ZHANG Meng, ZHAO Zhong-gai. Near Infrared Spectral Analysis Modeling Method Based on Deep Belief Network[J]. Spectroscopy and Spectral Analysis, 2020, 40(8): 2512

Search by keywords or author
- Spectroscopy and Spectral Analysis
- Vol. 40, Issue 8, 2512 (2020)
Abstract
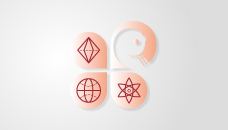
Set citation alerts for the article
Please enter your email address