
- Photonics Research
- Vol. 10, Issue 2, 433 (2022)
Abstract
1. INTRODUCTION
As one of the largest infrastructures, the optical fiber network has shown potential in more and more applications. Beside the basic data transmission function, it can be utilized as medium for time-frequency dissemination in the metrological field [1–12] and can also serve as the sensing medium to detect vibration events in the geodesy and earth observation fields [13–19]. Considering the widely distributed urban fiber links, it is likely to monitor public infrastructures in urban area, such as traffic vibrations, which will enrich the sensing method of a smart city.
In our previous study, we constructed a fiber-based time-frequency network in Beijing [Fig. 1(a)]. Through actively compensating the phase fluctuation, several urban fiber links were employed to realize a real-time frequency comparison network, which included five hydrogen masers from four institutions [20,21]. On the other hand, this fiber network has the potential to serve as a huge interferometer to detect vibrations along it. Generally, fiber vibration sensing techniques can be sorted as forward-transmission [22–26] and backscattering [27–33] schemes. The backscattering scheme, such as the distributed acoustic sensing, is a mature technique. However, great optical loss limits its usage range. Furthermore, due to the backscattering detection and the high-power injection pulses, it normally needs to occupy a dark fiber alone. In our case, we choose the forward-transmission scheme, which can be integrated with fiber communication function [15]. However, the main problem of this scheme is how to precisely localize vibration events. This problem limits its application area compared with the backscattering scheme.
Figure 1.Layout of the urban fiber test platform and scenarios of detected vibration events. (a) The fiber-based time-frequency network in Beijing mainly connecting Tsinghua University (THU) with the National Institute of Metrology (NIM), Beijing Satellite Navigation Center (BSNC), and Beijing Institute of Radio Metrology and Measurement (BIRM), respectively. To make a preliminary study of traffic monitoring, Urban Link 1 is chosen, which surrounds a relatively quiet area (the Olympic Stadium, education zones, and green parks). (b) The test scenario in campus. A part of armored fiber cable is placed through a speed bump of the road, from which we can localize vibrations of passing vehicles. (c) The test scenario on Urban Link 1. At the fourth ring road, a pedestrian underpass is in the way of the fiber link. Passing vehicles directly above this section generate vibrations which will be detected by the interferometry system.
Reference [15] adopts the cross-correlation method to localize earthquakes and realizes a localization accuracy of kilometer magnitude in the lab test. It is precise enough for earthquake detection but cannot meet the ultrahigh precision requirement for the scenario of urban vibration detection. Reference [26] uses a frequency-shifted optical delay line and realizes vibration localization on 615 km in-lab link. For vibration signals of 250 Hz, the localization accuracy is 553 m. Its accuracy cannot meet the requirement of ultrahigh precision localizing cases, and the frequency range is also not suitable for vibration events with low frequency (earthquake, traffic, etc.). Actually, cross correlation is a powerful method when evaluating the similarity of two time-infinite signals or time-finite period signals but will bring nonnegligible random error when dealing with aperiodic vibration signals [34]. This random error depends on the cutoff window of the detected signal. The detailed discussion is provided in Appendix A.
Sign up for Photonics Research TOC. Get the latest issue of Photonics Research delivered right to you!Sign up now
In this paper, we propose the time shifting deviation (TSDEV) method to replace the commonly used cross-correlation method. Actually, the TSDEV method is more precise and has fewer boundary conditions. In principle, it can realize ultrahigh precision time delay estimation with a random cutoff window. In addition to the theoretical analysis, we set up a laser interferometer to carry out a lab test. The localization accuracy of
2. EXPERIMENTAL SETUP
The experimental setup of the laser interferometer is shown in Fig. 2(a). The interferometer consists of three parts: the optical system, sensing ring, and data processing part. The optical system transmits input laser signals into the sensing ring, receives output signals, and converts them into RF beat signals by the photodiode (PD). The sensing ring is the fiber link under detection; in our case, we set up an in-lab link, campus link, and urban link to analyze vibrations along them. In the data processing part, RF signals are acquired by the data acquisition (DAQ) system and transformed into phase information, from which we can extract vibration signals and localize them.
Figure 2.Experimental setup of laser interferometer and the schematic diagram of vibration localization principle. (a) The vibration detection system consists of three parts: optical system, sensing ring, and data processing part. Optical system: laser (1550 nm laser module), acousto-optical modulator (AOM), synthesizer (providing shifter frequency for AOM), photodiode (PD). Sensing ring: in-lab test, campus test, and urban link test are carried out, respectively. Data processing part: data acquisition (DAQ) system, field programmable gate array (FPGA), computer for data processing (PC). (b) The principle of vibration localization is using the counter-propagating beams to detect the vibration event happening at
Starting from the laser source, the laser module we use is NKT Koheras BASIK X15, whose linewidth is
3. METHODS
A. Vibration Localization Principle
The vibration localization principle is illustrated in Fig. 2(b). The optical path length between the vibration point and PD 1 is
Using the time delay estimation method, we can extract the phase change time delay
Equation (1) shows that localization accuracy mainly depends on the accuracy of
B. Time Shifting Deviation Method
Actually, no matter how we improve the cross-correlation method, the estimation error will always exist [42]. Thus, we propose a novel time delay estimation method, TSDEV. Instead of the correlation coefficient in the cross-correlation method, we use the standard deviation of two signals’ difference
In Eq. (2),
In the localization case,
TSDEV is a more rigorous method than cross correlation. To make a further analysis of TSDEV, we carry out a calculation in Eq. (3). The phase changes
From Eq. (3), we can know that only if
In addition, the influence of background noise
4. RESULTS
A. In-Lab Demonstration
To demonstrate the advantages of the TSDEV method, we carry out an in-lab experiment and make a comparison between the results of TSDEV and the cross-correlation method. Fiber spools are utilized to form the sensing ring, as shown in Fig. 3(a). One is around 50 km (49.49 km), and the other spool
Figure 3.Diagram of the sensing ring and detection results of in-lab demonstration. (a) The sensing ring consists of a 50 km fiber spool, a fiber stretcher (FST), and a fiber spool
First, a 50–10 km scheme is under test (
Considering the cross-correlation method, we use different window sizes to localize the vibration mentioned above. Since the FST-induced vibration is single frequency, we cut off signal segments with integer multiples of half-period (Cutoff 1: 26 half-periods) and random length (Cutoff 2: 26.04 half-periods), respectively. Their results are shown in Fig. 3(c) to compare with the results of the TSDEV method. For those segments with Cutoff 1, the average position is 49,493.0 m away from the reference point and the standard deviation is 3.6 m. All results are centered within an error range of
Then, we extend the sensing ring to the 50–50 km scheme (
B. Field Test in Campus
To further demonstrate the superiority of the TSDEV method, we carry out a field test in the campus of Tsinghua University. As shown in Fig. 4(a), we connect the sensing ring to a part of the fiber cable in Tsinghua Campus, analyze vibration events induced by cars passing through the cable and localize them. The sensing ring consists of three parts: 50 km (49.49 km) fiber spool in lab, 800 m fiber cable in campus, and 10 km (9.84 km) fiber spool in lab. A piece of the 800 m armored fiber cable is placed through a speed bump.
Figure 4.Diagram of the sensing ring and detection results of the campus test. (a) The sensing ring consists of a 50 km fiber spool in lab, an 800 m fiber cable in campus, and a 10 km fiber spool in lab. A 9 m part of the 800 m fiber cable is placed through the speed bump of the campus road to detect passing vehicles running in Direction 1 and Direction 2. (b) The phase change detected by the CCW beam (blue) and the CW beam (red), with time delay
When cars drive across it, corresponding phase change [as shown in Fig. 4(b)] will allow us to extract and localize the vibrations. It is worth mentioning that the width of the road is 9 m, and cars from opposite directions (driving on the right) lead to different localization results. We divide the collected data into two parts in terms of driving direction and show localization results in Fig. 4(c). The average position of Direction 1 is 49,825.3 m away from the reference point, and the standard deviation is 8.4 m. The average position of Direction 2 is 49,830.4 m away from the reference point. Its standard deviation is 6.6 m. Obviously, the localized position of Direction 1 is closer to the reference point than that of Direction 2, which is in line with the setup of the sensing ring configuration in Fig. 4(a). The bias value 5.1 m between two center positions is also reasonable, considering the 9 m road width. While using the cross-correlation method, we cannot distinguish the vibrations in these two directions, and full details are provided in Appendix D.
C. Field Test on the Urban Link
To study the traffic cases in a larger district, we choose an urban fiber link [Fig. 5(a)] surrounding a relatively quiet area as the sensing ring. The link is buried underground deeply. It has a length of 31.42 km and passes through Olympic center, green parks, and education zones. As a preliminary study, this specific selection makes the results easy to analyze. We also connect a 10 km (9.84 km) fiber spool with Urban Link 1 as extension and make the sensing ring’s total length be 41.26 km.
Figure 5.Diagram of the sensing ring and detection results of the urban link test. (a) The sensing ring consists of a 10 km fiber spool in lab and a 31.42 km Urban Link 1. A vibration source (pedestrian underpass) is localized at point 15.749 km away from Tsinghua Campus, clockwise along the link. (b) Top part: the detected phase change from 21:00 to 3:00. Middle part: two segments of typical vibrations before 23:00 and after 24:00. Bottom part: sketch maps of traffic flow before and after 23:00.
In the top part of Fig. 5(b), the phase information shows us an interesting vibration sequence. Before midnight (21:00–23:00), vibrations behave as low-amplitude (
With the help of the urban fiber route diagram, we find out the location of the vibration source and discover a pedestrian underpass there, as shown in Fig. 5(a). At that point, urban fiber cable is deployed above the underpass and beneath the fourth ring road, much more shallowly than other places. Thus, the vibrations caused by passing vehicles can transmit to fiber cable without much attenuation. In addition, the soil between the ring road and the underpass forms a resonant cavity, which increases the detection sensitivity to some extent.
By monitoring the traffic at that point, the changing rule of vibrations is revealed. As shown in the bottom part of Fig. 5(b), we display two sketch maps which simply present the traffic flow cases. Before midnight, vehicles on the road are mainly cars and jeeps, which are light-weight and fast-running (corresponding to low-amplitude and high-frequency vibrations). After 23:00, big lorries are permitted to enter the downtown city; the corresponding regulation is provided in Appendix E. The lorries generate strong vibrations which lead to the length change of fiber cable up to 600 μm (
5. DISCUSSION
We obtain vibration information from the laser interferometer and use the TSDEV method to localize vibration events. The TSDEV method is more precise than the cross-correlation method. It has fewer boundary conditions, which leads to advantages in localization accuracy and practicability. The in-lab experiment demonstrates that localization accuracy is limited by the sampling rate of 40 MS/s. The standard deviations of localization results are
Initially, we want to choose a quiet urban fiber link to detect the background noise. Apart from the underpass, Urban Link 1 hardly feels vibrations on the rest of the link. Thus, vibrations at the underpass dominate and nearly submerge others. If we want to carry out further analysis, data processing such as filtering and signal extraction should be made in advance.
Further investigation will be focused on how to localize vibrations which occur at different positions of the link simultaneously. A procedure may be used to solve this problem. When several vibrations occur simultaneously, the minimum value of TSDEV will be larger than that of a single vibration. This could be an indicator to judge the number of vibrations. Then, the signal can be separated into different frequency bands. Because detected vibrations are actually broadband signals, we can always find a frequency band in which a single vibration dominates. TSDEV will be carried out in each frequency band, and the minimum values of TSDEV can help to judge whether it is a single vibration. If so, the corresponding time delay can be used to localize this vibration source. After going through all frequency bands, the localization of multiple vibrations can be realized.
Acknowledgment
Acknowledgment. We thank Professor Hongbo Sun of Tsinghua University and Dr. Pengbo Zhang of AutoNavi for fruitful discussions. We thank Jintao Yin of Beijing Gongjian Hengye Communication Technology Corporation Limited for arranging the urban fiber link.
APPENDIX A: ERROR ANALYSIS OF THE CROSS-CORRELATION METHOD
The commonly used cross-correlation method utilizes the expectation of
A simplified calculation is carried out as a preliminary demonstration, when we set the phase change to be pure sine wave:
In Eq. (
However, the derivative of
In Eq. (
The conclusion above will be more tightened when considering the normal case, in which phase changes are arbitrary signal segments. For time-finite signals
The fundamental frequency
It should be noted that the effective length of the sine series is
In Eq. (
Ideally,
From Eqs. (
APPENDIX B: MODIFIED TSDEV METHOD TO DECREASE THE INFLUENCE OF BACKGROUND NOISE
In Section
To make it clear, we separate background noise into two parts: related noise
In Eq. (
Hence, we propose a compensation method. We choose a segment of background noise near the signals, which can be written as
When background noise cannot be ignored, the TSDEV of background noise can be used to compensate the noise-induced error, and a precise estimation of time delay
In conclusion, the vibration localizing procedure is as follows. First, we demodulate the two counter-propagating beams and obtain phase change signals
Figure 6.Diagram of the localizing procedure, using the demodulated phase change signals
APPENDIX C: LOCALIZATION RESULTS OF 50–50?km SCHEME IN-LAB DEMONSTRATION
In Section
The results of the 50–50 km scheme are shown in Fig.
Figure 7.Detection results of the 50–50 km scheme in-lab demonstration. The red hollow circle points are the distribution frequency using the TSDEV method, and the red line is the fitted probability density curve.
APPENDIX D: LOCALIZATION RESULTS OF THE FIELD TEST IN CAMPUS USING THE CROSS-CORRELATION METHOD
In Section
Figure 8.Localization results of the campus test using the cross-correlation method. Red bars: the distribution frequency of Direction 1. Red line: the fitted probability density curve. Blue bars: the distribution frequency of Direction 2. Blue line: the fitted probability density curve.
APPENDIX E: LOCALIZATION RESULTS OF THE FIELD TEST ON URBAN LINK 1
In Section
Figure 9.Detection results of the urban link test. The blue bars are the distribution frequency of vibrations on Urban Link 1, and the blue line is the fitted probability density curve.
Figure 10.Photos of the traffic condition at the target vibration location. (a) The typical traffic picture before 23:00. Vehicles on the road are mainly light-weight cars, which will cause low-amplitude high-frequency fluctuations. (b) The typical traffic picture after 24:00. Cargo lorries are permitted to enter the downtown city, which will produce high-amplitude low-frequency shockwaves.
References
[1] L.-S. Ma, P. Jungner, J. Ye, J. L. Hall. Delivering the same optical frequency at two places: accurate cancellation of phase noise introduced by an optical fiber or other time-varying path. Opt. Lett., 19, 1777-1779(1994).
[2] N. R. Newbury, P. A. Williams, W. C. Swann. Coherent transfer of an optical carrier over 251 km. Opt. Lett., 32, 3056-3058(2007).
[3] M. Fujieda, M. Kumagai, S. Nagano, A. Yamaguchi, H. Hachisu, T. Ido. All-optical link for direct comparison of distant optical clocks. Opt. Express, 19, 16498-16507(2011).
[4] K. Predehl, G. Grosche, S. M. F. Raupach, S. Droste, O. Terra, J. Alnis, Th. Legero, T. W. Hänsch, Th. Udem, R. Holzwarth, H. Schnatz. A 920-kilometer optical fiber link for frequency metrology at the 19th decimal place. Science, 336, 441-444(2012).
[5] B. Wang, C. Gao, W. L. Chen, J. Miao, X. Zhu, Y. Bai, J. W. Zhang, Y. Y. Feng, T. C. Li, L. J. Wang. Precise and continuous time and frequency synchronisation at the 5 × 10−19 accuracy level. Sci. Rep., 2, 556(2012).
[6] D. Calonico, E. K. Bertacco, C. E. Calosso, C. Clivati, G. A. Costanzo, M. Frittelli, A. Godone, A. Mura, N. Poli, D. V. Sutyrin, G. Tino, M. E. Zucco, F. Levi. High-accuracy coherent optical frequency transfer over a doubled 642-km fiber link. Appl. Phys. B, 117, 979-986(2014).
[7] T. B. Gibbon, E. K. Rotich Kipnoo, R. R. G. Gamatham, A. W. R. Leitch, R. Siebrits, R. Julie, S. Malan, W. Rust, F. Kapp, T. L. Venkatasubramani, B. Wallace, A. Peens-Hough, P. Herselman. Fiber-to-the-telescope: MeerKAT, the South African precursor to square kilometre telescope array. J. Astron. Telesc. Instrum. Syst., 1, 028001(2015).
[8] C. Lisdat, G. Grosche, N. Quintin, C. Shi, S. M. F. Raupach, C. Grebing, D. Nicolodi, F. Stefani, A. Al-Masoudi, S. Dörscher, S. Häfner, J.-L. Robyr, N. Chiodo, S. Bilicki, E. Bookjans, A. Koczwara, S. Koke, A. Kuhl, F. Wiotte, F. Meynadier, E. Camisard, M. Abgrall, M. Lours, T. Legero, H. Schnatz, U. Sterr, H. Denker, C. Chardonnet, Y. Le Coq, G. Santarelli, A. Amy-Klein, R. Le Targat, J. Lodewyck, O. Lopez, P.-E. Pottie. A clock network for geodesy and fundamental science. Nat. Commun., 7, 12443(2016).
[9] D. Li, C. Qian, Y. Li, J. Y. Zhao. Efficient laser noise reduction method via actively stabilized optical delay line. Opt. Express, 25, 9071-9077(2017).
[10] C. Clivati, A. Tampellini, A. Mura, F. Levi, G. Marra, P. Galea, A. Xuereb, D. Calonico. Optical frequency transfer over submarine fiber links. Optica, 5, 893-901(2018).
[11] Y. He, K. G. H. Baldwin, B. J. Orr, R. B. Warrington, M. J. Wouters, A. N. Luiten, P. Mirtschin, T. Tzioumis, C. Phillips, J. Stevens, B. Lennon, S. Munting, G. Aben, T. Newlands, T. Rayner. Long-distance telecom-fiber transfer of a radio-frequency reference for radio astronomy. Optica, 5, 138-146(2018).
[12] S. Ebenhag, P. O. Hedekvist, C. Rieck, M. Bergroth, P. Krehlik, L. Sliwczynski. Evaluation of fiber optic time and frequency distribution system in a coherent communication network. Joint Conference of the IEEE International Frequency Control Symposium and European Frequency and Time Forum (EFTF/IFC), 1-5(2019).
[13] J. B. Ajo-Franklin, S. Dou, N. J. Lindsey, I. Monga, C. Tracy, M. Robertson, V. Rodriguez Tribaldos, C. Ulrich, B. Freifeld, T. Daley, X. Li. Distributed acoustic sensing using dark fiber for near-surface characterization and broadband seismic event detection. Sci. Rep., 9, 1328(2019).
[14] M. Karrenbach, S. Cole, L. LaFlame, E. Bozdağ, W. Trainor-Guitton, B. Luo. Horizontally orthogonal distributed acoustic sensing array for earthquake- and ambient-noise-based multichannel analysis of surface waves. Geophys. J. Int., 222, 2147-2161(2020).
[15] G. Marra, C. Clivati, R. Luckett, A. Tampellini, J. Kronjaeger, L. Wright, A. Mura, F. Levi, S. Robinson, A. Xuereb, B. Baptie, D. Calonico. Ultrastable laser interferometry for earthquake detection with terrestrial and submarine cables. Science, 361, 486-490(2018).
[16] P. Jousset, T. Reinsch, T. Ryberg, H. Blanck, A. Clarke, R. Aghayev, G. P. Hersir, J. Henninges, M. Weber, C. M. Krawczyk. Dynamic strain determination using fibre-optic cables allows imaging of seismological and structural features. Nat. Commun., 9, 2509(2018).
[17] E. F. Williams, M. R. Fernandez-Ruiz, R. Magalhaes, R. Vanthillo, Z. Zhan, M. Gonzalez-Herraez, H. F. Martins. Distributed sensing of microseisms and teleseisms with submarine dark fibers. Nat. Commun., 10, 5778(2019).
[18] A. Sladen, D. Rivet, J. P. Ampuero, L. De Barros, Y. Hello, G. Calbris, P. Lamare. Distributed sensing of earthquakes and ocean-solid Earth interactions on seafloor telecom cables. Nat. Commun., 10, 5777(2019).
[19] A. Mecozzi, M. Cantono, J. C. Castellanos, V. Kamalov, R. Muller, Z. Zhan. Polarization sensing using submarine optical cables. Optica, 8, 788-795(2021).
[20] Y. C. Guo, B. Wang, H. W. Si, Z. W. Cai, A. M. Zhang, X. Zhu, J. Yang, K. M. Feng, C. H. Han, T. C. Li, L. J. Wang. Correlation measurement of co-located hydrogen masers. Metrologia, 55, 631-636(2018).
[21] Y. C. Guo, B. Wang, F. M. Wang, F. F. Shi, A. M. Zhang, X. Zhu, J. Yang, K. M. Feng, C. H. Han, T. C. Li, L. J. Wang. Real-time free-running time scale with remote clocks on fiber-based frequency network. Metrologia, 56, 045003(2019).
[22] D. A. Jackson, A. Dandridge, S. K. Sheem. Measurement of small phase shifts using a single-mode optical-fiber interferometer. Opt. Lett., 5, 139-141(1980).
[23] Q. M. Chen, C. Jin, Y. Bao, Z. H. Li, J. P. Li, C. Lu, L. Yang, G. F. Li. A distributed fiber vibration sensor utilizing dispersion induced walk-off effect in a unidirectional Mach-Zehnder interferometer. Opt. Express, 22, 2167-2173(2014).
[24] J. W. Huang, Y. C. Chen, Q. H. Song, H. K. Peng, P. W. Zhou, Q. Xiao, B. Jia. Distributed fiber-optic sensor for location based on polarization-stabilized dual-Mach-Zehnder interferometer. Opt. Express, 28, 24820-24832(2020).
[25] Z. S. Sun, K. Liu, J. F. Jiang, T. H. Xu, S. Wang, H. R. Guo, T. G. Liu. High accuracy and real-time positioning using MODWT for long range asymmetric interferometer vibration sensors. J. Lightwave Technol., 39, 2205-2214(2021).
[26] Y. X. Yan, F. N. Khan, B. Zhou, A. P. T. Lau, C. Lu, C. J. Guo. Forward transmission based ultra-long distributed vibration sensing with wide frequency response. J. Lightwave Technol., 39, 2241-2249(2021).
[27] P. Healey. Statistics of Rayleigh backscatter from a single-mode fiber. IEEE Trans. Commun. Technol., 35, 210-214(1987).
[28] Y. L. Lu, T. Zhu, L. Chen, X. Y. Bao. Distributed vibration sensor based on coherent detection of phase-OTDR. J. Lightwave Technol., 28, 3242-3249(2010).
[29] H. J. Wu, X. R. Liu, Y. Xiao, Y. J. Rao. A dynamic time sequence recognition and knowledge mining method based on the hidden Markov models (HMMs) for pipeline safety monitoring with Φ-OTDR. J. Lightwave Technol., 37, 4991-5000(2019).
[30] N. J. Lindsey, T. C. Dawe, J. B. Ajo-Franklin. Illuminating seafloor faults and ocean dynamics with dark fiber distributed acoustic sensing. Science, 366, 1103-1107(2019).
[31] P. Lu, N. Lalam, M. Badar, B. Liu, B. T. Chorpening, M. P. Buric, P. R. Ohodnicki. Distributed optical fiber sensing: review and perspective. Appl. Phys. Rev., 6, 041302(2019).
[32] F. Walter, D. Gräff, F. Lindner, P. Paitz, M. Köpfli, M. Chmiel, A. Fichtner. Distributed acoustic sensing of microseismic sources and wave propagation in glaciated terrain. Nat. Commun., 11, 2436(2020).
[33] Y. Y. Yang, Y. Li, T. J. Zhang, Y. Zhou, H. F. Zhang. Early safety warnings for long-distance pipelines: a distributed optical fiber sensor machine learning approach. Proceedings of the AAAI Conference on Artificial Intelligence, 35, 14991-14999(2021).
[34] S. R. Xie, Q. L. Zou, L. W. Wang, M. Zhang, Y. H. Li, Y. B. Liao. Positioning error prediction theory for dual Mach-Zehnder interferometric vibration sensor. J. Lightwave Technol., 29, 362-368(2011).
[35] G. Wang, H. W. Si, Z. W. Pang, B. H. Zhang, H. Q. Hao, B. Wang. Noise analysis of the fiber-based vibration detection system. Opt. Express, 29, 5588-5597(2021).
[36] G. C. Carter. Coherence and time delay estimation. Proc. IEEE, 75, 236-255(1987).
[37] X. J. Fang. Fiber-optic distributed sensing by a two-loop Sagnac interferometer. Opt. Lett., 21, 444-446(1996).
[38] P. F. Du, S. Zhang, C. Chen, A. Alphones, W. D. Zhong. Demonstration of a low-complexity indoor visible light positioning system using an enhanced TDOA scheme. IEEE Photonics J., 10, 7905110(2018).
[39] J. W. Dong, B. Wang, C. Gao, Y. C. Guo, L. J. Wang. Highly accurate fiber transfer delay measurement with large dynamic range. Opt. Express, 24, 1368-1375(2016).
[40] H. W. Si, B. Wang, J. W. Dong, L. J. Wang. Accurate self-calibrated fiber transfer delay measurement. Rev. Sci. Instrum., 89, 083117(2018).
[41] Q. Li, S. X. Xu, J. W. Yu, L. J. Yan, Y. M. Huang. An improved method for the position detection of a quadrant detector for free space optical communication. Sensors, 19, 175(2019).
[42] S. R. Xie, M. Zhang, Y. H. Li, Y. B. Liao. Positioning error reduction technique using spectrum reshaping for distributed fiber interferometric vibration sensor. J. Lightwave Technol., 30, 3520-3524(2012).
[43] A. Bercy, F. Stefani, O. Lopez, C. Chardonnet, P.-E. Pottie, A. Amy-Klein. Two-way optical frequency comparisons at 5 × 10−21 relative stability over 100-km telecommunication network fibers. Phys. Rev. A, 90, 061802(2014).
[44] L. Hu, X. Y. Tian, G. L. Wu, J. G. Shen, J. P. Chen. Fundamental limitations of Rayleigh backscattering noise on fiber-based multiple-access optical frequency transfer(2020).
[45] V. K. Madissetti. Digital Signal Processing Handbook(2010).
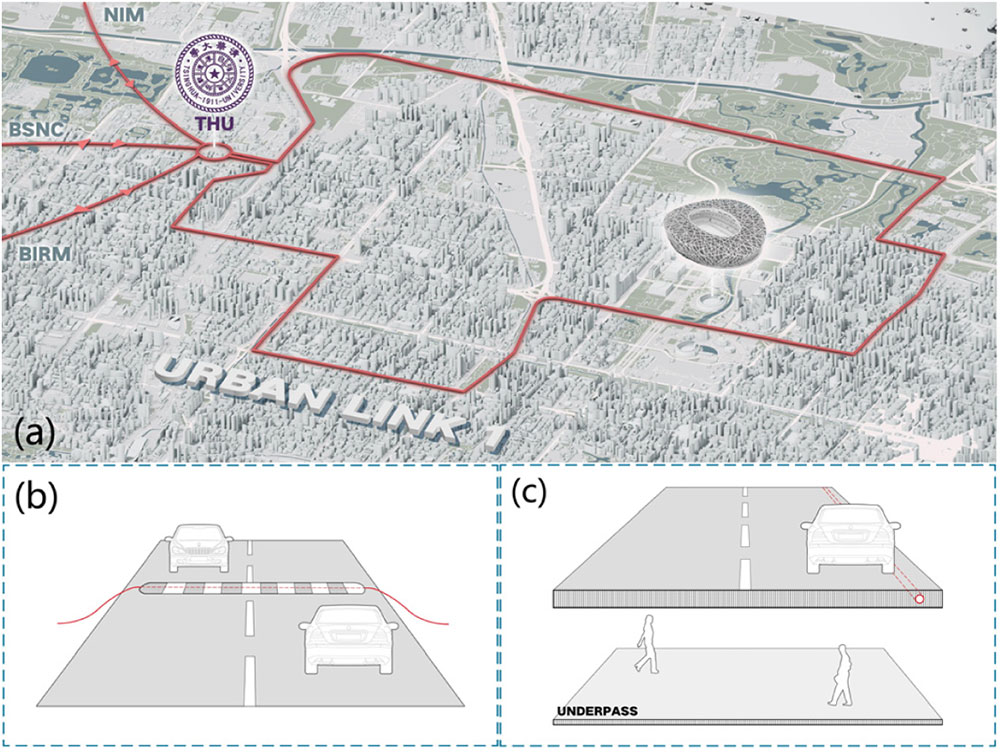
Set citation alerts for the article
Please enter your email address