Shuai-shuai ZHANG, Jun-hua GUO, Hua-dong LIU, Ying-li ZHANG, Xiang-guo XIAO, Hai-feng LIANG. Design of Subwavelength Narrow Band Notch Filter Based on Depth Learning[J]. Spectroscopy and Spectral Analysis, 2022, 42(5): 1393

Search by keywords or author
- Spectroscopy and Spectral Analysis
- Vol. 42, Issue 5, 1393 (2022)
Abstract
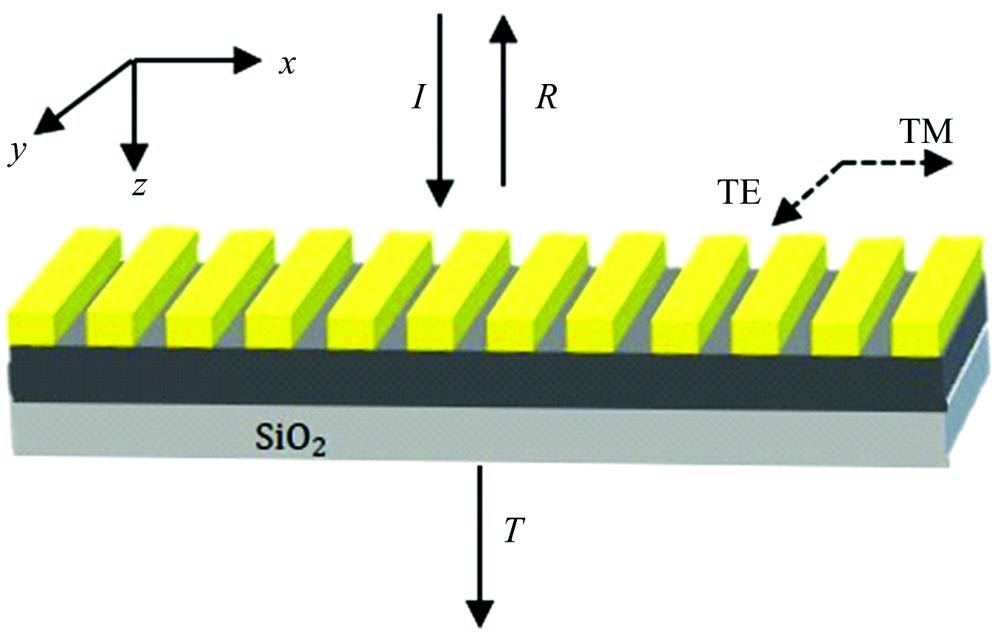
Set citation alerts for the article
Please enter your email address