Axin Fan, Tingfa Xu, Geer Teng, Xi Wang, Chang Xu, Yuhan Zhang, Xin Xu, Jianan Li, "Deep learning reconstruction enables full-Stokes single compression in polarized hyperspectral imaging," Chin. Opt. Lett. 21, 051101 (2023)

Search by keywords or author
- Chinese Optics Letters
- Vol. 21, Issue 5, 051101 (2023)
Abstract
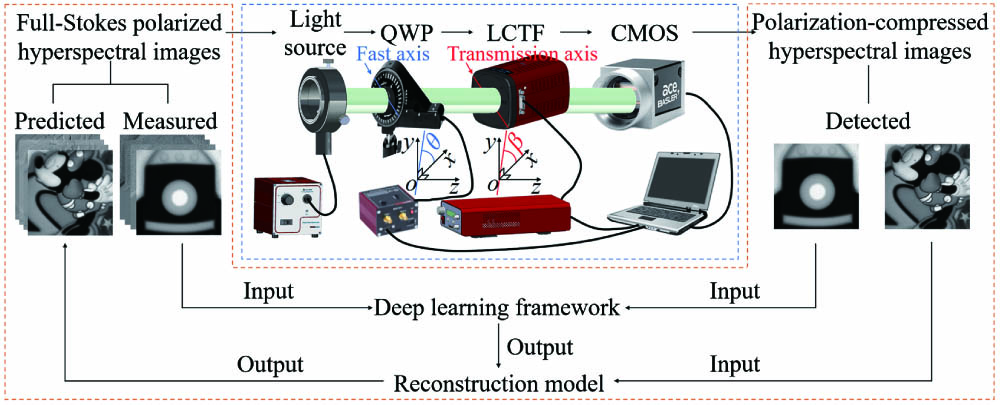
Set citation alerts for the article
Please enter your email address