George Giamougiannis, Apostolos Tsakyridis, Miltiadis Moralis-Pegios, George Mourgias-Alexandris, Angelina R. Totovic, George Dabos, Manos Kirtas, Nikolaos Passalis, Anastasios Tefas, Dimitrios Kalavrouziotis, Dimitris Syrivelis, Paraskevas Bakopoulos, Elad Mentovich, David Lazovsky, Nikos Pleros, "Neuromorphic silicon photonics with 50 GHz tiled matrix multiplication for deep-learning applications," Adv. Photon. 5, 016004 (2023)

Search by keywords or author
- Advanced Photonics
- Vol. 5, Issue 1, 016004 (2023)
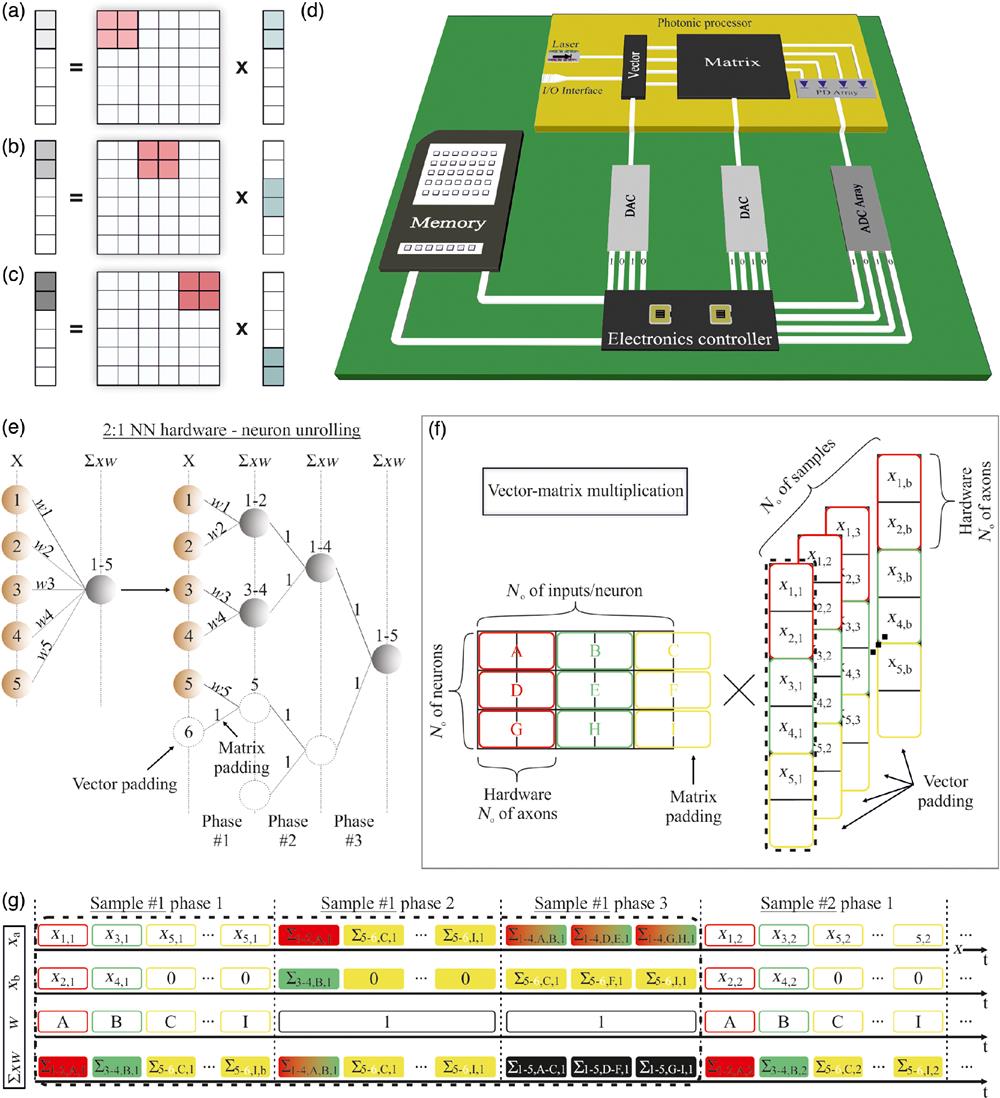
Fig. 1. (a)–(c) The process of tiled matrix multiplication. (d) Electro-optic blocks cointegration for the development of a neuromorphic photonic processor with tiled matrix multiplication capabilities. (e) 2:1 hardware implementing a 5:1 neuron in three phases. (f) The tiled MVM process for a weight matrix with input vectors via 2:1 hardware. (g) The TDM scheme followed during the tiled MVM process of (f).
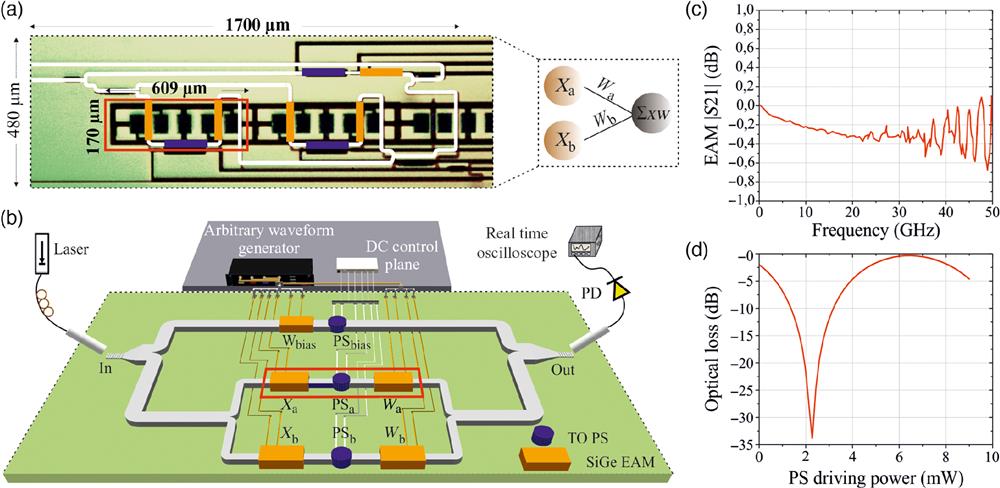
Fig. 2. (a) Microscope top-view photo of the integrated coherent optical linear neuron. The elementary computational cell is encapsulated within a red rectangle. Inset, 2-input neuron realized in the SiPho chip. (b) Experimental setup and visualization of the SiPho chip. (c) Normalized |S21| of the SiGe EAMs deployed in the SiPho processor. (d) Optical loss with respect to the electrical power injected to the .
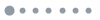
Fig. 3. (a) 6:8:2 NN topology for the classification of benign and malicious traffic. (b)–(g) Sample traces obtained at the output of each inference phase, where black dashed lines represent the software-obtained traces and the orange and blue lines correspond to the experimentally obtained traces at 16 and 50 Gbaud, respectively. (h) MSE representation of the 16 and 50 Gbaud experimentally obtained signals per inference phase.
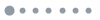
Fig. 4. (a) and (b) Experimentally derived confusion matrices of the RA binary classifier at 16 and 50 Gbaud. (c) Equivalent confusion matrix calculated via the software. (d) Experimentally obtained Cohen’s -score and SNR of the output layer at 16 and 50 Gbaud.
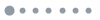
Fig. 5. (a) -input COLN. (b) The photonic Xbar layout realizing the weight matrix (blue rectangle) and utilized as an MVM engine onto an -element input vector (red rectangle). Green rectangle shows the 2:1 MVM architectural part fabricated as a silicon chip with SiGe EAMs for both the input and the weighting stage.
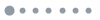
Fig. 6. Square Xbar radix scaling with respect to its IL.
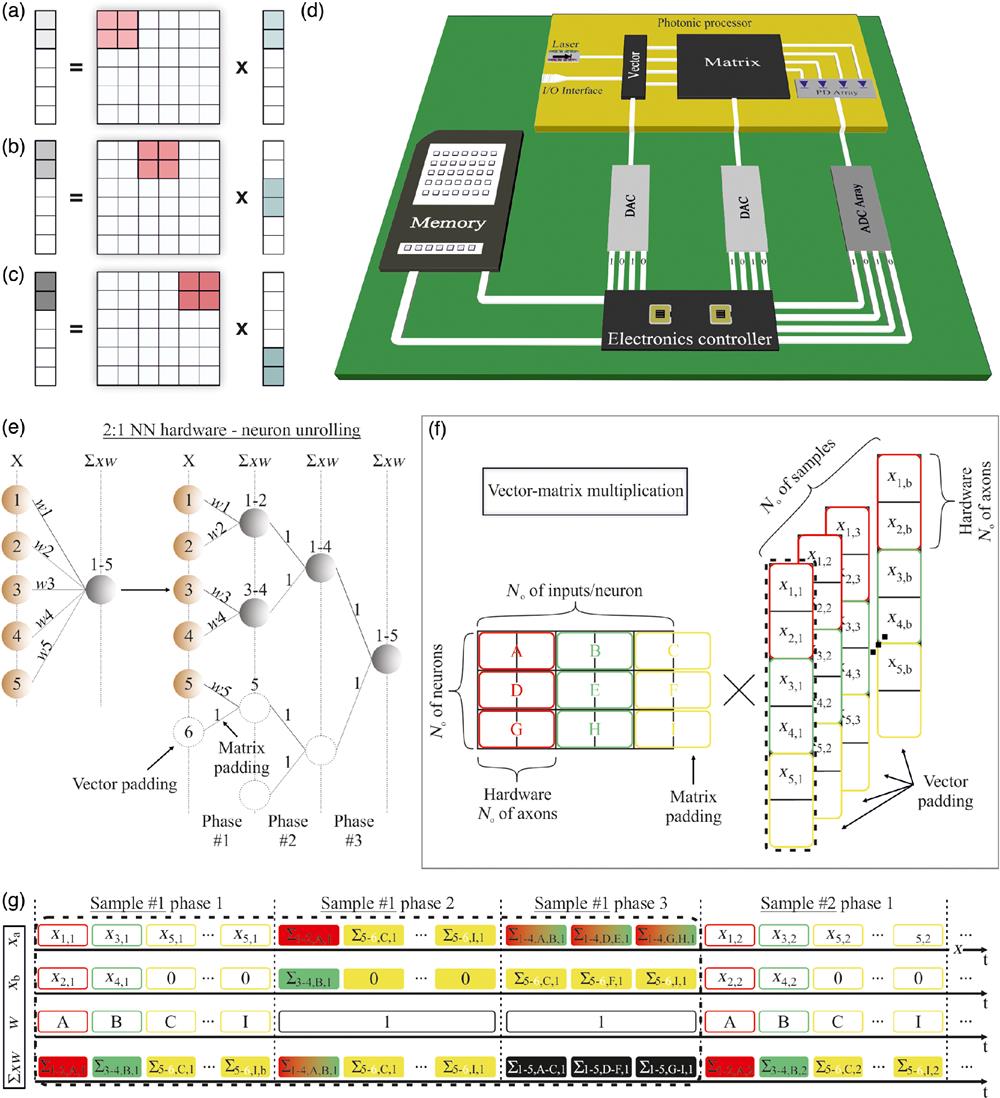
Set citation alerts for the article
Please enter your email address