Zhengfen Jiang, Boyi Li, Tho N. H. T. Tran, Jiehui Jiang, Xin Liu, Dean Ta, "Fluo-Fluo translation based on deep learning," Chin. Opt. Lett. 20, 031701 (2022)

Search by keywords or author
- Chinese Optics Letters
- Vol. 20, Issue 3, 031701 (2022)
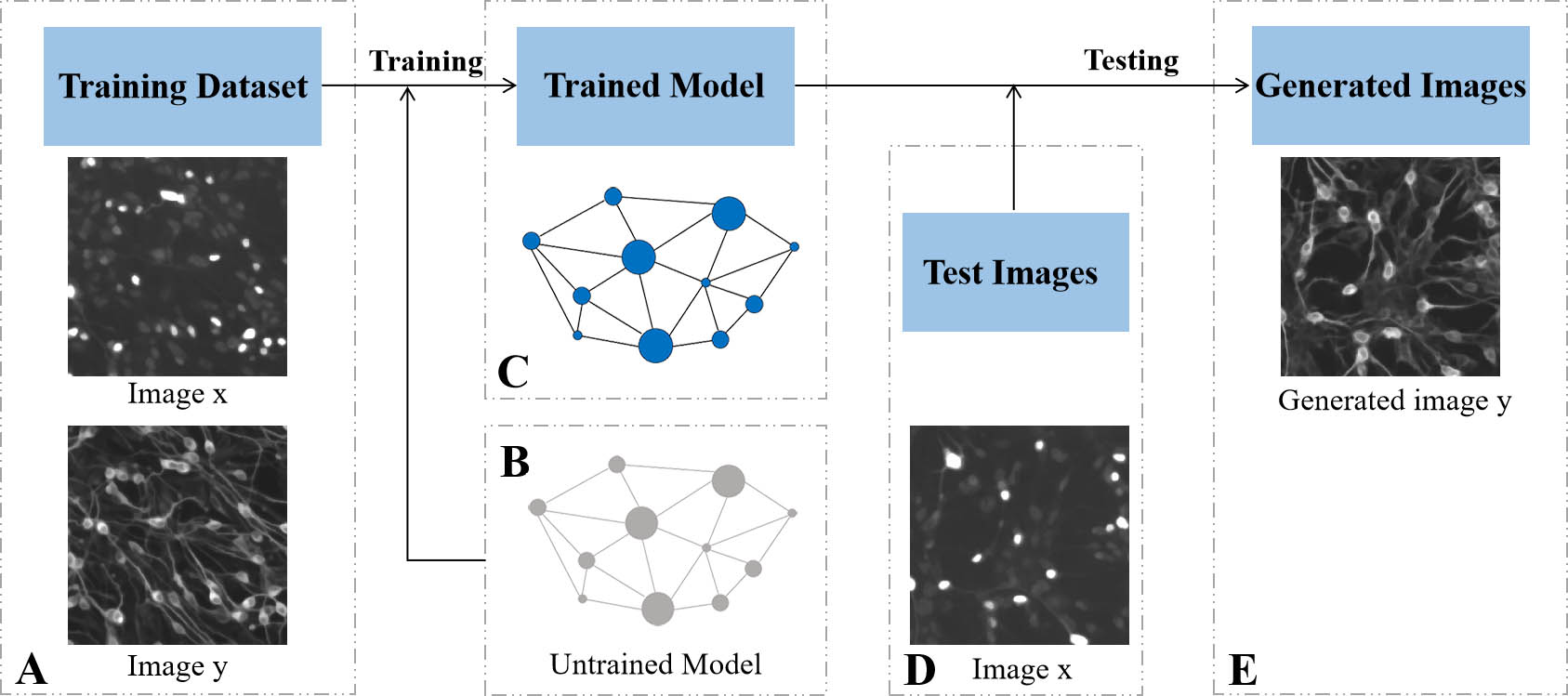
Fig. 1. System for Fluo-Fluo translation based on cGAN. (A) The training dataset is composed of fluorescence images x and y in the same field of view. (B) A deep neural network is composed of untrained parameters. (C) The deep neural network trained with data in (A). (D) Test image. (E) Based on the trained deep learning model, the fluorescence image y is predicted from the fluorescence image x.
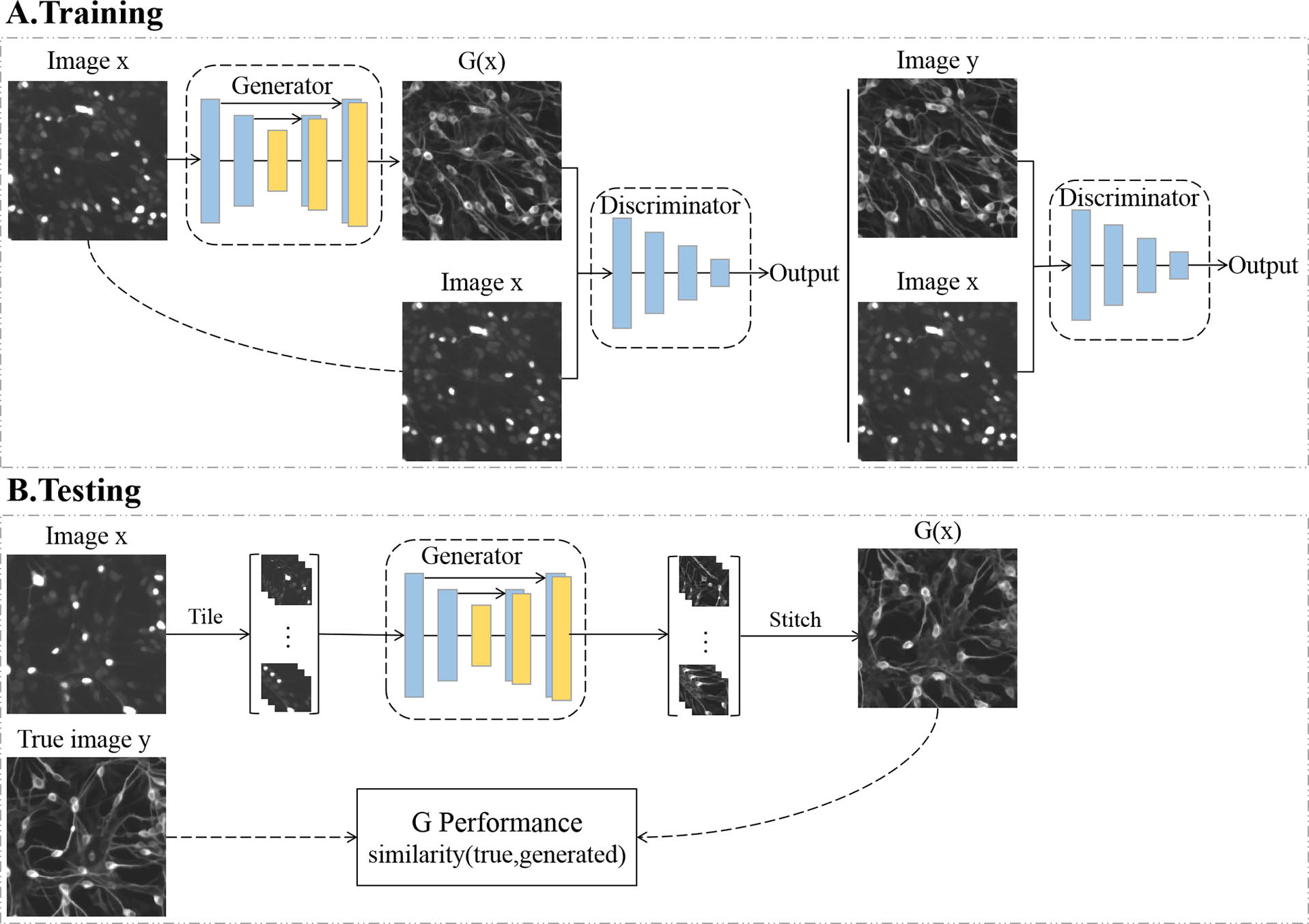
Fig. 2. cGAN framework for Fluo-Fluo translation based on deep learning. (A) The generator network attempts to generate image y with respect to image x, and the discriminator network attempts to distinguish between the generated image y and the true image y. There is a competitive relationship between these two networks. Briefly, image x is used as the input of the generator to obtain the generated image G(x), and then G(x) and x are combined as the input of the discriminator. During training, two error functions are calculated: (i) L1 and MS-SSIM loss functions are used to measure the similarity between the generated image G(x) and the target image y; (ii) the cGAN error attempts to distinguish the generated image G(x) from the target image y corresponding to the input image x. The combined loss functions are optimized by the Adam algorithm. (B) Once trained, the generator can immediately predict the fluorescence image y from the fluorescence image x of the test dataset.
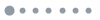
Fig. 3. Prediction results obtained by the proposed method. (A) Predict TuJ1 protein from Islet1 protein based on deep learning. (B) Predict CellMask from DAPI based on deep learning. (C) Predict PI from Hoechst based on deep learning. From left to right, the input image, the true image (ground truth), the network generated image, the absolute error map, and the scatter plot are displayed in turn.
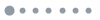
Fig. 4. Prediction results obtained by the proposed method. (A) Predict MAP2 from DAPI based on deep learning. (B) Predict NFH from DAPI based on deep learning. (C) Multi-label visualization co-localization.
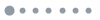
Fig. 5. Effects of the number of training sets on the prediction performance of the proposed method. (A) and (B) Prediction results obtained by using the reduced training sets. (A) Predict TuJ1 from Islet1. (B) Predict PI from Hoechst. (C) and (D) Quantify the differences in terms of the SSIM, PSNR, and MAE indicators, respectively.
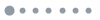
Fig. 6. Predict CellMask from DAPI based on our rat cardiomyocyte dataset. The public dataset from Group 2 (predicting CellMask from DAPI based on human breast cancer cells) is used for network training, and then the trained model is used to predict our own experimental data.
|
Table 1. Detailed Information of Experimental Data
|
Table 2. SSIM, PSNR, and MAE Values Between the True and the Network Generated Images
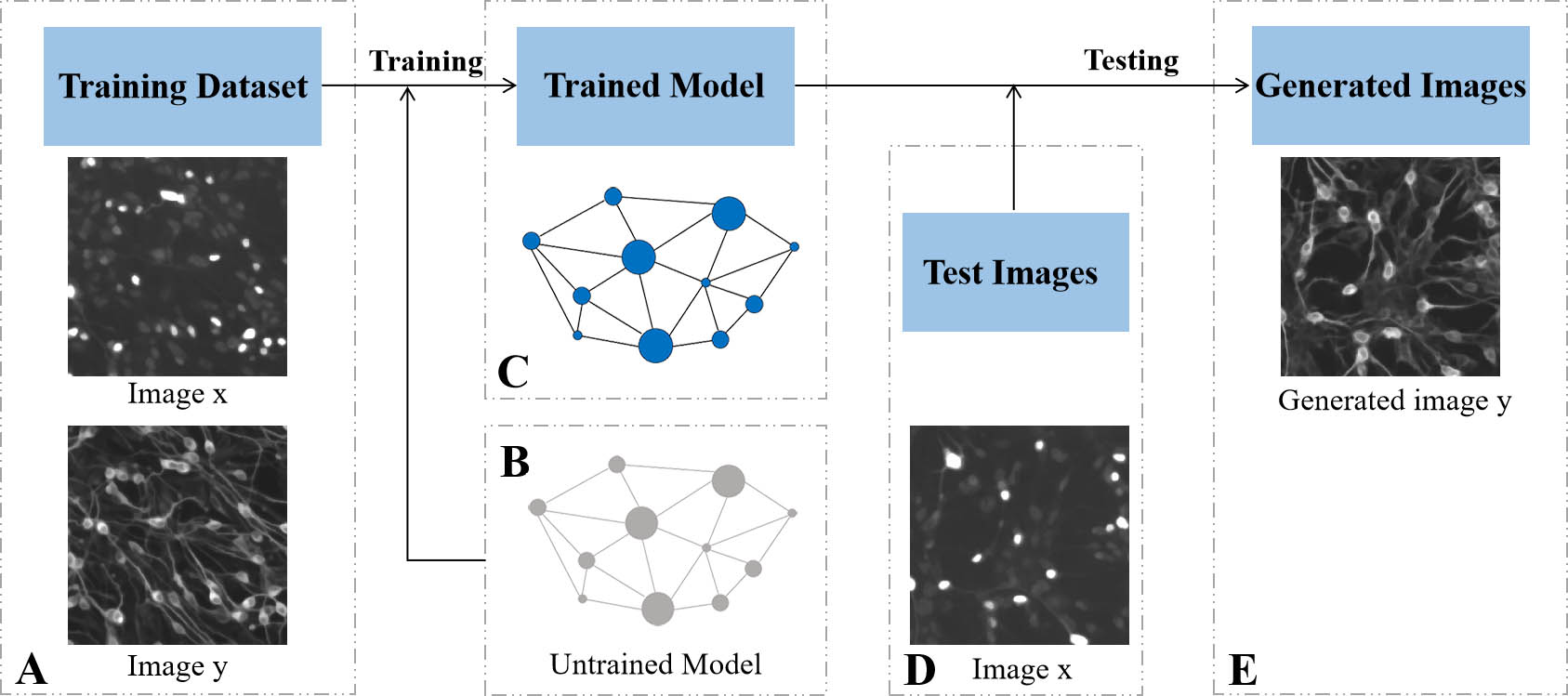
Set citation alerts for the article
Please enter your email address