Mei-feng GAO, Huan-ming TAO. Near Infrared Spectral Wavelength Selection Based on Improved Team Progress Algorithm[J]. Spectroscopy and Spectral Analysis, 2021, 41(10): 3032

Search by keywords or author
- Spectroscopy and Spectral Analysis
- Vol. 41, Issue 10, 3032 (2021)
Abstract
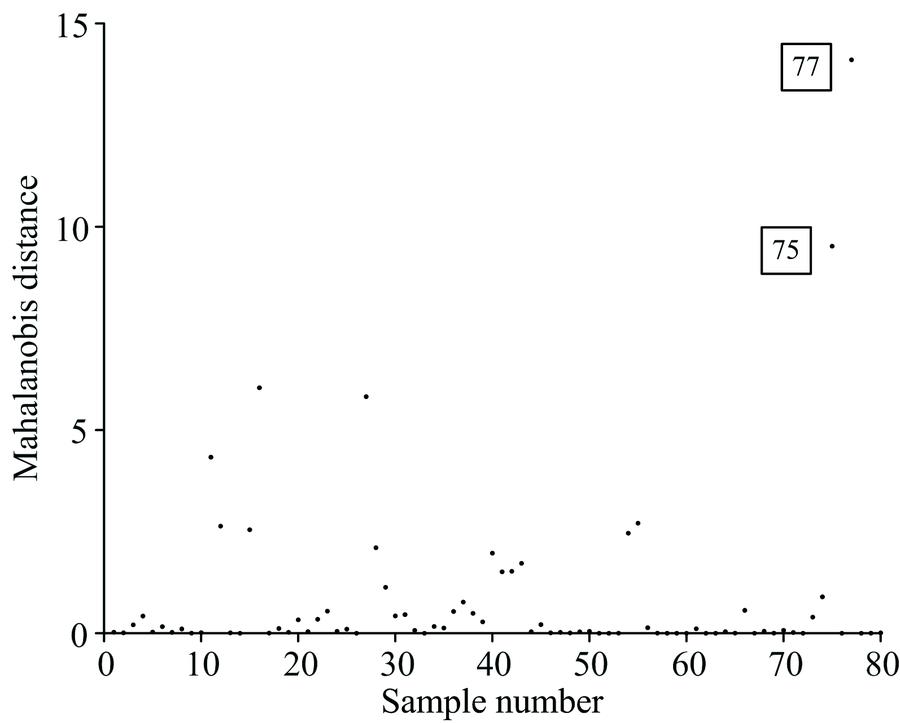
Set citation alerts for the article
Please enter your email address