Qiang Zhang, Ning Jiang, Yiqun Zhang, Anran Li, Huanhuan Xiong, Gang Hu, Yongsheng Cao, Kun Qiu, "On-chip spiking neural networks based on add-drop ring microresonators and electrically reconfigurable phase-change material photonic switches," Photonics Res. 12, 755 (2024)

Search by keywords or author
- Photonics Research
- Vol. 12, Issue 4, 755 (2024)
Abstract
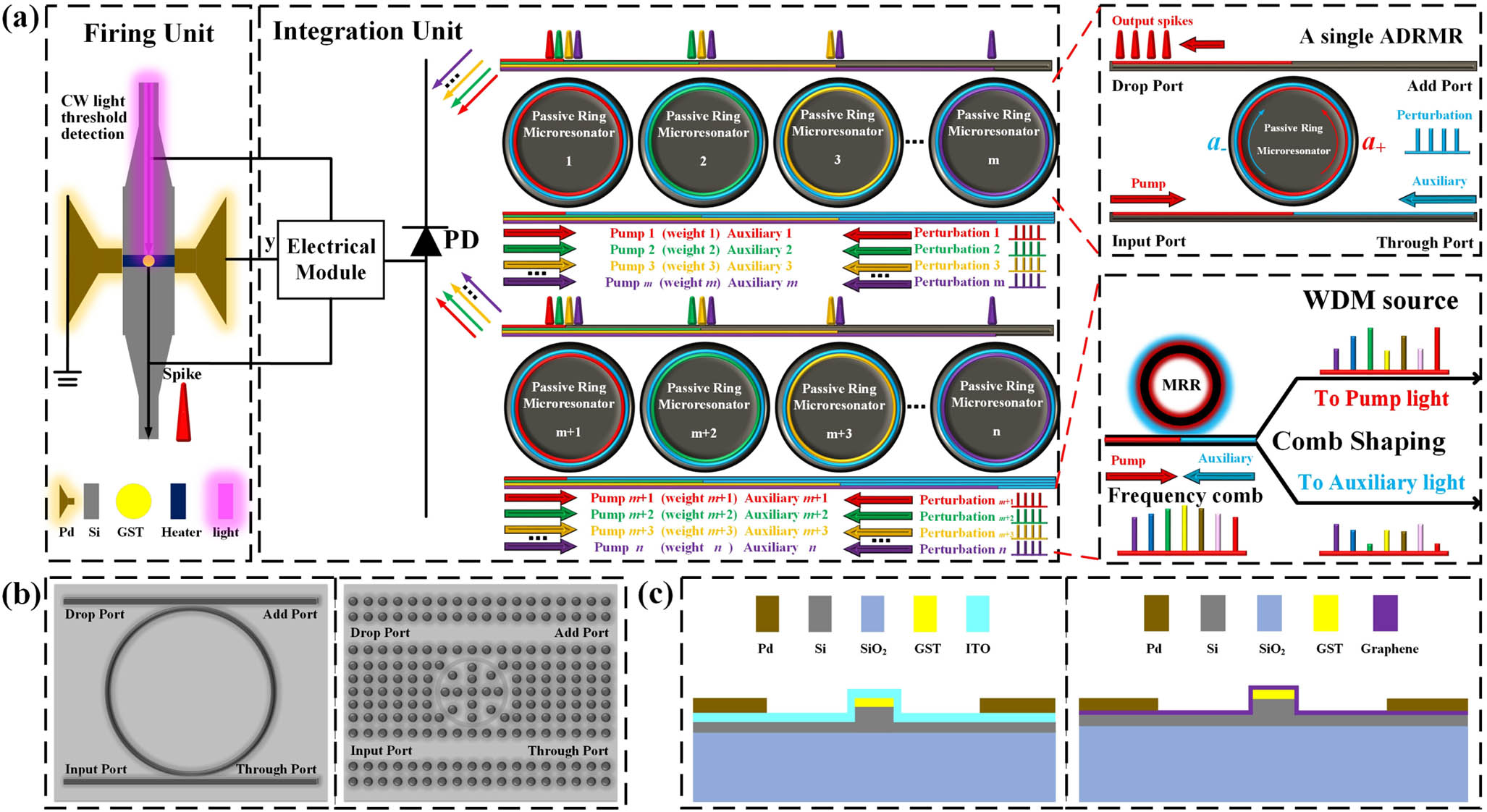
Set citation alerts for the article
Please enter your email address