Zhengfen Jiang, Boyi Li, Tho N. H. T. Tran, Jiehui Jiang, Xin Liu, Dean Ta, "Fluo-Fluo translation based on deep learning," Chin. Opt. Lett. 20, 031701 (2022)

Search by keywords or author
- Chinese Optics Letters
- Vol. 20, Issue 3, 031701 (2022)
Abstract
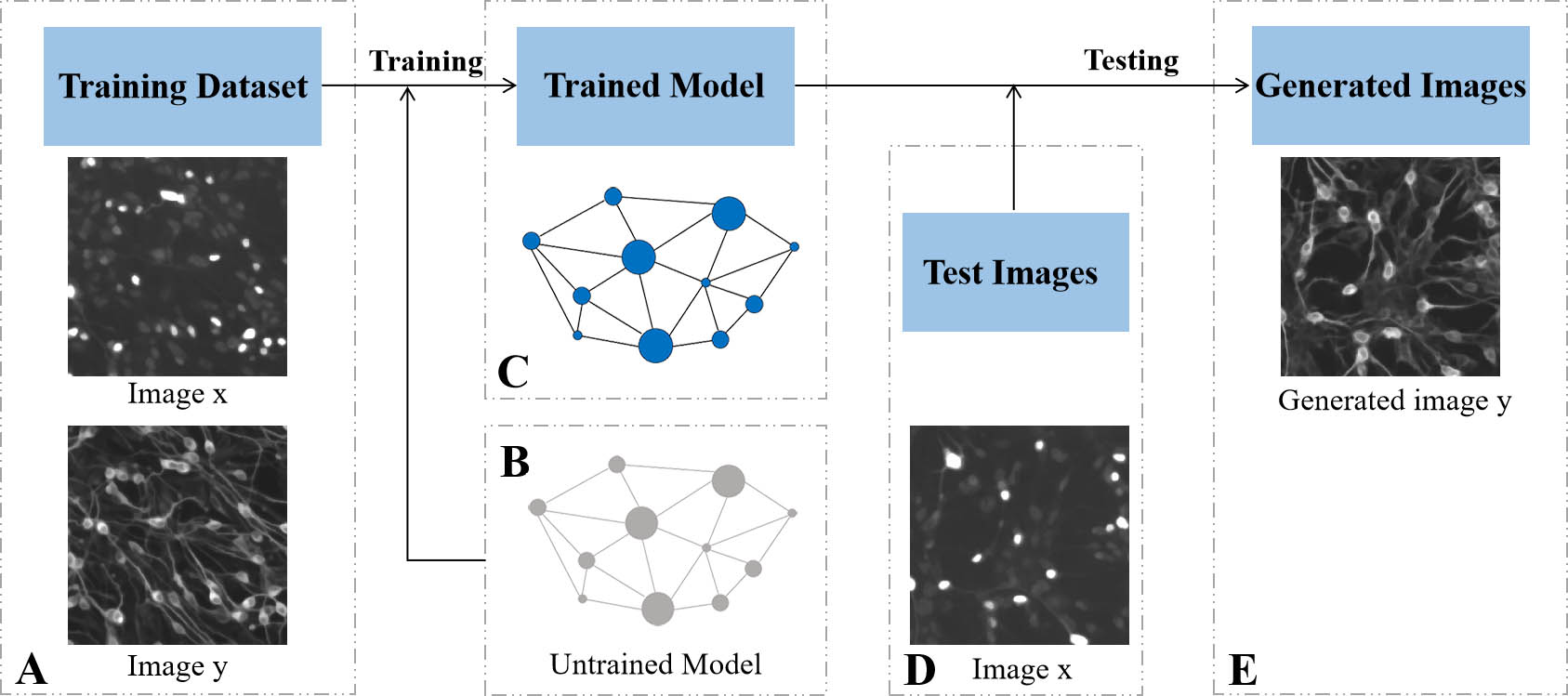
Set citation alerts for the article
Please enter your email address