Pengfei Xu, Zhiping Zhou, "Silicon-based optoelectronics for general-purpose matrix computation: a review," Adv. Photon. 4, 044001 (2022)

Search by keywords or author
- Advanced Photonics
- Vol. 4, Issue 4, 044001 (2022)
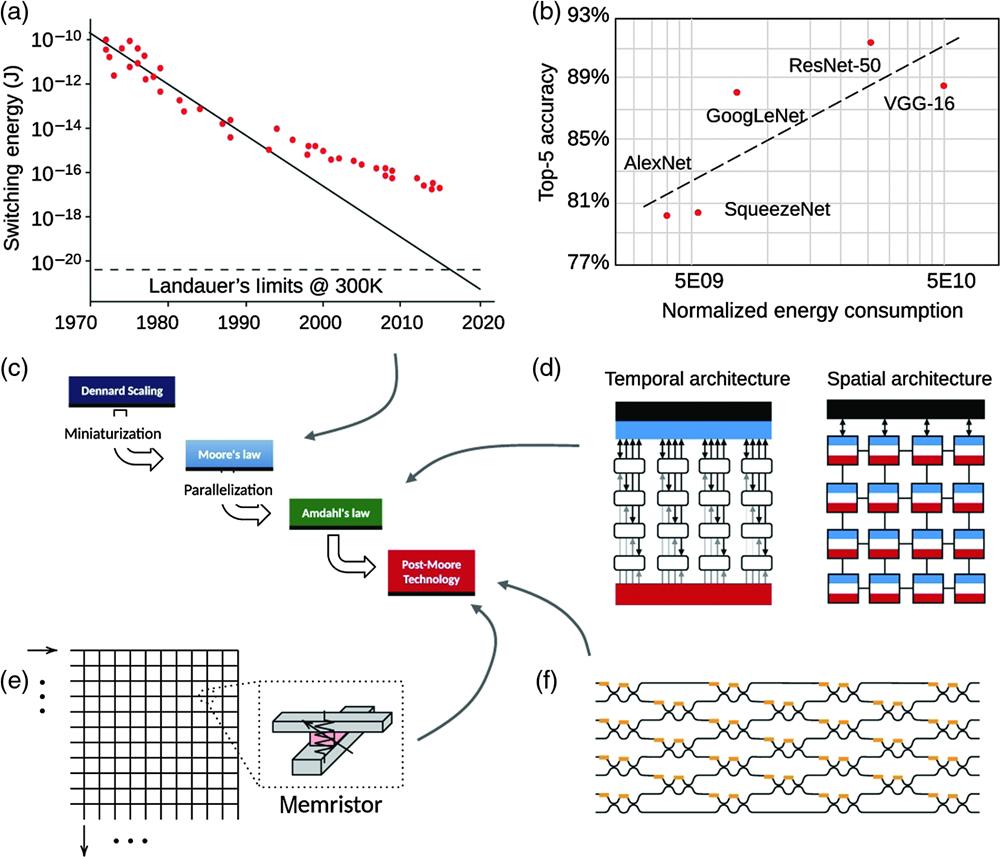
Fig. 1. Development of processors for matrix computation. (a) Moore’s law no longer seems applicable.16 (b) Exponential growth of energy consumption for more accurate ANN models.17 (c) Development trends of processors.18 (d) Temporal and spatial architectures for multicore parallelization.17 (e) Memristor crossbar arrays in post-Moore’s law era.19 (f) Integrated waveguide meshes for general-purpose matrix computation.20
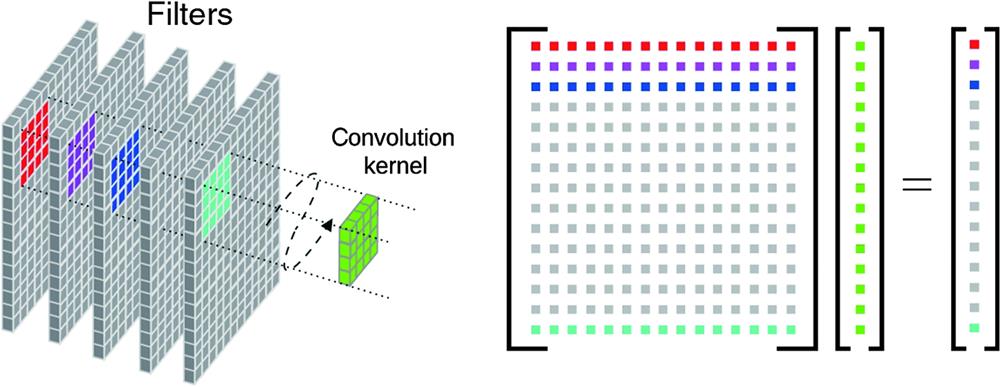
Fig. 2. Intuitive visualization showing energy-efficient models processing in the convolutional neural network. The CONV between the filters and kernel can be deployed into the MVM to improve efficiency.
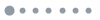
Fig. 3. Integrated waveguide meshes: from QIP to MVM. (a) Bulk-optical CNOT gate in 2004.29 (b) On-chip photonic CNOT gates in 2007.30 (c) Programmable quantum processor in 2016.31 (d) Large-scale photonic processor for arbitrary two-qubit operations.32 (e) Large-scale photonic processor for multidimensional quantum entanglement.33 (f) Schematic of optical switch topologies in the data center.34 (g) Reconfigurable hexagonal mesh for programmable signal processing.35 (h) Photonic “FPGA” for programmable radiofrequency signal processing.36 (i) Self-configuring -port linear processor. (j) First optical computing processor for vowel recognition.20 (k) Large scale MVM processor.37,38 (l) FFTNet architecture for better fault tolerance against imprecise components.39 (m) Redundant architecture to overcome fabrication errors.40
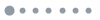
Fig. 4. Multiple laser source MVM. (a) MVM based on microring modulators.54 (b) MVM based on on-chip photorefractive interaction.55,56 (c) Photonic tensor core constituted by dot-product engines.57 (d) Photonic crossbar arrays with phase-change material.58
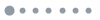
Fig. 5. FT-based CONV. (a) Flowchart of CONV using FT. (b) FT based on MMI coupler and compensating phase shifter arrays.59 (c) FT operation with -star coupler.60 (d) Compact surface plasmon polaritons device for FT.61 (e) CONV based on Cooley–Tukey method FT.62
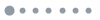
Fig. 6. Element-wise MAC operations. (a) Basic CONV consisting of nine MAC operations. (b) MAC based on balanced homodyne detection.63 (c) MAC based on cascaded acousto-optical modulators.64 (d) MAC based on microring modulators.65
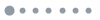
Fig. 7. MAC based on dispersion. (a) The 118 GigaMAC/s matrix operation is realized by 1.1-km long linear dispersion fiber.66 (b) 11.9 GigaFLOPs/s MAC conducted with 13-km spool of standard single-mode fiber.67 (c) Time-stretch method for MAC operations.68 (d) Temporal CONV (a series of MAC) by spiral waveguide with linear group dispersion.69
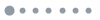
Fig. 8. Interconnections in processors, memory, and peripheral hardware. (a) The memory-processor interconnections are one of the major factors influencing the overall performance and the memory wall problem that has hindered high-performance computing.70 (b) Large-scale matrix multiplication is decomposed into small-scale matrix multiplications while processing.71 (c) On-chip optical transceivers are good alternatives for low-energy-budget interconnections and boosting the data movement among the computation hardware.72
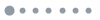
Fig. 9. Optical computing from bulk-optics to photonic-electronic integration. (a) SLM-based MVM processor released by Enlight in 2003.82 (b) Bulk-optical 4f-system for convolutional neural network.83 (c) Diffractive deep neural network by 3D-printed multi-layer phase mask.84 (d) 3D copackaged module for enhancing the interaction between the photonic core and electronic ASIC.34
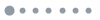
Fig. 10. Energy efficiency of silicon-based optoelectronic matrix computation processor (consider all the photonic, optoelectronic, and electronic devices and circuits). (a) The equivalent energy efficiency (energy consumption per MAC operation) linearly decreases as the side-length of the matrix increases.63 (b) Expectations of future compute density and energy efficiency in silicon-based optoelectronic matrix computation (the energy efficiency depends on the matrix configuration).
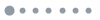
Fig. 11. Photonic matrix computation can be used for solving some difficult problems and reducing their time complexity. (a) Heuristic recurrent algorithm for the annealing of Ising models. (b) Reconstruction of -sparse signals in compressed sensing. (c) Very large-scale discrete Fourier transform.
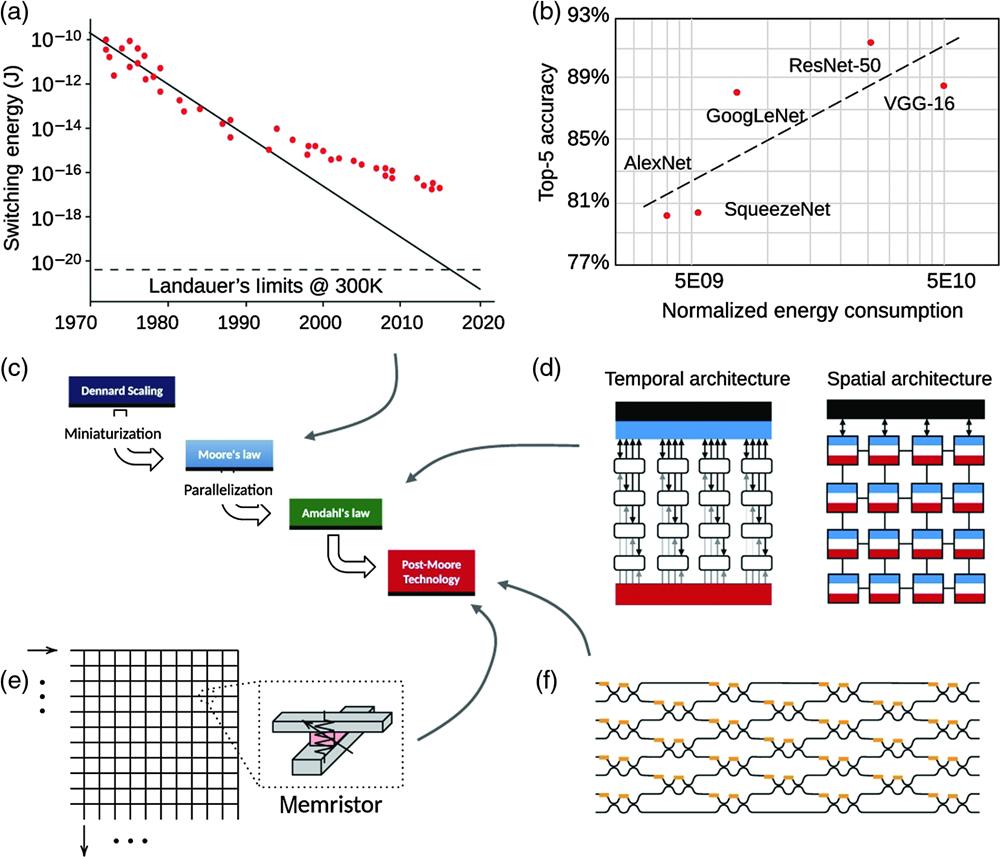
Set citation alerts for the article
Please enter your email address