)–(j) Zoomed-in 4X MUSE, 4X MUSES, and its standard H&E (from adjacent layer) images of the yellow dotted box marked in (a), respectively.
D. High Tolerance to Tissue Irregularity and Visualization of Deeper Layers Using Fresh Hand-Cut Tissue Provided by MUSES
Figure 5 clearly shows the advantages of preserving long DOF in MUSES, which are more prominent when handling fresh tissues that are sectioned by a common blade. Surface irregularity has easily resulted without the use of specialized machines (e.g., a microtome). We demonstrated the advantage of using a low-NA objective lens (4×/0.1 NA) over a high-NA objective lens (10×/0.3 NA) in accommodating the surface irregularity of the hand-cut tissue. An obvious out-of-focus region is observed in the 10X MUSE image [Fig. 5(c)], while our 4X MUSE [Fig. 5(a)] and 4X MUSES [Fig. 5(b)] images can provide high tolerance to surface roughness, generating sufficient image contrast for better color transformation via deep learning. The corresponding color-transformed images [Figs. 5(d)–5(f)] illustrated the importance of sufficient image contrast for generating virtual H&E-stained images with the correct style transformation of the cell nuclei. Also, the improved resolution of the 4X MUSES image allows us to resolve subcellular features such as nucleoli [orange arrows, Fig. 5(h)], which are not visible in the corresponding 4X MUSE image [orange arrows, Fig. 5(g)]. Furthermore, cell nuclei at other depths are clearly visualized by preserving a longer DOF in our 4X MUSES image [orange arrows, Fig. 5(h)] when compared to the 10X MUSE image [orange arrows, Fig. 5(i)]. The drop of image resolution and contrast in the 4X and 10X MUSE images, respectively, also led to an incorrect color transformation of nuclei by the deep-learning algorithm [Figs. 5(j) and 5(l)], showing the importance of MUSES imaging.
Figure 5.(a)–(c) 4X MUSE, 4X MUSES, and 10X MUSE images of fresh hand-cut mouse brain tissue stained with Rhodamine B and Hoechst 33342. (d)–(f) Virtual H&E-stained images of (a), (b), and (c), respectively, generated by CycleGAN. (g)–(i) 4X MUSE, 4X MUSES, and 10X MUSE images of another fresh mouse brain tissue stained with Hoechst 33342 and propidium iodide. Cell nuclei from other layers are clearly visualized only in the 4X MUSES image with improved resolution and long DOF (orange arrows). (j)–(l) Virtual H&E-stained images of (g), (h), and (i), respectively, generated by CycleGAN.
4. CONCLUSION
In conclusion, building on the strengths of MUSE, this project achieved an average of 2.4 times resolution improvement on the reconstructed MUSES images, while preserving a long DOF and reducing the optical sectioning thickness by incorporating with an oblique speckle illumination using a low-NA objective lens. Depending on the needs of applications, resolution improvement could be further enhanced by generating a finer speckle pattern using a condenser lens with a higher NA. However, a few points should be considered: (1) an adequate working distance should be satisfied in this oblique illumination implementation to prevent light being blocked by the microscope body, (2) vignetting correction may be needed to compensate for uneven illumination across the field of view (FOV), and (3) modulation contrast may decrease when the pattern spatial frequency approaches the detection limit of the imaging system, and therefore, a condenser lens with an optimal NA should be chosen to provide sufficient speckle contrast, ensuring satisfactory reconstruction quality. In the current implementation, 144 speckle-illuminated images were captured in
By preserving a long DOF while enjoying high spatial resolution, we demonstrated the potential of MUSES in providing better image contrast when visualizing subcellular features by UV-surface excitation, as well as relieving the tissue flatness constraint. Although the use of a high-NA objective lens with extended DOF (EDOF) is also an option for addressing the surface roughness issue, one of the advantages of MUSES is that a large FOV can be simultaneously provided. The higher the objective NA used in the EDOF approach, the more images are required to cover the large FOV and long DOF; hence, a more extensive image processing would be needed. While MUSES resolution improvement is currently limited by the working distance of the condenser lens under this oblique illumination implementation, it could be a promising strategy in practice to first use MUSES for providing a large FOV with a long DOF while using a high-NA objective lens with EDOF to achieve higher resolution for a selected region of interest, further improving the efficiency in generating high-quality images. An unsupervised deep-learning algorithm, CycleGAN, was also implemented for generating virtual H&E-stained images based on MUSE or MUSES image inputs. These improvements help generalize UV-surface excitation to different organs and obviate the lengthy thin tissue slice preparation. The experimental results have shown the great potential of MUSES in providing reliable, high-resolution, and slide-free histological images during surgery.
References
[1] B. W. Maloney, D. McClatchy, B. Pogue, K. Paulsen, W. Wells, R. Barth. Review of methods for intraoperative margin detection for breast conserving surgery. J. Biomed. Opt., 23, 100901(2018).
[2] J. B. Taxy. Frozen section and the surgical pathologist a point of view. Arch. Pathol. Lab. Med., 133, 1135-1138(2009).
[3] F. T. Nguyen, A. M. Zysk, E. J. Chaney, J. G. Kotynek, U. J. Oliphant, F. J. Bellafiore, K. M. Rowland, P. A. Johnson, S. A. Boppart. Intraoperative evaluation of breast tumor margins with optical coherence tomography. Cancer Res., 69, 8790-8796(2009).
[4] D. S. Gareau, Y. G. Patel, Y. Li, I. Aranda, A. C. Halpern, K. S. Nehal, M. Rajadhyaksha. Confocal mosaicing microscopy in skin excisions: a demonstration of rapid surgical pathology. J. Microsc., 233, 149-159(2009).
[5] M. Ragazzi, S. Piana, C. Longo, F. Castagnetti, M. Foroni, G. Ferrari, G. Gardini, G. Pellacani. Fluorescence confocal microscopy for pathologists. Mod. Pathol., 27, 460-471(2014).
[6] T. Pham, B. Banerjee, B. Cromey, S. Mehravar, B. Skovan, H. Chen, K. Kieu. Feasibility of multimodal multiphoton microscopy to facilitate surgical margin assessment in pancreatic cancer. Appl. Opt., 59, G1-G7(2020).
[7] B. Wang, Q. Zhan, Y. Zhao, R. Wu, J. Liu, S. He. Visible-to-visible four-photon ultrahigh resolution microscopic imaging with 730-nm diode laser excited nanocrystals. Opt. Express, 24, A302-A311(2016).
[8] F. Fereidouni, Z. T. Harmany, M. Tian, A. Todd, J. A. Kintner, J. D. McPherson, A. D. Borowsky, J. Bishop, M. Lechpammer, S. G. Demos, R. Levenson. Microscopy with ultraviolet surface excitation for rapid slide-free histology. Nat. Biomed. Eng., 1, 957-966(2017).
[9] T. T. W. Wong, R. Zhang, P. Hai, C. Zhang, M. A. Pleitez, R. L. Aft, D. V. Novack, L. V. Wang. Fast label-free multilayered histology-like imaging of human breast cancer by photoacoustic microscopy. Sci. Adv., 3, e1602168(2017).
[10] D.-K. Yao. Optimal ultraviolet wavelength for
[11] T. Yoshitake, M. G. Giacomelli, L. M. Quintana, H. Vardeh, L. C. Cahill, B. E. Faulkner-Jones, J. L. Connolly, D. Do, J. G. Fujimoto. Rapid histopathological imaging of skin and breast cancer surgical specimens using immersion microscopy with ultraviolet surface excitation. Sci. Rep., 8, 4476(2018).
[12] C. Chiappa, F. Rovera, A. D. Corben, A. Fachinetti, V. De Berardinis, V. Marchionini, S. Rausei, L. Boni, G. Dionigi, R. Dionigi. Surgical margins in breast conservation. Int. J. Surg., 11, S69-S72(2013).
[13] T. Sellaro, R. Filkins, C. Hoffman, J. Fine, J. Ho, A. Parwani, L. Pantanowitz, M. Montalto. Relationship between magnification and resolution in digital pathology systems. J. Pathol. Inform., 4, 21(2013).
[14] M. G. L. Gustafsson. Surpassing the lateral resolution limit by a factor of two using structured illumination microscopy. J. Microsc., 198, 82-87(2000).
[15] E. Mudry, K. Belkebir, J. Girard, J. Savatier, E. Le Moal, C. Nicoletti, M. Allain, A. Sentenac. Structured illumination microscopy using unknown speckle patterns. Nat. Photonics, 6, 312-315(2012).
[16] F. Schmid, L. Beer. Biological macromolecules: UV-visible spectrophotometry. Encyclopedia of Life Science, 99, 178-181(2001).
[17] M. Guizar-Sicairos, S. T. Thurman, J. R. Fienup. Efficient subpixel image registration algorithms. Opt. Lett., 33, 156-158(2008).
[18] L.-H. Yeh, S. Chowdhury, L. Waller. Computational structured illumination for high-content fluorescence and phase microscopy. Biomed. Opt. Express, 10, 1978-1998(2019).
[19] Y. Zhang, L. Kang, I. H. M. Wong, W. Dai, X. Li, R. C. K. Chan, M. K. Y. Hsin, T. T. W. Wong. High-throughput, label-free and slide-free histological imaging by computational microscopy and unsupervised learning. Adv. Sci., 2102358(2021).
[20] A. Maiden, D. Johnson, P. Li. Further improvements to the ptychographical iterative engine. Optica, 4, 736-745(2017).
[21] M. Weigert, U. Schmidt, T. Boothe, A. Müller, A. Dibrov, A. Jain, B. Wilhelm, D. Schmidt, C. Broaddus, S. Culley, M. Rocha-Martins, F. Segovia-Miranda, C. Norden, R. Henriques, M. Zerial, M. Solimena, J. Rink, P. Tomancak, L. Royer, F. Jug, E. W. Myers. Content-aware image restoration: pushing the limits of fluorescence microscopy. Nat. Methods, 15, 1090-1097(2018).
[22] X. Li, G. Zhang, H. Qiao, F. Bao, Y. Deng, J. Wu, Y. He, J. Yun, X. Lin, H. Xie, H. Wang, Q. Dai. Unsupervised content-preserving transformation for optical microscopy. Light Sci. Appl., 10, 44(2021).
[23] Y. Zhang, K. de Haan, Y. Rivenson, J. Li, A. Delis, A. Ozcan. Digital synthesis of histological stains using micro-structured and multiplexed virtual staining of label-free tissue. Light Sci. Appl., 9, 78(2020).
[24] D. S. Gareau. Feasibility of digitally stained multimodal confocal mosaics to simulate histopathology. J. Biomed. Opt., 14, 034050(2009).
[25] M. G. Giacomelli, L. Husvogt, H. Vardeh, B. E. Faulkner-Jones, J. Hornegger, J. L. Connolly, J. G. Fujimoto. Virtual hematoxylin and eosin transillumination microscopy using epi-fluorescence imaging. PLoS ONE, 11, e0159337(2016).
[26] G. Barbastathis, A. Ozcan, G. Situ. On the use of deep learning for computational imaging. Optica, 6, 182-192(2021).
[27] J. Y. Zhu, T. Park, P. Isola, A. A. Efros. Unpaired image-to-image translation using cycle-consistent adversarial networks. IEEE International Conference on Computer Vision (ICCV), 2242-2251(2017).
[28] Z. Chen, W. Yu, I. H. M. Wong, T. T. W. Wong. Deep-learning-assisted microscopy with ultraviolet surface excitation for rapid slide-free histological imaging. Biomed. Opt. Express, 12, 5920-5938(2021).
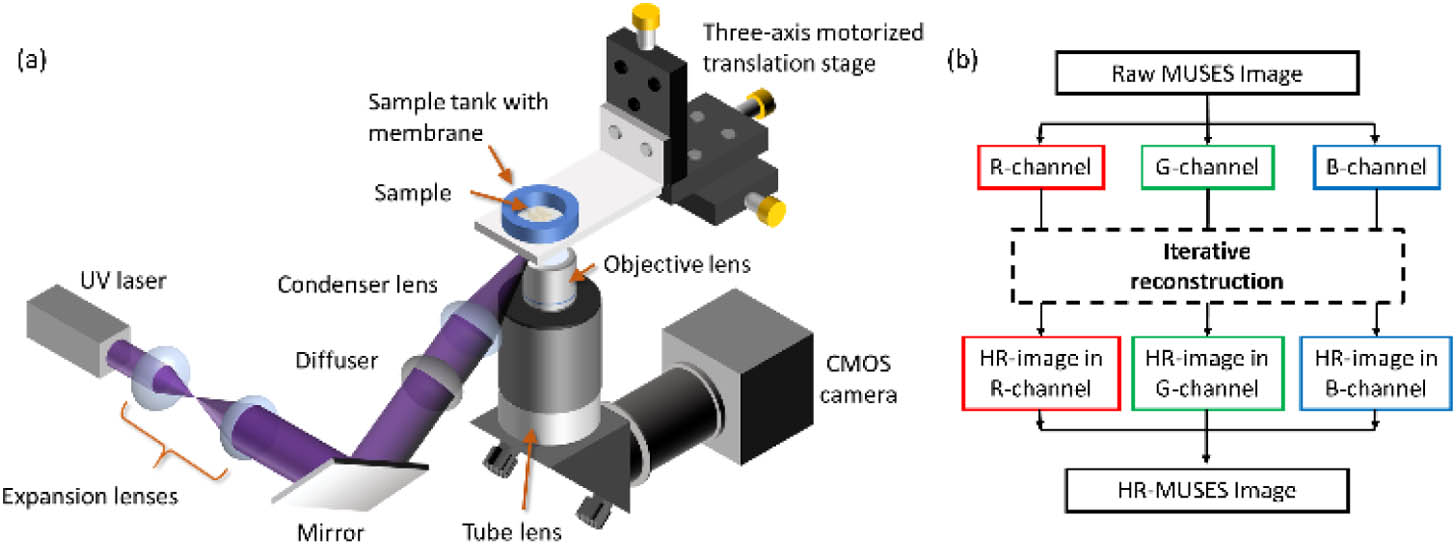
Set citation alerts for the article
Please enter your email address