Rui Sun, Xiaoquan Shan, Qijing Sun, Chunjun Han, Xudong Zhang. NIR-VIS face image translation method with dual contrastive learning framework[J]. Opto-Electronic Engineering, 2022, 49(4): 210317

Search by keywords or author
- Opto-Electronic Engineering
- Vol. 49, Issue 4, 210317 (2022)
Abstract
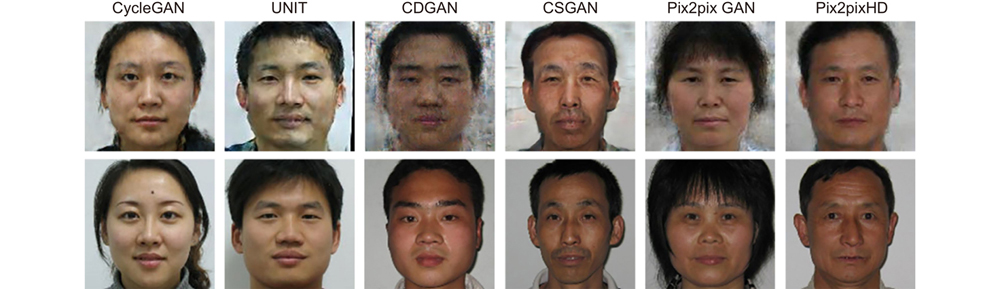
Set citation alerts for the article
Please enter your email address