GAO Shuang, LUAN Xiao-li, LIU Fei. Near Infrared Spectroscopy Process Pattern Fault Detection Based on Mutual Information Entropy[J]. Spectroscopy and Spectral Analysis, 2019, 39(6): 1736

Search by keywords or author
- Spectroscopy and Spectral Analysis
- Vol. 39, Issue 6, 1736 (2019)
Abstract
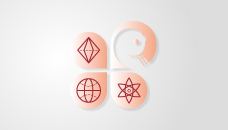
Set citation alerts for the article
Please enter your email address