Yu He, Yunhua Yao, Yilin He, Zhengqi Huang, Dalong Qi, Chonglei Zhang, Xiaoshuai Huang, Kebin Shi, Pengpeng Ding, Chengzhi Jin, Lianzhong Deng, Zhenrong Sun, Xiaocong Yuan, Shian Zhang, "Untrained neural network enhances the resolution of structured illumination microscopy under strong background and noise levels," Adv. Photon. Nexus 2, 046005 (2023)

Search by keywords or author
- Advanced Photonics Nexus
- Vol. 2, Issue 4, 046005 (2023)
Abstract
Keywords
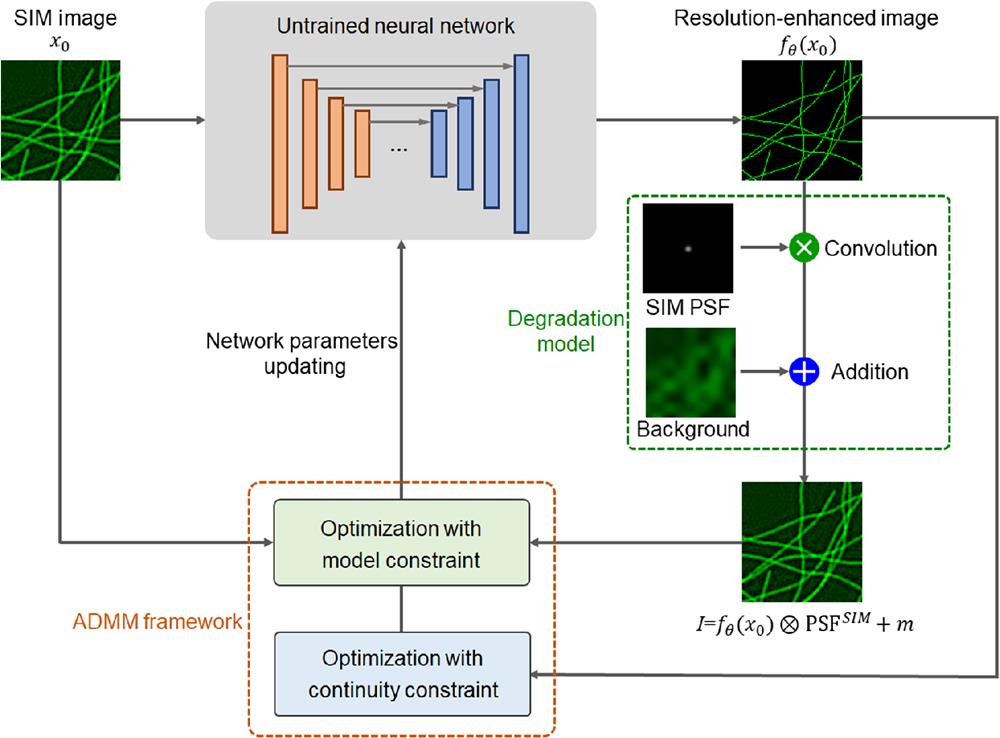
Set citation alerts for the article
Please enter your email address