Bin HU, Hao FU, Wen-bin WANG, Bing ZHANG, Fan TANG, Shan-wei MA, Qiang LU. Research on Deep Sorting Approach Based on Infrared Spectroscopy for High-Value Utilization of Municipal Solid Waste[J]. Spectroscopy and Spectral Analysis, 2022, 42(5): 1353

Search by keywords or author
- Spectroscopy and Spectral Analysis
- Vol. 42, Issue 5, 1353 (2022)
Abstract
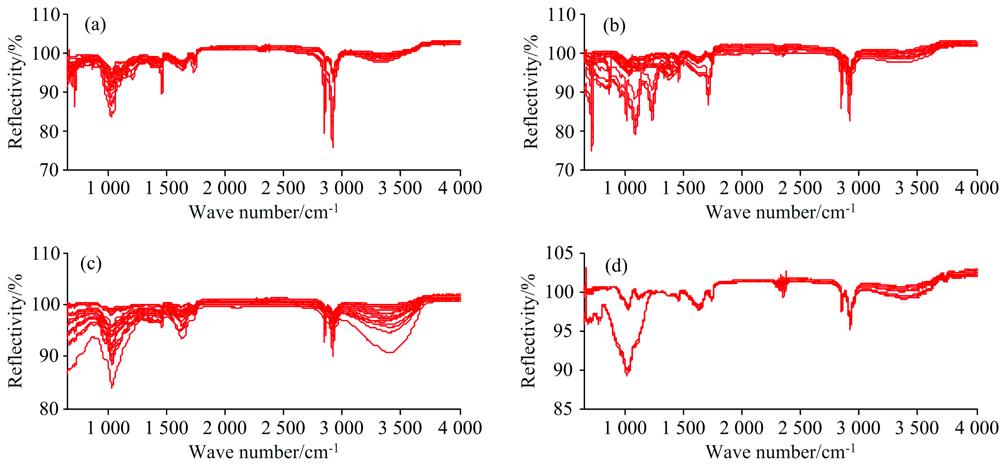
Set citation alerts for the article
Please enter your email address