LIU Rui-min, YIN Yong, YU Hui-chun, YUAN Yun-xia. Extraction of 3D Fluorescence Feature Information Based on Multivariate Statistical Analysis Coupled With Wavelet Packet Energy for Monitoring Quality Change of Cucumber During Storage[J]. Spectroscopy and Spectral Analysis, 2023, 43(9): 2967

Search by keywords or author
- Spectroscopy and Spectral Analysis
- Vol. 43, Issue 9, 2967 (2023)
Abstract
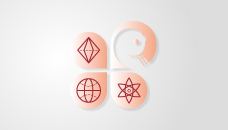
Set citation alerts for the article
Please enter your email address