Hanchu Ye, Zitong Ye, Yunbo Chen, Jinfeng Zhang, Xu Liu, Cuifang Kuang, Youhua Chen, Wenjie Liu, "Video-level and high-fidelity super-resolution SIM reconstruction enabled by deep learning," Adv. Imaging 1, 011001 (2024)

Search by keywords or author
- Advanced Imaging
- Vol. 1, Issue 1, 011001 (2024)
Abstract
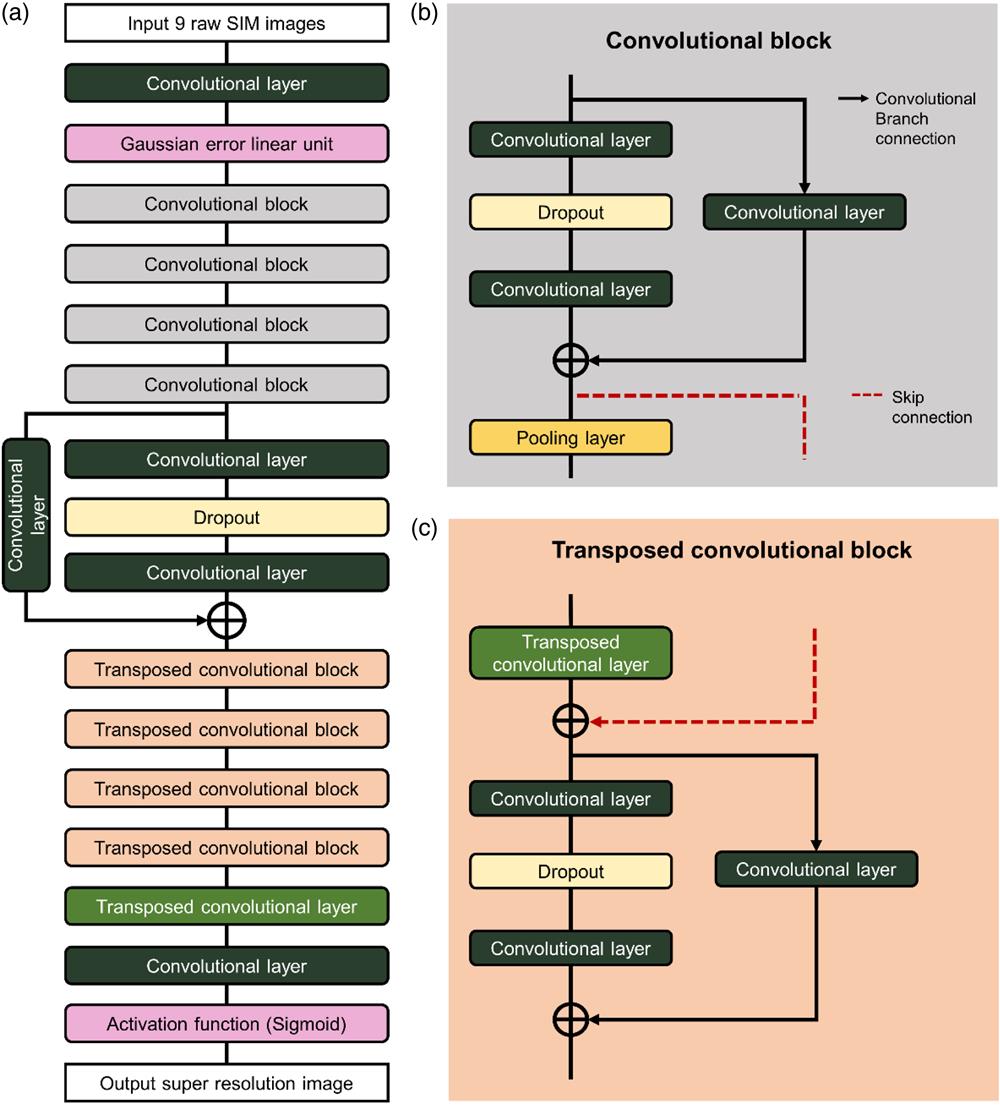
Set citation alerts for the article
Please enter your email address