Chen Bai, Tong Peng, Junwei Min, Runze Li, Yuan Zhou, Baoli Yao, "Dual-wavelength in-line digital holography with untrained deep neural networks," Photonics Res. 9, 2501 (2021)

Search by keywords or author
- Photonics Research
- Vol. 9, Issue 12, 2501 (2021)
Abstract
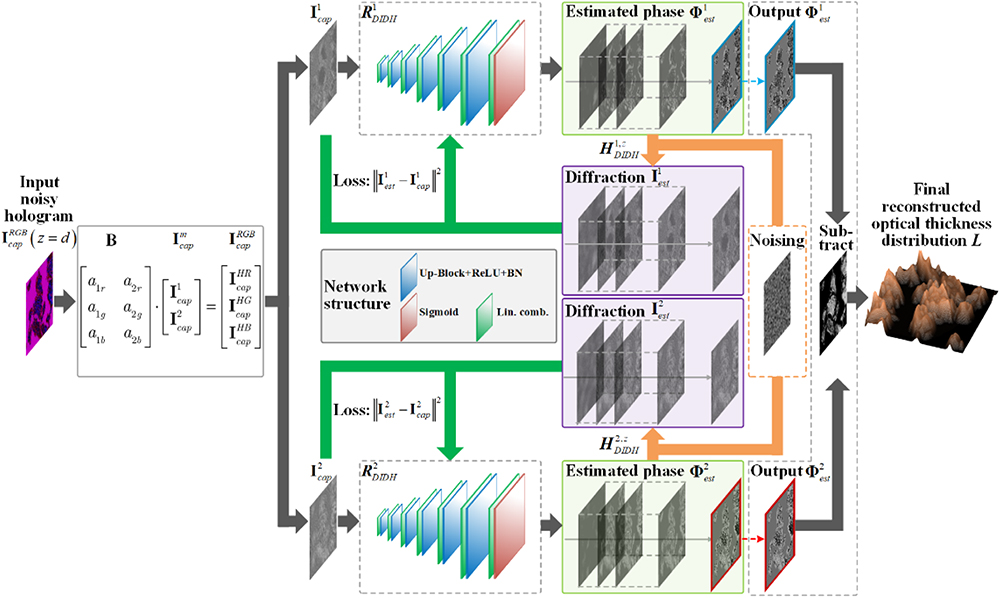
Set citation alerts for the article
Please enter your email address