Yangming Ren, Lingxuan Zhang, Weiqiang Wang, Xinyu Wang, Yufang Lei, Yulong Xue, Xiaochen Sun, Wenfu Zhang, "Genetic-algorithm-based deep neural networks for highly efficient photonic device design," Photonics Res. 9, B247 (2021)

Search by keywords or author
- Photonics Research
- Vol. 9, Issue 6, B247 (2021)
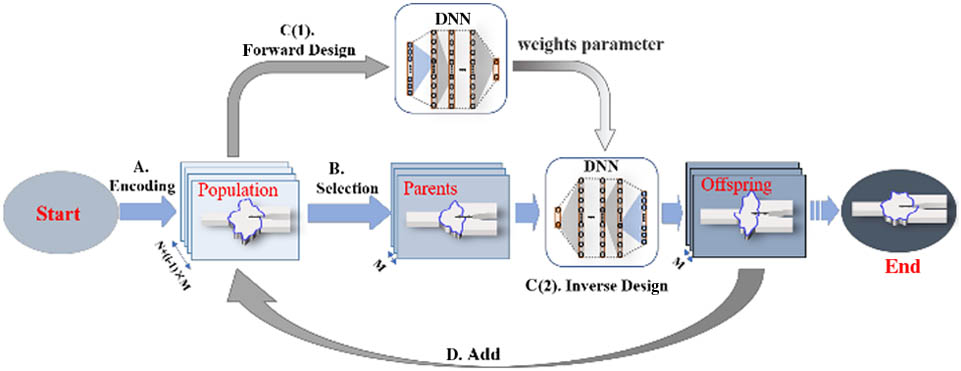
Fig. 1. Workflow of the GDNN algorithm developed in this paper.
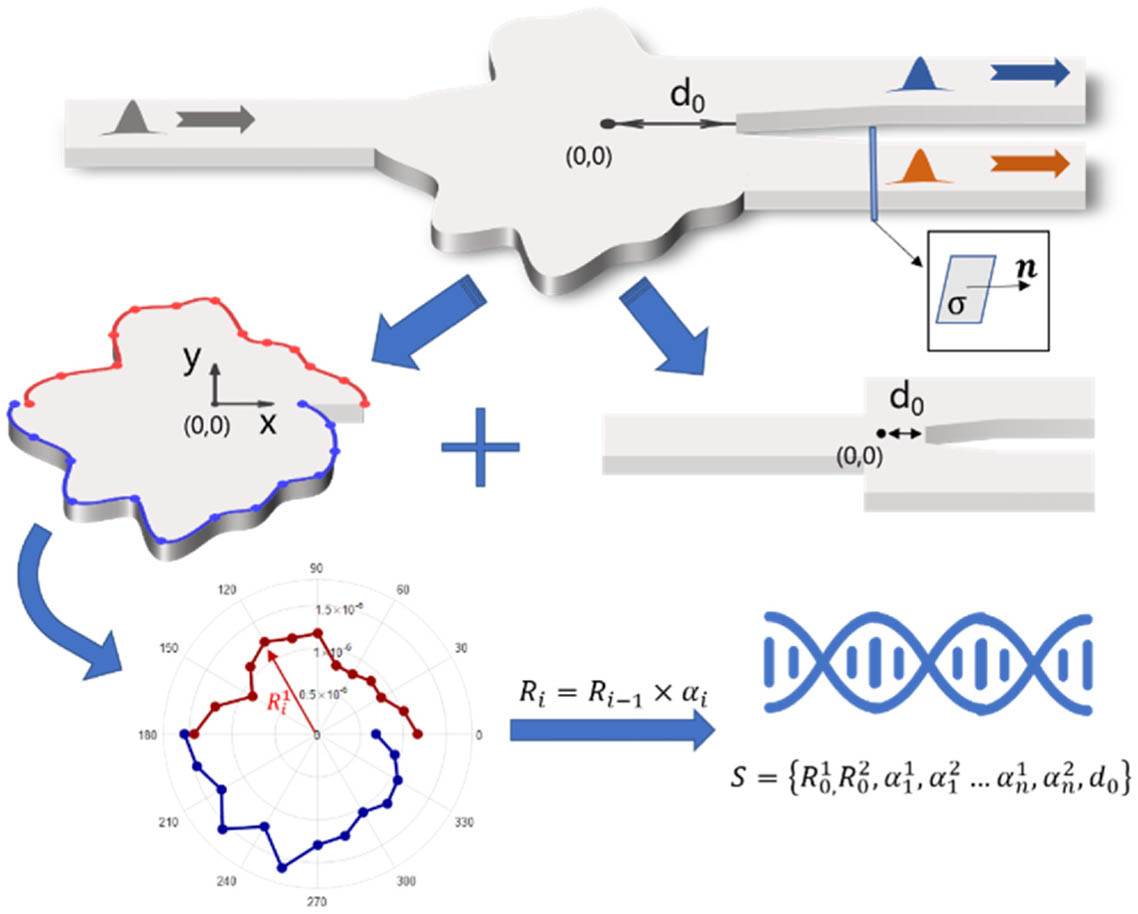
Fig. 2. Encoding process that uses polar vectors and design rule constrains as a parameter vector to describe the design of a given photonic device.
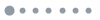
Fig. 3. Schematic drawing of the DNN models of the forward and inverse design processes.
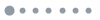
Fig. 4. Design analyses of a power splitter with splitting ratio of 2:3: (a) the evolution of the qualified population proportion; (b) and (c) the FDTD simulation result of the best devices in the initial population and the final population; (d) the distribution of optical transmission of the initial population.
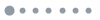
Fig. 5. GDNN design examples with transmission spectrum and FDTD simulation results: (a) a 1:2 power splitter, (b) a 1:1 power splitter, (c) a TE mode converter, and (d) a broadband power splitter.
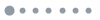
Fig. 6. Comparison of GAN and GDNN design results.
|
Table 1. Training Data Summary of the Designs in This Work
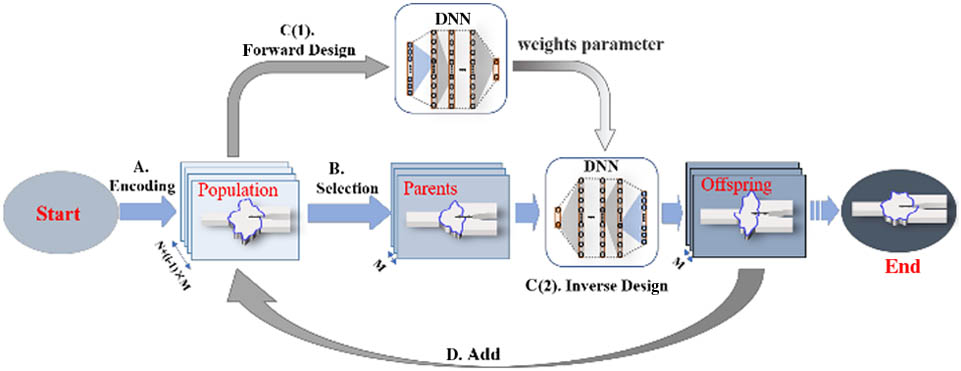
Set citation alerts for the article
Please enter your email address