Xiao-qin LIAN, Qun CHEN, Shen-miao TANG, Jing-zhu WU, Ye-lan WU, Chao GAO. Quantitative Analysis Method of Key Nutrients in Lanzhou Lily Based on NIR and SOM-RBF[J]. Spectroscopy and Spectral Analysis, 2022, 42(7): 2025

Search by keywords or author
- Spectroscopy and Spectral Analysis
- Vol. 42, Issue 7, 2025 (2022)
Abstract
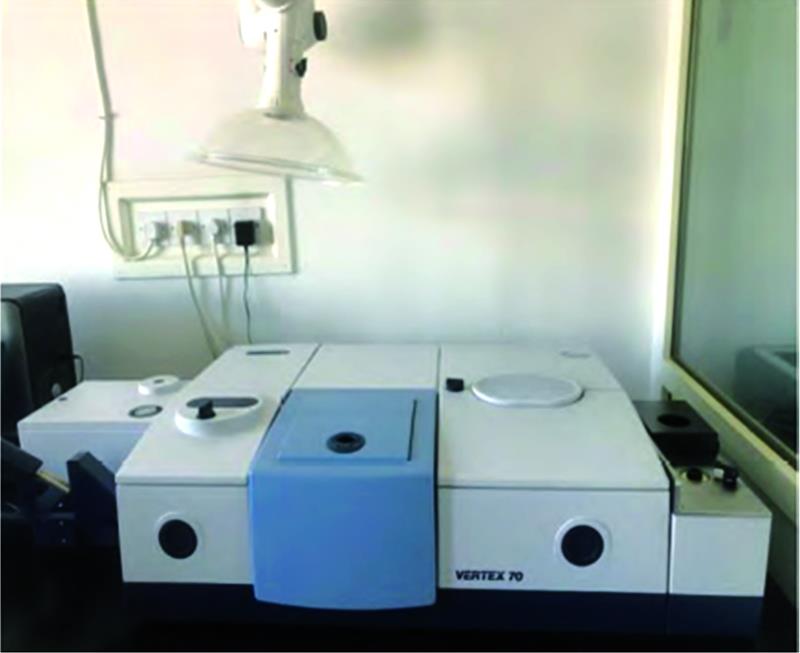
Set citation alerts for the article
Please enter your email address