Yuezhi He, Jing Yao, Lina Liu, Yufeng Gao, Jia Yu, Shiwei Ye, Hui Li, Wei Zheng, "Self-supervised deep-learning two-photon microscopy," Photonics Res. 11, 1 (2023)

Search by keywords or author
- Photonics Research
- Vol. 11, Issue 1, 1 (2023)
Abstract
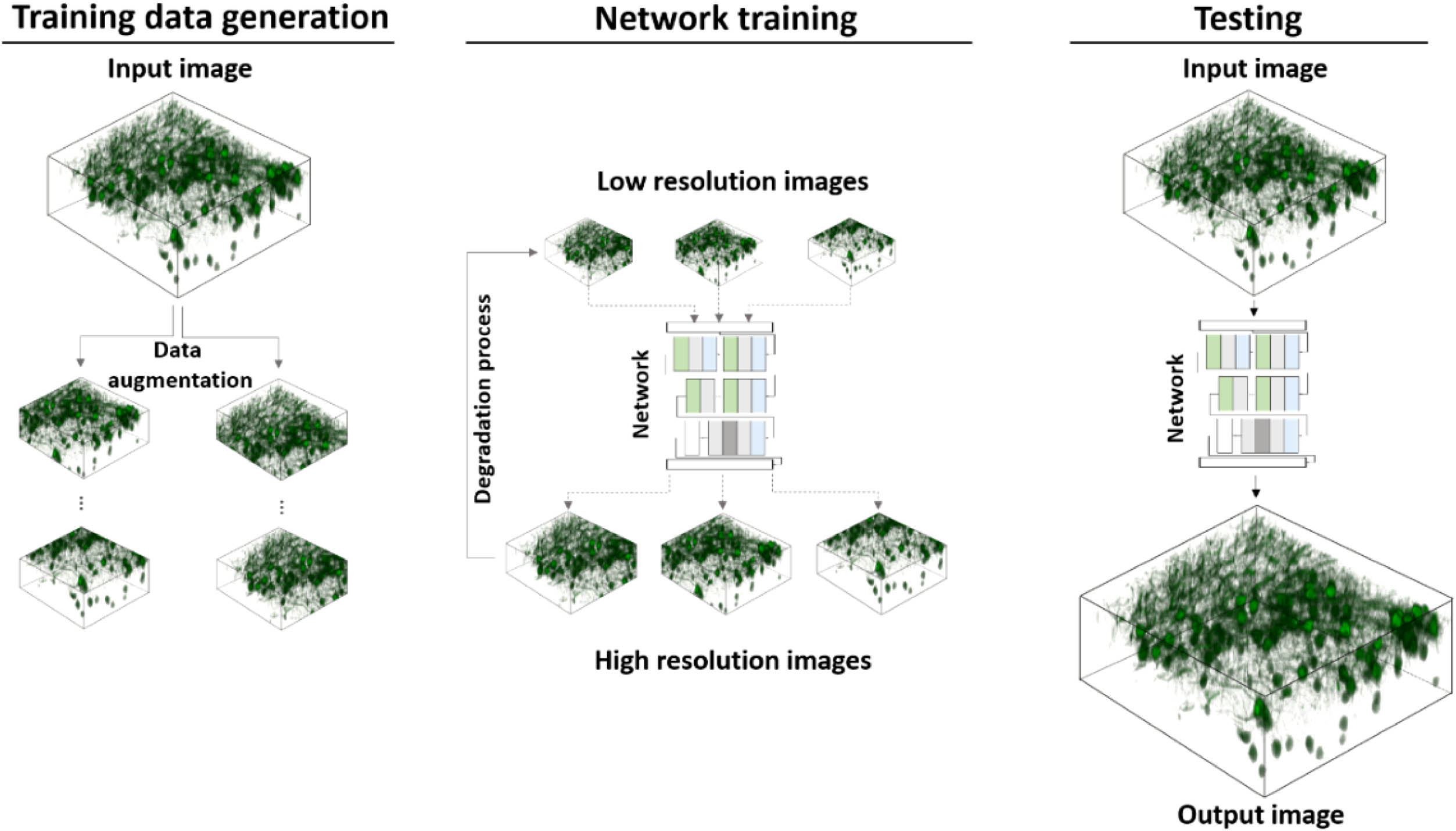
Set citation alerts for the article
Please enter your email address