
- Chinese Optics Letters
- Vol. 21, Issue 5, 051101 (2023)
Abstract
1. Introduction
Due to the rich information reflected, polarized hyperspectral imaging has been widely applied in environmental monitoring[1], biological diagnosis[2], food safety[3], and other fields. In terms of technological development, polarized imaging is mainly based on Fourier transform[4], pixelated polarizers[5], and compressive sensing (CS)[6]. Currently, all the above three methods can achieve full-Stokes polarized imaging.
Typically, Fourier transform imaging spectropolarimetry based on polarization modulation array (PMAFTISP)[7] requires only one acquisition to obtain full-Stokes images. The PMAFTISP includes three polarization modulation arrays and three independent optical elements. System complexity and channel crosstalk may affect imaging quality. In addition, pixelated full-Stokes polarimeters require rotating polarizers[8] or designing metasurfaces[9,10]. Moreover, the fabrication of precision pixelated devices is costly and time-consuming.
Recently, compressive full-Stokes polarimeters are constructed with only two commercial components, providing an easy-to-operate and time-saving system. Full-Stokes images can be reconstructed from two measurements compressed by a quarter-wave plate (QWP) and a liquid crystal tunable filter (LCTF)[11–13]. Furthermore, benefiting from a retarder followed by a Wollaston prism with a splitting effect, full-Stokes images can be reconstructed from one measurement[14]. Nevertheless, the above compressive polarimeters all rely on traditional reconstruction methods, such as the two-step iterative shrinkage/threshold (TwIST) algorithm[15], which require careful selection of polarization parameters and sparse basis.
Sign up for Chinese Optics Letters TOC. Get the latest issue of Chinese Optics Letters delivered right to you!Sign up now
This work develops full-Stokes single compression in polarized hyperspectral imaging by introducing deep learning reconstruction (DL-FSCPHI). Full-Stokes images are compressed by a QWP and an LCTF into only one measurement. In addition, the deep learning method can efficiently reconstruct full-Stokes images in one step, avoiding sparse basis selection.
2. DL-FSCPHI Method Overview
Figure 1 illustrates the overall schematic diagram of the DL-FSCPHI method comprising imaging system and polarization reconstruction. The imaging system mainly consists of a light source (Thorlabs, OSL2), a QWP (Thorlabs, SAQWP05M-700), an LCTF (Thorlabs, KURIOS-VB1/M), and a complementary metal oxide semiconductor (CMOS) detector (Basler, acA2040-180km). The polarization state of light can be expressed by four Stokes parameters. The polarization characteristics of an optical device can be described by a Mueller matrix with 16 elements in four rows and four columns. The interaction between light and optical devices is then reflected in the fact that optical devices can adjust the polarization state of light. Mathematically, the Mueller matrix of an optical device is multiplied by the four Stokes parameters of the input light to obtain the four Stokes parameters of the output light.
Figure 1.Overall schematic diagram of DL-FSCPHI method.
The Mueller matrices of the QWP and the LCTF are respectively expressed as
The four Stokes parameters of target light are modulated by the system Mueller matrix. Then, the modulated first Stokes parameter representing the total light intensity is detected by the CMOS. By fixing the angles of the QWP and the LCTF and by switching the center wavelength of the LCTF, a set of polarization-compressed hyperspectral images are obtained for each target.
The polarization reconstruction is divided into two steps: model training and model testing. The model is trained using a deep learning framework based on measured full-Stokes images and detected images. The trained model is then used to predict the unmeasured full-Stokes images from the detected images.
3. DL-FSCPHI Method Verification
The feasibility of the DL-FSCPHI method is verified by laboratory measurements of full-Stokes polarized spectral images. The verification process mainly involves measuring full-Stokes images as ground-truth values and designing reconstruction strategy.
3.1. Full-Stokes images measurement
First, full-Stokes images are measured by establishing an imaging system with a light source (Thorlabs, OSL2), a QWP (Thorlabs, SAQWP05M-700), a linear polarizer (LP) (Thorlabs, LPVISC100-MP2), multiple narrowband filters (Thorlabs, FB520-10, FB530-10, …, FB690-10), and a CMOS detector (Basler, acA2040-180km). The transmission axis angle of the LP is
Combined with the Mueller matrix of the QWP in Eq. (1), the polarization measurement matrix of the system is denoted as
A total of 18 spectral bands from 520 nm to 690 nm at 10 nm intervals are obtained by switching filters. At each spectral band, the full-Stokes images are acquired by five polarization measurements. In the five measurements, the fast axis of the QWP is rotated to 0°, 22.5°, 45°, 67.5°, and 90°, respectively, and the transmission axis of the LP is fixed at 45°. The polarized light intensities detected by the CMOS are denoted as
Obviously, full-Stokes polarized multispectral images measured in the laboratory can reflect the unique polarization distribution of each target. Therefore, laboratory measurements are better suited to validating the proposed DL-FSCPHI method by avoiding inaccurate assumptions about polarization distribution based on polarization simulation strategies[16,17].
3.2. Reconstruction strategy design
Figure 2 shows the reconstruction strategy proposed in this work. In the DL-FSCPHI method, the QWP angle
Figure 2.The reconstruction strategy proposed in this work.
First, set epoch, batch size, and initial learning rate for the model training. Let
Based on the trained model, the full-Stokes polarized hyperspectral images of the
4. Results and Discussion
To meet the model training requirements, we measure the full-Stokes images with
Figure 3.Measured and reconstructed full-Stokes images of three test targets in 6 spectral bands from 560 nm to 660 nm with an interval of 20 nm. The reconstructed images are marked with the PSNR and the SSIM values.
In the DL-FSCPHI method, the fast axis of the QWP is randomly rotated to 114°, and the incidence axis of LCTF is 0°. The reconstruction model consists of two convolutional layers. The first layer has 4 convolution kernels with the size of
Figure 4.PSNR and SSIM values of the reconstructed full-Stokes images of the three test targets in 18 spectral bands ranging from 520 nm to 690 nm at intervals of 10 nm.
To further demonstrate the robustness of the DL-FSCPHI method, the fast axis of the QWP is again randomly rotated to 27°. In addition, the two convolutional layers of the model are adjusted to 8 convolution kernels with the size of
θ = 27°, β = 0° | DL-M1 | DL-M2 | TwIST | |||
---|---|---|---|---|---|---|
Evaluation metrics | Epoch = 20 | Epoch = 40 | Epoch = 20 | Epoch = 40 | Accuracy = 0.005 | |
Batch size = 7 | Batch size = 5 | Batch size = 7 | Batch size = 5 | |||
PSNR/dB | S0 | 37.58 | 38.04 | 38.95 | 38.76 | 29.37 |
S1 | 22.17 | 22.62 | 22.06 | 22.27 | 10.96 | |
S2 | 24.87 | 25.17 | 24.38 | 25.22 | 10.37 | |
S3 | 32.63 | 33.57 | 31.20 | 32.19 | 9.85 | |
Average | 29.31 | 29.85 | 29.15 | 29.61 | 15.14 | |
SSIM | S0 | 1.00 | 1.00 | 1.00 | 1.00 | 1.00 |
S10 | 0.80 | 0.82 | 0.80 | 0.81 | 0.52 | |
S2 | 0.89 | 0.90 | 0.87 | 0.88 | 0.52 | |
S3 | 0.98 | 0.98 | 0.97 | 0.97 | 0.52 | |
Average | 0.92 | 0.92 | 0.91 | 0.92 | 0.64 |
Table 1. Average PSNR and SSIM Values of the Reconstructed Full-Stokes Images of 7 Test Targets in 18 Spectral Bands under Different Settings, Including Two Sets of Polarization Angles (
Figure 5.Loss curves of the training models under different settings, including two sets of training parameters (epoch = 20, batch size = 7 and epoch = 40, batch size = 5), two sets of polarization angles (θ = 114°, β = 0° and θ = 27°, β = 0°), and two convolution models (DL-M1 and DL-M2).
5. Conclusion
In conclusion, this work comprehensively introduces the DL-FSCPHI method to achieve full-Stokes single compression with deep learning reconstruction. A QWP followed by an LCTF constitutes the polarization-compressed hyperspectral imaging system with the fewest critical components, the highest compression rate, and no moving parts. The full-Stokes images are compressed in one snapshot by fixing the fast axis angle of the QWP and the incidence axis angle of the LCTF. Meanwhile, the deep learning-based reconstruction strategy is proposed to simultaneously obtain full-Stokes images from one compressed image. Furthermore, the feasibility and effectiveness of the DL-FSCPHI method are fully verified based on extensive laboratory measurements. Compared with the traditional TwIST algorithm, the proposed deep learning method significantly improves the reconstruction effect of the last three Stokes parameters in terms of image quality and evaluation metrics. The test results also verify the wide applicability of the reconstruction strategy. This work demonstrates great promise for developing deep learning reconstruction for full-Stokes single compression and other applications.
References
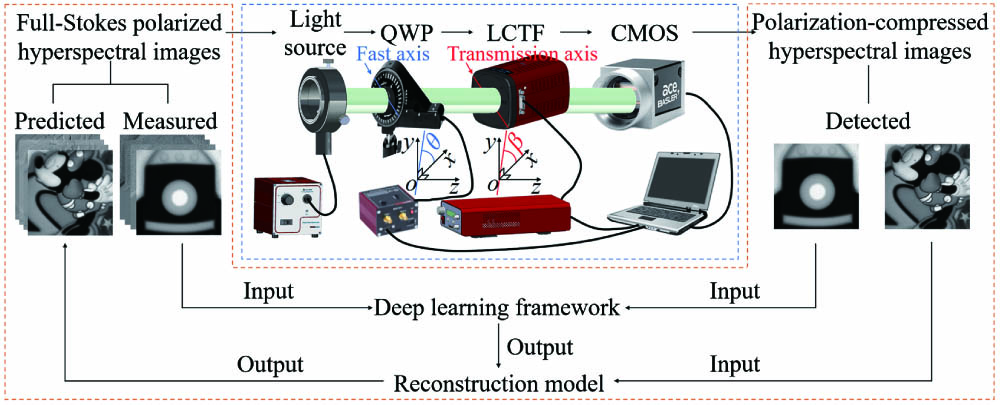
Set citation alerts for the article
Please enter your email address