Ping JIANG, Hao-xiang LU, Zhen-bing LIU. Drugs Identification Using Near-Infrared Spectroscopy Based on Random Forest and CatBoost[J]. Spectroscopy and Spectral Analysis, 2022, 42(7): 2148

Search by keywords or author
- Spectroscopy and Spectral Analysis
- Vol. 42, Issue 7, 2148 (2022)
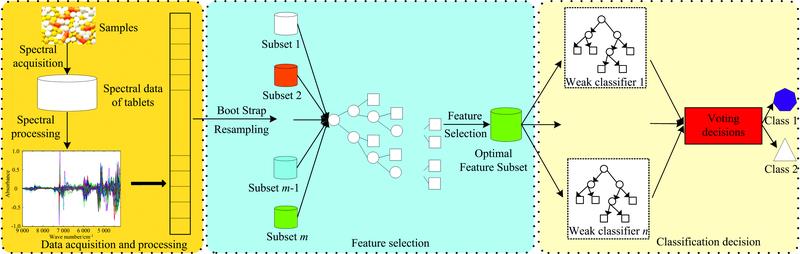
Fig. 1. The structure of RF-CatBoost
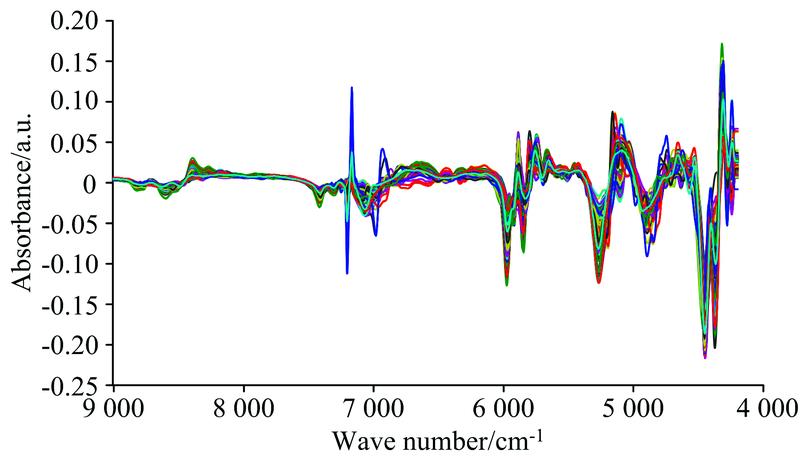
Fig. 2. NIR spectra of pretreated cefixime tablets
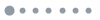
Fig. 3. Covariance matrix of drug NIR data before (a) and after (b) pretreatment
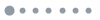
Fig. 4. Classification accuracy of different decision tree numbers in Catboost on datasets of different sizes in group A (a) and group B (b)
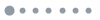
Fig. 5. Standard deviations of each model on different sizes data sets in group A (a) and group B (b)
|
Table 1. Near infrared spectral data of cefixime tablets
|
Table 2. Configuration table of different number of training sets in group A and B
|
Table 3. Classification accuracy of each model on different sizes data sets in group A and B (%)
|
Table 4. Runningtime of each model on different sizes data sets in group A and group B (s)
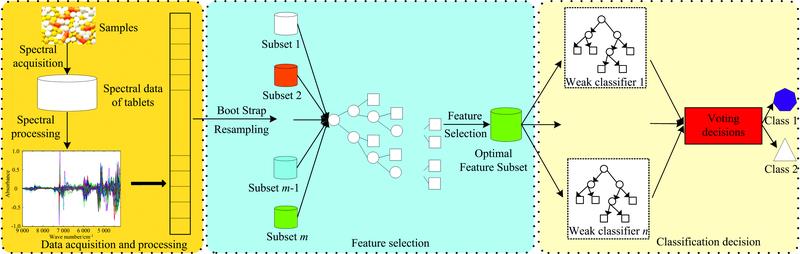
Set citation alerts for the article
Please enter your email address