Xinshan Zhu, Yongjun Qian, Biao Sun, Chao Ren, Ya Sun, Siru Yao. Image Inpainting Forensics Algorithm Based on Deep Neural Network[J]. Acta Optica Sinica, 2018, 38(11): 1110005

Search by keywords or author
- Acta Optica Sinica
- Vol. 38, Issue 11, 1110005 (2018)
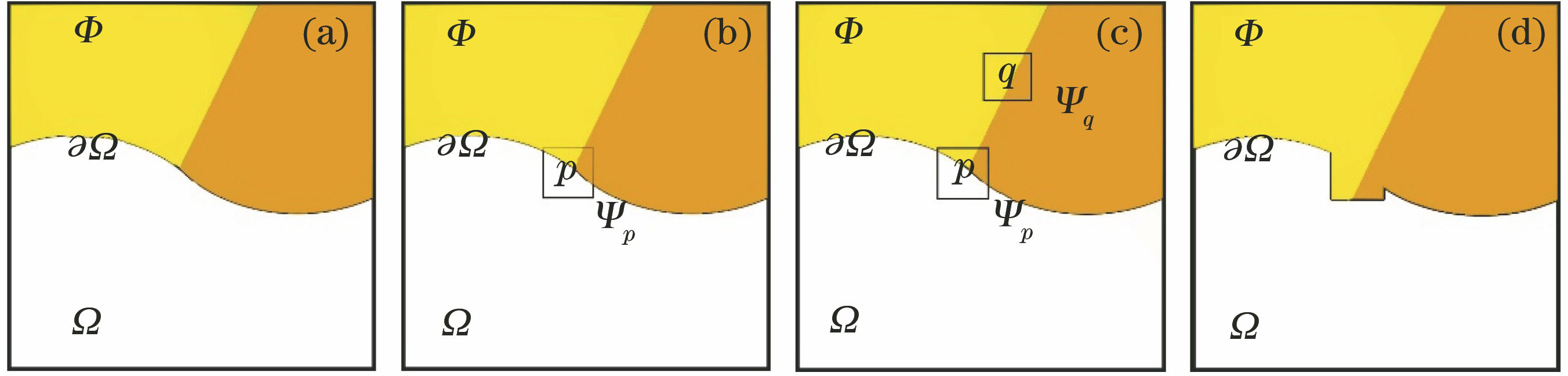
Fig. 1. Image inpainting process by Criminisi algorithm. (a) Determining the inpainting block; (b) determining the matching block; (c) inpainting the image block; (d) renewal the margin
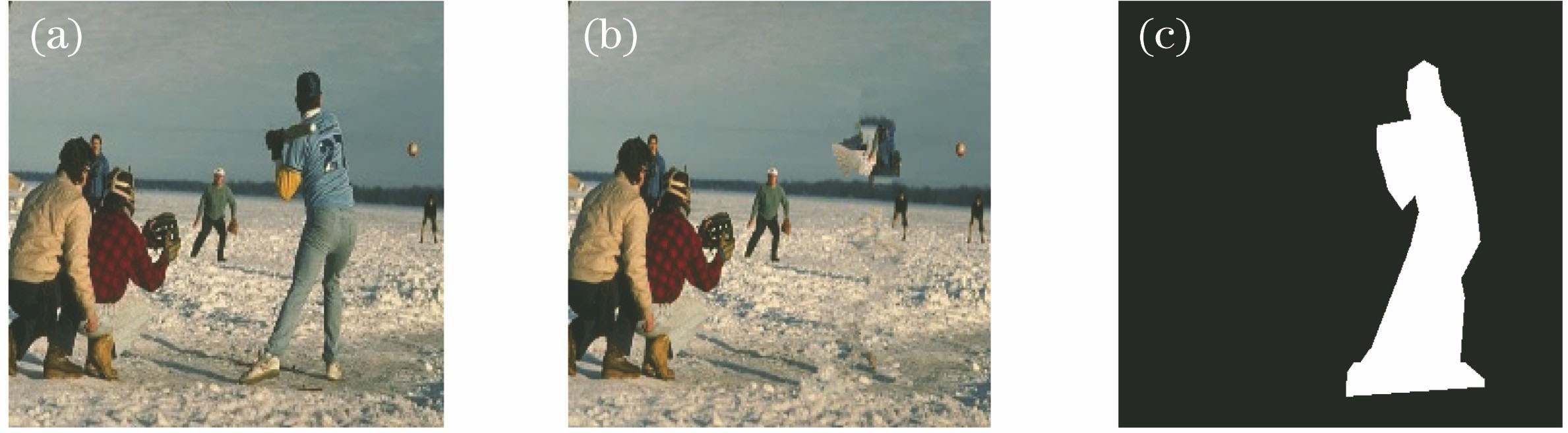
Fig. 2. Image inpainting forensics. (a) Original image; (b) falsified image; (c) image after CNN inpainting forensics
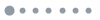
Fig. 3. Structural diagram of network
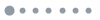
Fig. 4. Structural diagram of network
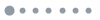
Fig. 5. Partial classical images in testing set
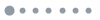
Fig. 6. Falsified images with different area ratios of falsification regions. (a) 5%; (b) 10%; (c) 20%; (d) 0-5%; (e) 10%-30%; (f) 30%-50%
![Detection results of inpainting regions. (a) Original image; (b) mask image; (c) inpainted image; (d) detection results by algorithm in Ref. [14]; (e) detection results by algorithm in Ref. [15]; (f) detection results by proposed method](/Images/icon/loading.gif)
Fig. 7. Detection results of inpainting regions. (a) Original image; (b) mask image; (c) inpainted image; (d) detection results by algorithm in Ref. [14]; (e) detection results by algorithm in Ref. [15]; (f) detection results by proposed method
|
Table 1. Structural parameters of convolution-wide network
|
Table 2. TPR and FPR of classical images by different algorithms (unit: %)
|
Table 3. TPR and FPR of images in UCID dataset by different algorithms (unit: %)
|
Table 4. Detection results of images inpainted by Criminisi algorithm
|
Table 5. Detection results of images inpainted by Shift-map algorithm
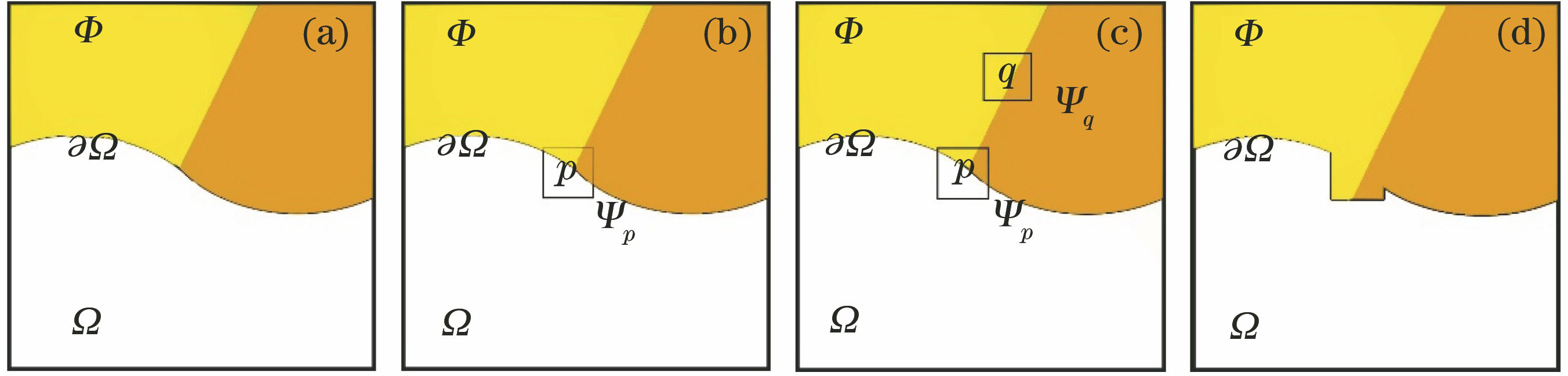
Set citation alerts for the article
Please enter your email address