Guoqing Jing, Peipei Wang, Haisheng Wu, Jianjun Ren, Zhiqiang Xie, Junmin Liu, Huapeng Ye, Ying Li, Dianyuan Fan, Shuqing Chen, "Neural network-based surrogate model for inverse design of metasurfaces," Photonics Res. 10, 1462 (2022)

Search by keywords or author
- Photonics Research
- Vol. 10, Issue 6, 1462 (2022)
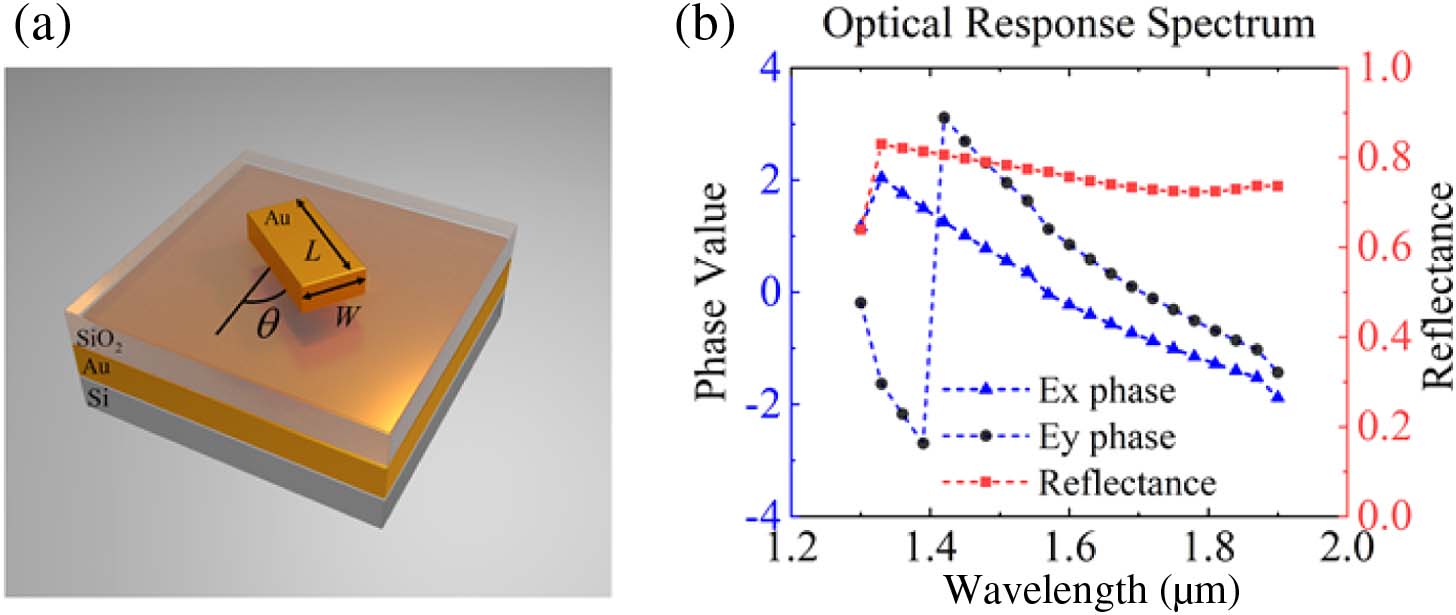
Fig. 1. (a) Unit cell of reflection-type metasurface. (b) Optical response spectrum data simulated using the FDTD method.
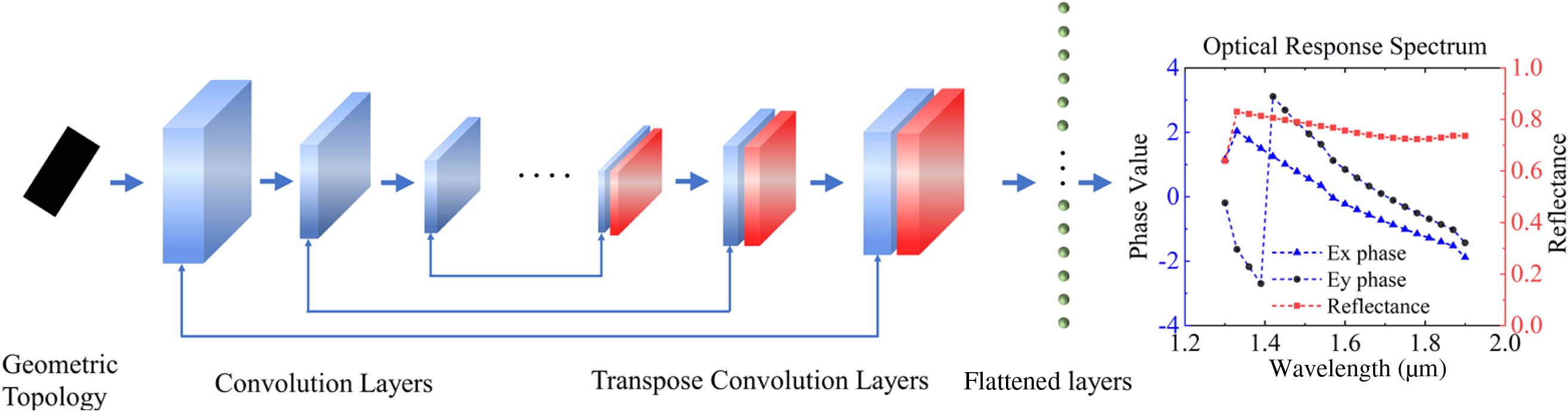
Fig. 2. Diagram of physics-based NNSM.
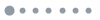
Fig. 3. Schematic of the inverse design process of the intelligent optimization algorithm.
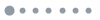
Fig. 4. Predicted results of NNSM. (a) Loss curve as a function of training iteration. (b) Phase spectrum in x polarization. (c) Phase spectrum in y polarization. (d) Reflectance spectrum.
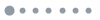
Fig. 5. (a) Comparison between the predicted results by the inverse design model and the ideal results calculated by FDTD method. (b) SEM image of the fabricated metasurfaces. (c) First line represents the intensity distributions of vortex beams with different OAM modes generated by Gaussian beam with different incident angles and polarization states, and the second line represents the intensity distributions of vortex beams after passing through the C-lens.
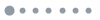
Fig. 6. Results of metasurfaces-based four channels OAM multiplexing communication. (a) BER as a function of received power for different OAM modes. (b) Signal and noise powers corresponding to OAM multiplexing. (c) Constellations of different channels at received optical powers of 20 dBm and 24.5 dBm.
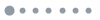
Fig. 7. Test results of the metasurface at different wavelengths (1500 nm, 1550 nm, 1600 nm). Top row, far-field light intensity distributions of right circularly polarized plane waves. Bottom row, detection results of projecting a vortex beam with l = − 1 on the OAM generator.
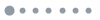
Fig. 8. Specific architecture of physics-based NNSM.
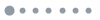
Fig. 9. Metasurfaces-based four channels OAM multiplexing communication link. PC, polarization controller; IQ Mod., in-phase/quadrature modulator; EDFA, erbium-doped fiber amplifier; AWG, arbitrary waveform generator; BPF, bandpass filter; OC, optical coupler; SMF, single-mode fiber; Att., attenuator; LO, local oscillator; ICR, integrated coherent receiver; DSO, digital sampling oscilloscope.
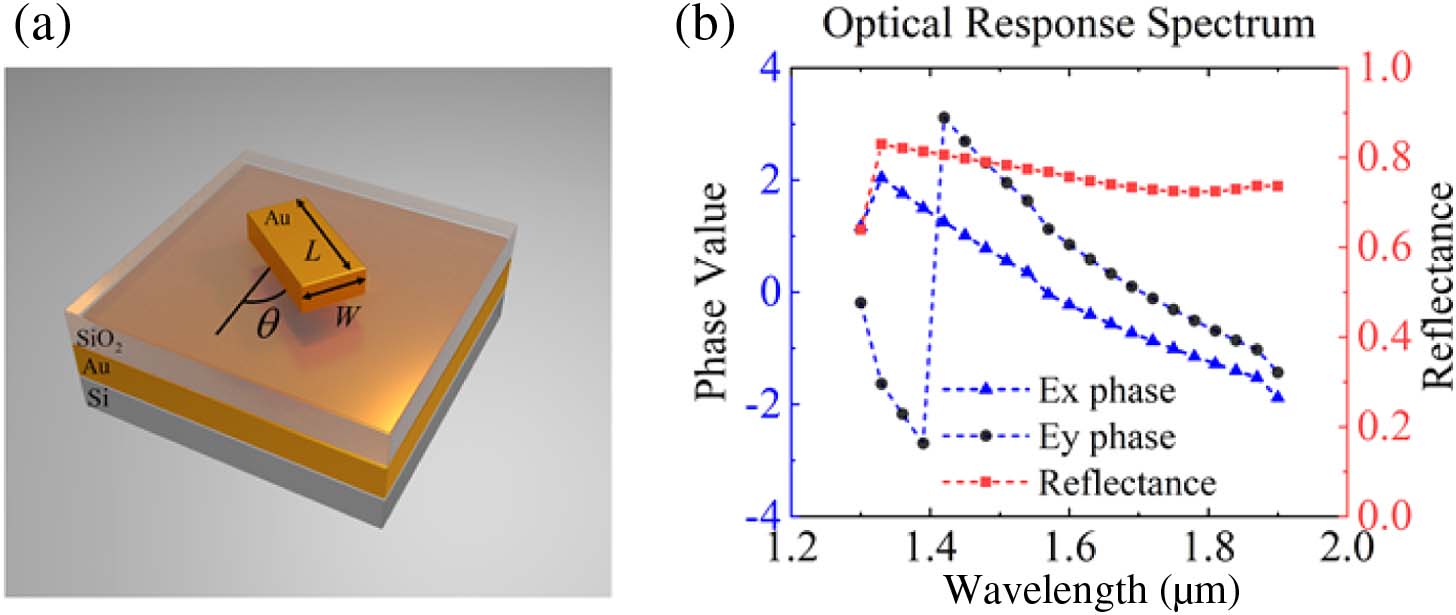
Set citation alerts for the article
Please enter your email address