Aoni Wei, Chengbing Qin, Shuai Dong, Xinqin Meng, Yunrui Song, Xiangdong Li, Xilong Liang, Guofeng Zhang, Ruiyun Chen, Jianyong Hu, Zhichun Yang, Jianzhong Huo, Liantuan Xiao, Suotang Jia. Research Progress of Super-Resolution Fluorescence Microscopy[J]. Laser & Optoelectronics Progress, 2023, 60(11): 1106012

Search by keywords or author
- Laser & Optoelectronics Progress
- Vol. 60, Issue 11, 1106012 (2023)
![Development of super-resolution photoluminescence imaging techniques[10]](/richHtml/lop/2023/60/11/1106012/img_01.jpg)
Fig. 1. Development of super-resolution photoluminescence imaging techniques[10]
![Schematic diagrams of the principle and experimental setup of MINFLUX. (a) (b) Schematic diagrams of the principle to localize the center of fluorescent molecules for common nanoscopy and MINFLUX, respectively[17]; (c) schematic diagram of the setup of MINFLUX[18]; (d)-(f) principles of MINFLUX illustrated in one, two, and three dimensions, respectively[16,18]; (g) comparison of the spatial resolution between SMLM and MINFLUX under same photon counts (scale is 10 nm)[16]](/richHtml/lop/2023/60/11/1106012/img_02.jpg)
Fig. 2. Schematic diagrams of the principle and experimental setup of MINFLUX. (a) (b) Schematic diagrams of the principle to localize the center of fluorescent molecules for common nanoscopy and MINFLUX, respectively[17]; (c) schematic diagram of the setup of MINFLUX[18]; (d)-(f) principles of MINFLUX illustrated in one, two, and three dimensions, respectively[16,18]; (g) comparison of the spatial resolution between SMLM and MINFLUX under same photon counts (scale is 10 nm)[16]
![Development in terms of resolution and 3D multicolor imaging of MINFLUX. (a) Two-color MINFLUX nanoscopy in 3D of U-2 OS cell with AF647 and CF680(scale: 500 nm)[19]; (b) three-dimensional localization accuracy of MINFLUX[20]; (c) schematic of the p-MINFLUX setup[21]; (d)(e) 2D localization image and fluorescence lifetime image for one DNA origami structure through p-MINFLUX[21]; (f)(g) measurement method based on grating scanning single molecule localization and imaging simulation results of this method at different scanning distances[23]](/Images/icon/loading.gif)
Fig. 3. Development in terms of resolution and 3D multicolor imaging of MINFLUX. (a) Two-color MINFLUX nanoscopy in 3D of U-2 OS cell with AF647 and CF680(scale: 500 nm)[19]; (b) three-dimensional localization accuracy of MINFLUX[20]; (c) schematic of the p-MINFLUX setup[21]; (d)(e) 2D localization image and fluorescence lifetime image for one DNA origami structure through p-MINFLUX[21]; (f)(g) measurement method based on grating scanning single molecule localization and imaging simulation results of this method at different scanning distances[23]
![Super-resolution imaging by combining the MINFLUX with other techniques. Simulation of z-axis localization for (a) single-photon MINFLUX and (b) two-photon MINFLUX, respectively(scale: 1 nm)[25];(c) concept of ISM-FLUX, an activation laser beam activates a single fluorophore in the sample (yellow star) which is sequentially excited by a series of spatially displaced doughnut beams[27]; (d) SMLM and (e) SIMFLUX image for the same nano-rulers(scale: 50 nm)[28]; (f) comparison of imaging effects of confocal microscopy, (g) stimulated radiation loss microscopy, and (h) minimum photon number stimulated radiation loss technique on similar mitochondria (scale: 200 nm)[29]; (i) comparison of confocal fluorescence imaging using Vimentin-reEGFP2 fluorescent protein (left image) and minimum photon number microscopy imaging using DNA-PAINT technology (scale: 200 nm)[31]](/Images/icon/loading.gif)
Fig. 4. Super-resolution imaging by combining the MINFLUX with other techniques. Simulation of z-axis localization for (a) single-photon MINFLUX and (b) two-photon MINFLUX, respectively(scale: 1 nm)[25];(c) concept of ISM-FLUX, an activation laser beam activates a single fluorophore in the sample (yellow star) which is sequentially excited by a series of spatially displaced doughnut beams[27]; (d) SMLM and (e) SIMFLUX image for the same nano-rulers(scale: 50 nm)[28]; (f) comparison of imaging effects of confocal microscopy, (g) stimulated radiation loss microscopy, and (h) minimum photon number stimulated radiation loss technique on similar mitochondria (scale: 200 nm)[29]; (i) comparison of confocal fluorescence imaging using Vimentin-reEGFP2 fluorescent protein (left image) and minimum photon number microscopy imaging using DNA-PAINT technology (scale: 200 nm)[31]
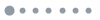
Fig. 5. Comparison of wide field imaging, minimum photon number microscopic imaging technology, and their combination with other super-resolution methods in field of view, photon efficiency, signal to back ratio, system simplicity, and resolution ability
![Principle of SOFI[32]. (a) Emitter distribution in the object plane; (b) magnified detail of the dotted box in Fig. (a); (c) time trajectory of each pixel; (d) second-order correlation function is calculated from the fluctuations for each pixel; (e) result of integrating the second-order correlation function per pixel](/Images/icon/loading.gif)
Fig. 6. Principle of SOFI[32]. (a) Emitter distribution in the object plane; (b) magnified detail of the dotted box in Fig. (a); (c) time trajectory of each pixel; (d) second-order correlation function is calculated from the fluctuations for each pixel; (e) result of integrating the second-order correlation function per pixel
![SOFI images with different fluorescent labels. (a) Schematic diagram of joint labeled wave super-resolution microscopy imaging[37]; (b) schematic cross-section of the joint-tagging SOFI imaging[37]; (c) workflow of multicolor SOFI imaging by spectral cross-cumulant analysis followed by linear unmixing using simulations[38]; (d) conventional and (e) pcSOFI image of HeLa cell labeled with Lyn-Dronpa (green) and Kras-rsTagRFP (red), respectively[41] (scale: 10 μm)](/Images/icon/loading.gif)
Fig. 7. SOFI images with different fluorescent labels. (a) Schematic diagram of joint labeled wave super-resolution microscopy imaging[37]; (b) schematic cross-section of the joint-tagging SOFI imaging[37]; (c) workflow of multicolor SOFI imaging by spectral cross-cumulant analysis followed by linear unmixing using simulations[38]; (d) conventional and (e) pcSOFI image of HeLa cell labeled with Lyn-Dronpa (green) and Kras-rsTagRFP (red), respectively[41] (scale: 10 μm)
![Flowchart of algorithm to solve artifacts in the high-order SOFI. (a) Flowchart of local dynamic range compression algorithm[44]; (b) flowchart to illustrate the different steps of the bSOFI algorithm[45]](/Images/icon/loading.gif)
Fig. 8. Flowchart of algorithm to solve artifacts in the high-order SOFI. (a) Flowchart of local dynamic range compression algorithm[44]; (b) flowchart to illustrate the different steps of the bSOFI algorithm[45]
![Flowchart of the analysis of SOFI data and the contrast between SOFI and other imaging techniques. (a) Schematic overview of a super-resolution localization or SOFI analysis[46]; (b) comparison of the spatial distribution of individual chromophores and their wide field imaging, balanced super-resolution wave microscopy imaging, and random optical reconstruction super-resolution imaging[48]; (c) comparison of confocal fluorescence microscopy, image scanning microscopy, and second-order and fourth-order super-resolution optical wave image scanning microscopy for commercial quantum dot QD625[51]](/Images/icon/loading.gif)
Fig. 9. Flowchart of the analysis of SOFI data and the contrast between SOFI and other imaging techniques. (a) Schematic overview of a super-resolution localization or SOFI analysis[46]; (b) comparison of the spatial distribution of individual chromophores and their wide field imaging, balanced super-resolution wave microscopy imaging, and random optical reconstruction super-resolution imaging[48]; (c) comparison of confocal fluorescence microscopy, image scanning microscopy, and second-order and fourth-order super-resolution optical wave image scanning microscopy for commercial quantum dot QD625[51]
![Principle of super-resolution image based on anti-bunching effect. (a) Schematic diagram of the HBT measurement[61]; (b) anti-bunching signal of single molecule excited by continuous laser[62]; (c)-(e) first order, second order, and third order anti-bunching images of quantum dots in the same region[64]](/Images/icon/loading.gif)
Fig. 10. Principle of super-resolution image based on anti-bunching effect. (a) Schematic diagram of the HBT measurement[61]; (b) anti-bunching signal of single molecule excited by continuous laser[62]; (c)-(e) first order, second order, and third order anti-bunching images of quantum dots in the same region[64]
![Imaging results of super-resolution image based on anti-bunching effect. (a) Schematic diagram of a super-resolution imaging device for measuring second-order anti-bunching in a confocal system[65]; (b) reconstructed image according to the single and double photon signals of NVs collected from the system in a[65]; (c) schematic diagram of a super-resolution imaging device for measuring high-order anti-bunching with a single-photon fiber beam camera[67]; (d) two-dimensional localization accuracy measured for a single QD (solid blue) and theoretical accuracy by using the system in c[67]; (e) schematic of SIQCM by combining SIM and quantum correlation microscopy[68]; (f) simulation results illustrated the resolution of SIQCM[68]; (g) (h) imaging results of QD625-labeled microtubule cell samples in confocal microscopy and Q-ISM[69]](/Images/icon/loading.gif)
Fig. 11. Imaging results of super-resolution image based on anti-bunching effect. (a) Schematic diagram of a super-resolution imaging device for measuring second-order anti-bunching in a confocal system[65]; (b) reconstructed image according to the single and double photon signals of NVs collected from the system in a[65]; (c) schematic diagram of a super-resolution imaging device for measuring high-order anti-bunching with a single-photon fiber beam camera[67]; (d) two-dimensional localization accuracy measured for a single QD (solid blue) and theoretical accuracy by using the system in c[67]; (e) schematic of SIQCM by combining SIM and quantum correlation microscopy[68]; (f) simulation results illustrated the resolution of SIQCM[68]; (g) (h) imaging results of QD625-labeled microtubule cell samples in confocal microscopy and Q-ISM[69]
![Development of neural network architecture. (a) Taxonomy of AI[72]; (b) overall architecture of the CNN includes an input layer, multiple alternating convolution, and max-pooling layers, one fully-connected layer, and one classification layer[72]; (c) GAN model frame diagram[75]; (d) U-net architecture[76]](/Images/icon/loading.gif)
Fig. 12. Development of neural network architecture. (a) Taxonomy of AI[72]; (b) overall architecture of the CNN includes an input layer, multiple alternating convolution, and max-pooling layers, one fully-connected layer, and one classification layer[72]; (c) GAN model frame diagram[75]; (d) U-net architecture[76]
![Latest progress in fluorescence super-resolution microscopy imaging based on deep learning. (a) Reconstruction results of wide-field images of bovine pulmonary artery endothelial cells through deep learning[78]; (b) total internal reflection fluorescence microscopic effect based on depth learning, and its comparison with structured light super-resolution microscopic imaging based on total internal reflection, the cell used is SUM159[78]; (c) resolution characterization of GAN net [79]; (d) based on unsupervised content retention transformation, microscopic imaging can convert wide-field images into super-resolution images, enabling the resolution of sub diffractive structures such as microtubules and secretory particles from the wide-field images[80]; (e) statistical comparison of normalized root mean square error, multi-scale structure similarity index, and resolution of 121 groups of actin images reconstructed by scU-net, DFCAN, and DFGAN networks respectively, black cross is the outlier[82]](/Images/icon/loading.gif)
Fig. 13. Latest progress in fluorescence super-resolution microscopy imaging based on deep learning. (a) Reconstruction results of wide-field images of bovine pulmonary artery endothelial cells through deep learning[78]; (b) total internal reflection fluorescence microscopic effect based on depth learning, and its comparison with structured light super-resolution microscopic imaging based on total internal reflection, the cell used is SUM159[78]; (c) resolution characterization of GAN net [79]; (d) based on unsupervised content retention transformation, microscopic imaging can convert wide-field images into super-resolution images, enabling the resolution of sub diffractive structures such as microtubules and secretory particles from the wide-field images[80]; (e) statistical comparison of normalized root mean square error, multi-scale structure similarity index, and resolution of 121 groups of actin images reconstructed by scU-net, DFCAN, and DFGAN networks respectively, black cross is the outlier[82]
![Deep learning-enabled transformation of images of curves from 1d_SIM to 9_SIM[83]. (a) WF curve image; (b) 1d_SIM image is network input; (c) 3_SIM image is network output; (d) 9_SIM image is real images. Reconstruction effect of microtubule imaging based on U-Net network[81]. (e) Average projection of 15 SIM raw data images; (f) reconstruction results of reconstruction algorithm of traditional structured light illumination microscopic imaging; (g) output effect of U-Net-SIM15 network; (h) output effect of U-Net-SIM3 network. Comparison of results of ANNA-PALM reconstruction of immunostaining microtubule microscopy images[85]. (i) Widefield image; (j) sparse PALM image obtained from the first 9 s of acquisition (k=300 frame, n=11740 localizations); (k) dense PALM image obtained from a 15 min-long acquisition (K=30000 frame, N=409364 localizations); (l) ANNA-PALM reconstruction from the widefield Fig. (i) only; (m) ANNA-PALM reconstruction from the sparse PALM Fig. (j) only; (n) ANNA-PALM reconstruction from the widefield Fig. (i) and sparse PALM Fig. (j) combined (scale: 1 µm). Two-color sSMLM images in COS-7 cell[86]. (o) Low-density image with 3000 frame; (p) deep CNN reconstruction; (q) high-density image with 19997 frame (pixel size:16 nm. scale: 1.5 µm)](/Images/icon/loading.gif)
Fig. 14. Deep learning-enabled transformation of images of curves from 1d_SIM to 9_SIM[83]. (a) WF curve image; (b) 1d_SIM image is network input; (c) 3_SIM image is network output; (d) 9_SIM image is real images. Reconstruction effect of microtubule imaging based on U-Net network[81]. (e) Average projection of 15 SIM raw data images; (f) reconstruction results of reconstruction algorithm of traditional structured light illumination microscopic imaging; (g) output effect of U-Net-SIM15 network; (h) output effect of U-Net-SIM3 network. Comparison of results of ANNA-PALM reconstruction of immunostaining microtubule microscopy images[85]. (i) Widefield image; (j) sparse PALM image obtained from the first 9 s of acquisition (k=300 frame, n=11740 localizations); (k) dense PALM image obtained from a 15 min-long acquisition (K=30000 frame, N=409364 localizations); (l) ANNA-PALM reconstruction from the widefield Fig. (i) only; (m) ANNA-PALM reconstruction from the sparse PALM Fig. (j) only; (n) ANNA-PALM reconstruction from the widefield Fig. (i) and sparse PALM Fig. (j) combined (scale: 1 µm). Two-color sSMLM images in COS-7 cell[86]. (o) Low-density image with 3000 frame; (p) deep CNN reconstruction; (q) high-density image with 19997 frame (pixel size:16 nm. scale: 1.5 µm)
|
Table 1. Parameters of the deep-learning super-resolution imaging
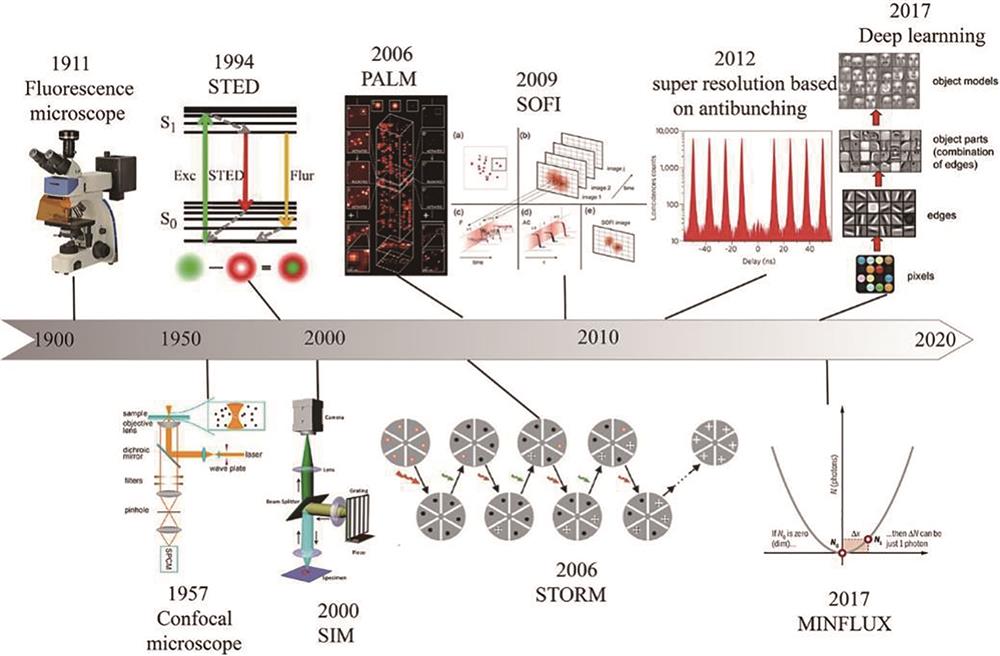
Set citation alerts for the article
Please enter your email address