CHEN Dong-jie, JIANG Pei-hong, GUO Feng-jun, ZHANG Yu-hua, ZHANG Chang-feng. Effects of Prediction Model of Kolar Pear Based on NIR Diffuse Transmission under Different Moving Speed on Online[J]. Spectroscopy and Spectral Analysis, 2020, 40(6): 1839

Search by keywords or author
- Spectroscopy and Spectral Analysis
- Vol. 40, Issue 6, 1839 (2020)
Abstract
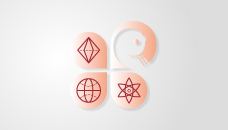
Set citation alerts for the article
Please enter your email address