Shuang LIU, Hai-ye YU, Zhao-jia PIAO, Mei-chen CHEN, Tong YU, Li-juan KONG, Lei ZHANG, Jing-min DANG, Yuan-yuan SUI. Study on Extracting Characteristic Wavelength of Soybean Physiological Information Based on Hyperspectral Technique[J]. Spectroscopy and Spectral Analysis, 2020, 40(11): 3542

Search by keywords or author
- Spectroscopy and Spectral Analysis
- Vol. 40, Issue 11, 3542 (2020)
Abstract
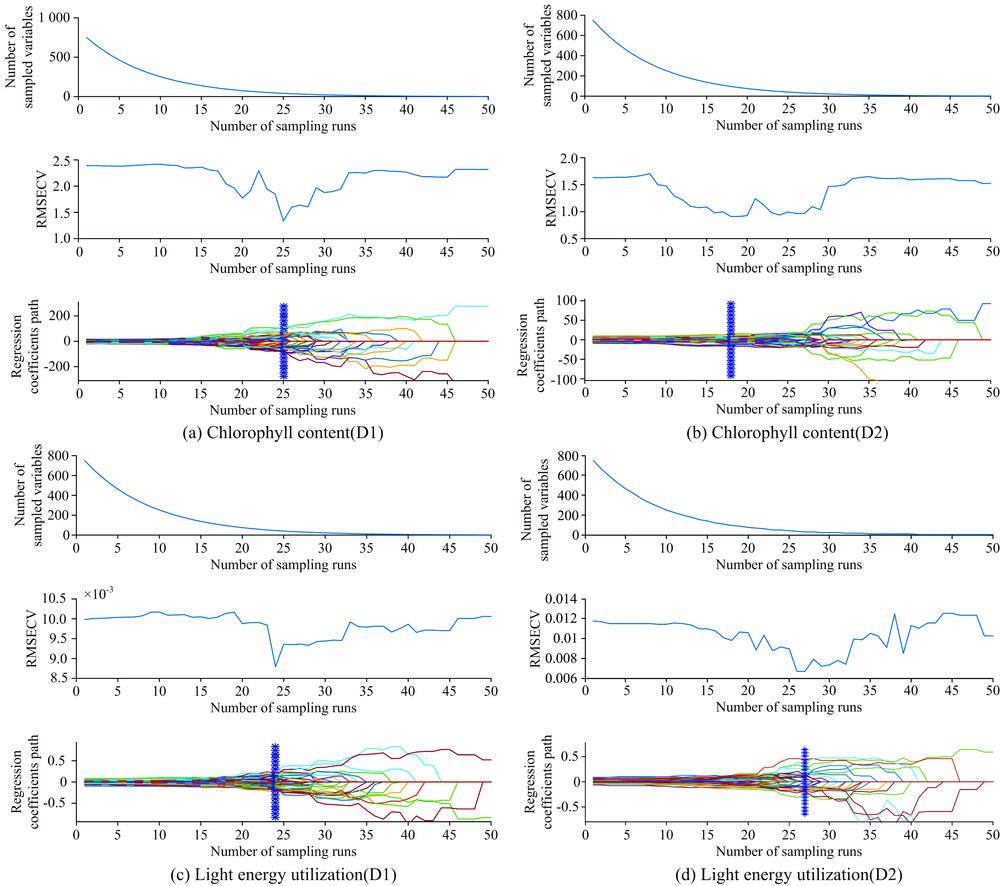
Set citation alerts for the article
Please enter your email address