Ping JIANG, Hao-xiang LU, Zhen-bing LIU. Drugs Identification Using Near-Infrared Spectroscopy Based on Random Forest and CatBoost[J]. Spectroscopy and Spectral Analysis, 2022, 42(7): 2148

Search by keywords or author
- Spectroscopy and Spectral Analysis
- Vol. 42, Issue 7, 2148 (2022)
Abstract
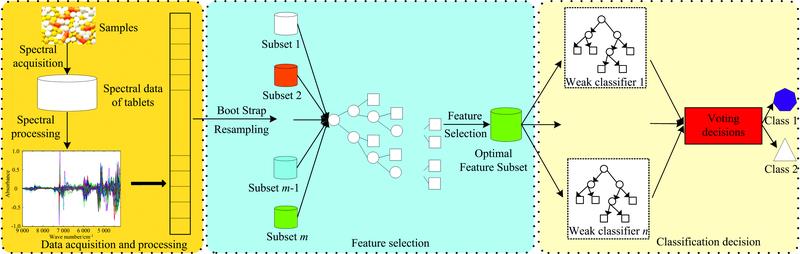
Set citation alerts for the article
Please enter your email address