Guowu Zhang, Dan-Xia Xu, Yuri Grinberg, Odile Liboiron-Ladouceur, "Experimental demonstration of robust nanophotonic devices optimized by topological inverse design with energy constraint," Photonics Res. 10, 1787 (2022)

Search by keywords or author
- Photonics Research
- Vol. 10, Issue 7, 1787 (2022)
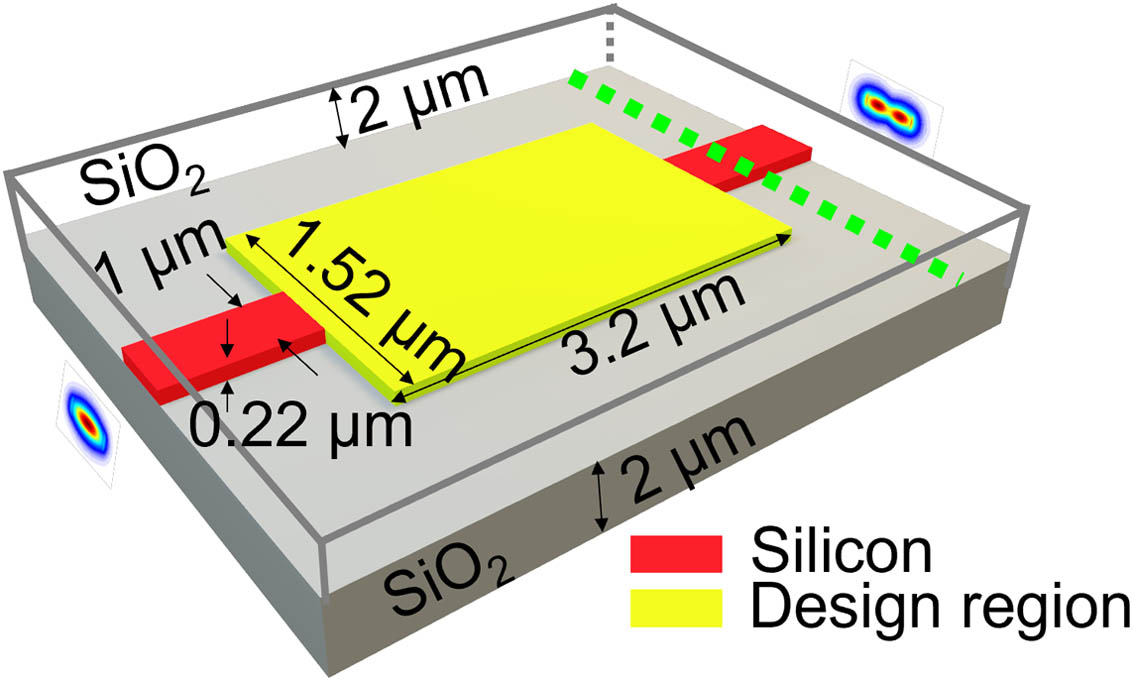
Fig. 1. Illustration of the dimensions of the input and output waveguides (in red) and the design region (in yellow) for the TE0 to TE1 mode converter.
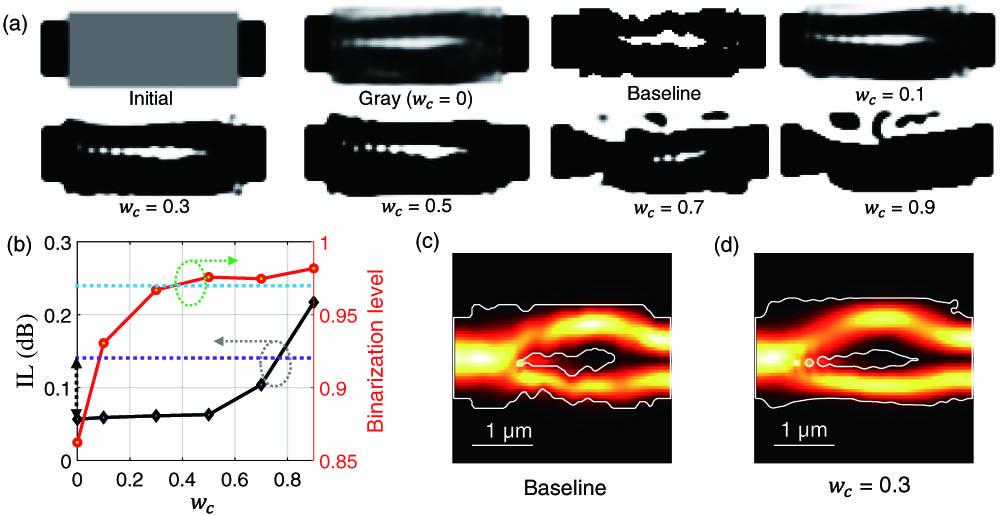
Fig. 2. (a) Initial and optimized mode converters for gray scale optimization strategies (note that w c = 0 corresponds to a gray scale optimization), baseline, and with energy constraint coefficients w c of 0.1, 0.3, 0.5, 0.7, and 0.9; (b) insertion loss and binarization level as a function of the energy constraint coefficient parameter w c (horizontal purple dashed line, IL performance of the baseline design; horizontal light blue dashed line, binarization level of the baseline design); energy intensity distribution for the optimized designs (c) without and (d) with energy constraint.
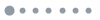
Fig. 3. Optical image of the test structures for characterizing the mode converter in terms of (a) IL performance and (b) cross talk performance; (c) SEM images for the fabricated design of the baseline (left) and energy constraint strategies (right); (d) measured IL and XT for the mode converter designed by the baseline (solid line) and energy constraint strategies (dashed line); right part shows the zoom in version of the measured IL performance.
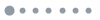
Fig. 4. (a) Illustration of the dimensions of the input and output waveguides (in red) and the design region (in yellow) for the 1310 nm/1550 nm wavelength duplexer; (b) illustration of the wavelength points at which the optimization is conducted for the 1310 nm and 1550 nm channels, respectively.
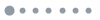
Fig. 5. (a) Initial and optimized 1310 nm/1550 nm wavelength duplexer layouts for different strategies (note that w c = 0 corresponds to the gray scale optimization); (b) energy intensity distribution inside the optimized designs for baseline (left) and the case with energy constraint coefficient w c = 0.3 (right) (red, 1550 nm; cyan, 1310 nm); simulated transmission spectrum results from (c) baseline optimization and (d) the case with energy constraint coefficient w c = 0.3 .
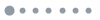
Fig. 6. Simulated transmission spectrum for (a) baseline optimization and (b) the case with energy constraint coefficient w c = 0.3 under ± 10 nm fabrication error. For clarity of the figure, the data for the nominal designs are not shown here.
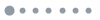
Fig. 7. Histogram of minimum feature size ranges for (a) 15 baseline optimization runs and (b) 15 runs with an energy constraint coefficient of w c = 0.3 ; overlap of all 15 simulated transmission spectra for (c) baseline optimization and (d) with energy constraint coefficient w c = 0.3 under ± 10 nm fabrication imperfection.
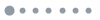
Fig. 8. SEM images of eight fabricated 1310 nm/1550 nm wavelength duplexers optimized from different random start parameters. Top row, devices from baseline optimization strategy; bottom row, corresponding devices from energy constraint optimization strategy.
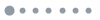
Fig. 9. Measured and normalized transmission spectrum of the fabricated 1310 nm/1550 nm wavelength duplexer under ± 10 nm and 0 nm boundary bias for (a) baseline, (b) energy constraint optimization; filtered transmission spectrum of the results presented in (a) and (b) for (c) baseline, (d) energy constraint optimization.
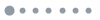
Fig. 10. Measured, normalized, and smoothed transmission spectra of the fabricated 1310 nm/1550 nm wavelength duplexers under different ± 10 nm boundary bias for the 15 designs optimized from different random initial parameters for (a) baseline and (b) energy constraint optimization.
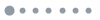
Fig. 11. (a) Illustration of the dimensions of the input and output waveguides (in red) and the design region (in yellow) for the CWDM demultiplexer. (b) Illustration of the wavelength points at which the optimization is conducted for the CH1, CH2, and CH3, respectively.
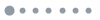
Fig. 12. (a) Optimized CWDM demultiplexer layouts for different strategies (note that w c = 0 corresponds to the gray scale only optimization); (b) corresponding energy distribution inside the optimized designs with different energy constraint coefficients; (c) corresponding simulated transmission spectrum for the optimized designs with different parameters w c .
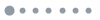
Fig. 13. Simulated transmission spectrum of the optimized CWDM demultiplexers under different ± 10 nm and 0 nm boundary biases optimized with different energy constraint coefficients for CH1 (left), CH2 (middle) and CH3 (right), (a) w c = 0.5 , (b) w c = 0.7 , and (c) w c = 0.9 , respectively.
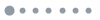
Fig. 14. (a) SEM images of the fabricated CWDM demultiplexer for w c = 0.5 , 0.7 , 0.9 ; (b) zoom-in SEM images with the overlap of the desired boundary; (c)–(e) measured transmission spectrum of the nominal demultiplexers optimized with energy constraint coefficients w c = 0.5 , 0.7 , 0.9 .
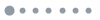
Fig. 15. Measured transmission spectrum of the fabricated CWDM demultiplexers under different ± 10 nm and 0 nm boundary biases optimized with different energy constraint coefficients for CH1 (left), CH2 (middle), and CH3 (right), respectively, (a) w c = 0.5 , (b) w c = 0.7 , and (c) w c = 0.9 .
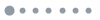
Fig. 16. Measured spectrum shifts due to ± 10 nm etching changes as a function of the energy constraint coefficient w c for CH1 (blue), CH2 (green), and CH3 (red).
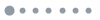
Fig. 17. Top view illustration of filtering and projection on a predefined design region (dash blue line): (a) design variable of material distribution ρ ranging between 0 and 1, (b) the variable of material distribution ρ ˜ after filtering using a hat-shaped filter h ( x , y ) shown in the insert, (c) the variable of material distribution ρ ¯ , which is the projection of the filtered material distribution ρ ˜ . The inset for projection shows how changing β will help binarize the structure.
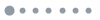
Fig. 18. (a) Illustration of continuous distribution from optimization; (b) illustration of the GDS transferring process using Eq. (A15 ); (c) illustration of boundary bias erosion (− 10 nm ), normal (0 nm), and dilation (+ 10 nm ) at the cross section position; (d) and (e) zoom-in illustration for part of the design boundary.
|
Table 1. Comparison of the Performance of Mode Converters with the Previous Reported Inversely Designed Mode Converters/Exchangers
|
Table 2. Comparison of the Performance of the 1310 nm/1550 nm Wavelength Duplexer with Previous Reported Inversely Designed 1310 nm/1550 nm Wavelength Duplexers
|
Table 3. Simulated and Measured Spectrum Shifts for CH1, CH2, and CH3 under Different Energy Constraint Coefficients for ± 10 nm Boundary Biases
|
Table 4. Comparison of the Performance of the CWDM Demultiplexers with Previous Reported Inversely Designed CWDM Demultiplexers
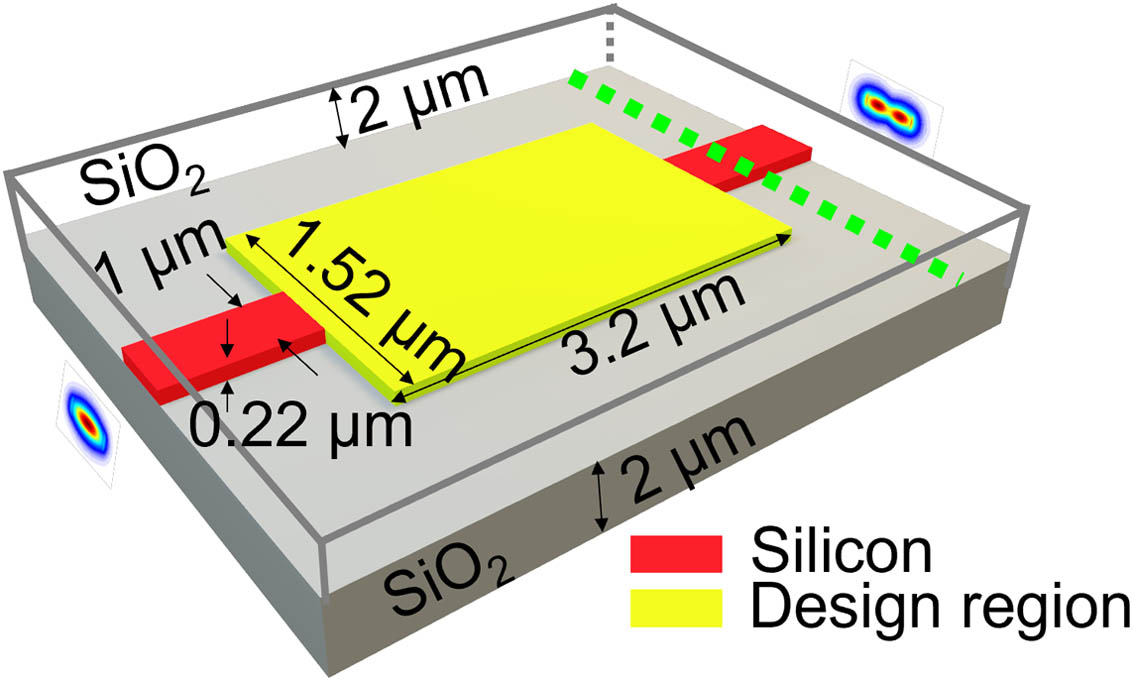
Set citation alerts for the article
Please enter your email address