
- Journal of Geographical Sciences
- Vol. 30, Issue 5, 843 (2020)
Abstract
Keywords
1 Introduction
Earth’s surface is an intersectional study point of geology, hydrology, and meteorology. Geomorphologists have always been taking the most time to pay attention to the full use of Earth surface resources. Along with the development of the knowledge-based economy and sci-tech globalization, numerous approaches have been used to help understanding landform dynamics. Deeper insights into basic principles in turn suggest the interactions of various surface processes that represented by fluvial processes varying with climate processes need to be concentrated more due to the increase of extreme weather events attributed by human-induced global warming (
Some linear/nonlinear bend theories help us understand some unexplored nonlinear aspects of meandering channels for a long time. However, contradictions and limitations are inevitable in the development course of river theory. Driven by seasonal and abnormal events, the state of river channels is continuously changing with the development of hydrologic force, which undoubtedly makes us question the traditional model assumption that whether river could reach a dynamic equilibrium state subjecting to repeated sequence of external disturbances. The frequent occurrence of storms and floods adds to some confusions: how the morphology fluctuations and event characteristics relate to each other; whether it is reasonable to define a singular and dominant discharge to account for unsteady factors that affect the shape of landscape (
Recently, many physicists turned to system theory to study complicated dynamic process with biological and ecological factors to participate in. In particular, transfer function, a single-input single-output filter based on differential equations, has occupied a primary and central place within the fields of system analysis over time. Unlike some simple systems with specific components changing according to deterministic equations, river systems are observed to behave in a non-equilibrium manner with the characteristics of rich irregularity and interactivity. For this reason, scientists developed stochastic models, for example, the deformations of classic Transfer Function Noise models, for enhancing the forecast of river flows generated by rainfall (
Until now, the stochastic response of fluvial relationships is still lack of analysis as complete, accurate and reliable as possible. Different minimization theories and nonlinear dynamic analysis (
2 Stochastic model establishment
2.1 Model description
Classic theory presumed that the rivers are most powerfully shaped by water and sediment inflow at the bankfull stage where the river channel and floodplain become connected. Numerous geomorphologists focused on identifying the bankfull stage and defining a single dominant discharge as the bankfull discharge for river channels in equilibrium. Real-Riverworld experience, however, suggests that this problem was oversimplified and it is doubtful whether the rivers could evolve into a relative steady state. In the Lower Yellow River,
where
where
Then for such channels, we can define a bankfull channel geometry group constituted by bankfull discharge, channel slope, width, depth and velocity in terms of simple power functions:
where
From the general view, Eqs.(3) can be simplified by:
where
After taking the derivative of Eq.(4), we have:
Combining Eq.(5) and Eq.(1), then a deterministic differential equation set with respect to bankfull channel geometries are established:
However, highly organized river systems possess the characteristics of rich regularity and interactivity(
where
The classic SDEs usually use Gaussian white noise to describe the random noise terms. However, the main weakness of such processing is that Gaussian white noise only represents kind of continuous and stable stochastic excitation, it fails to describe the sudden changes of variables. In fact, white noise is equivalent to white light which consists of all different visible spectrums, multiple random noises are also involved in natural noise system. Not only is there Gaussian white noise existing, but also works in non-Gaussian ways. Non-Gaussian shot noise, or Poisson noise, is a typical type of impulse noise which carries some important sharp information and can be modeled by Poisson process. Many stochastic studies (
2.1.1 Single Gaussian white noise model
with discrete solution (
where
Here, we use a CKLS (
2.1.2 Compound Gaussian white noise plus Poisson noise model:
where
where ${{\eta }_{u}}>1$, ${{\eta }_{d}}>0$and \[0\le p=\frac{{{\lambda }^{u}}}{{{\lambda }^{u}}+{{\lambda }^{d}}},q=\frac{{{\lambda }^{d}}}{{{\lambda }^{u}}+{{\lambda }^{d}}}\le 1$.
Here, we use a combined approach to simulate the times (${{\tau }_{1}},{{\tau }_{2}},\ldots $) at which jumps occur, of which times difference ${{R}_{i+1}}={{\tau }_{i+1}}-{{\tau }_{i}}$can be generated from the exponential distribution with mean $1/\lambda $. $\lambda $ is estimated according to historical data (
in which, $
2.1.3 Compound fractional white noise plus Poisson noise model:
where the difference here with Eqs. (10) is that fractional Brownian motion $W_{t}^{{{H}^{[1]}}}$and $W_{t}^{{{H}^{[2]}}} $are a set of zeros-mean Gaussian process with continuous paths and correlation coefficient:
where
It follows that, conditional on the times ${{\tau }_{1}},{{\tau }_{2}},\ldots $ of the jumps, Fractional SDEs-(13) can be discretized using the following improved Euler-Maruyama method:
For satisfying the dual criteria “accuracy and efficiency”, we use a fast one dimensional fBm generator (
2.2 Nonparametric estimate in SDEs
Parameter estimation in SDEs on the basis of short-term discrete-time measurements is an important and difficult task to which a large number of methods exist in the literature in this area. Each of these methods, including MLE method, the generalized method of moments, the simulated moment estimation, and MCMC methods, has particular features that made them valuable. A detailed survey and comparison by
Let $\left\{ {{x}_{i}},i=0,1,\cdots N \right\}$be the available real data;
The detailed estimation procedure of
(1) Let $\left\{ {{F}_{t}}:t\in [0,\ T] \right\}$ denotes the time range. Sampling interval is fixed as$\Delta \text{=}\frac{T}{N}$. Consider the time interval [
(2) Based on the sample matrix$\{X_{{{t}_{i}}}^{r}\}$, in the first case, a nonparametric kernel-function can be constructed to estimate the transition density function of the diffusion processes driven by simple Wiener process:
where the kernel function $K(\cdot ) $is given by the normal kernel:
with bandwidth
where
While for the jump-diffusion processes in the second case, defining $l_{i}^{r}=\ln (X_{i}^{r})-$ $\ln ({{x}_{i-1}})$(
where
Then the optimum result is just the needed maximum likelihood estimate of
3 Case study
3.1 Data
In recent decades, the worldwide frequency of extreme weather events attributed by global warming has brought serious disasters to the international society, and the study predicted the threat will continue to increase in the future. Meanwhile, the Yellow River has always been suffering from frequent devastating floods, droughts, and the consequent channel changes. The Lower Yellow River (LYR) has a length of 786 km and an average slope of 0.12‰ measured from the Taohuayu Valley to the estuary of the Yellow River. In general, this region is divided into four sub-reaches: the braided Huayuankou-Gaocun reach, the transitional Gaocun-Sunkou reach, Sunkou-Aishan reach, and the meandering Aishan-Lijin reach. Hyper-concentrated flows frequently occur in the Lower Yellow River due to the serious soil erosion on the Loess Plateau. Many dams were constructed along the mainstream and have aroused the drastic changes in river regime over time. Particularly zero flow conditions (
Figure 1.The Gaocun-Sunkou reach of the Lower Yellow River
Year | Flood season’s average value (*) | Bankfull discharge | Slope | Width | Depth | Velocity | ||
---|---|---|---|---|---|---|---|---|
Discharge | IS coefficient | |||||||
1952 | 2417.073 | 0.0080 | 6700 | 0.120 | 750 | 1.48 | 1.57 | |
1953 | 2562.967 | 0.0153 | 5800 | 0.112 | 487.5 | 2.58 | 1.25 | |
1954 | 3531.862 | 0.0132 | 5500 | 0.104 | 450 | 3.17 | 1.13 | |
1955 | 3218.593 | 0.0095 | 5400 | 0.142 | 711.5 | 1.54 | 1.57 | |
1956 | 2673.740 | 0.0154 | 5420 | 0.121 | 652 | 2.19 | 0.96 | |
1957 | 1838.984 | 0.0197 | 5300 | 0.125 | 620.8 | 2.20 | 0.95 | |
1958 | 4190.626 | 0.0123 | 5500 | 0.124 | 719.5 | 1.32 | 1.07 | |
1959 | 2070.854 | 0.0356 | 6700 | 0.126 | 684 | 1.10 | 1.47 | |
1960 | 1092.754 | 0.0343 | 6500 | 0.121 | 325.5 | 1.02 | 0.90 | |
1961 | 2729.593 | 0.0061 | 7200 | 0.103 | 445 | 2.37 | 1.84 | |
1962 | 2232.033 | 0.0079 | 7800 | 0.114 | 581 | 1.78 | 1.20 | |
1963 | 2926.106 | 0.0065 | 8500 | 0.110 | 584 | 1.30 | 1.00 | |
1964 | 4969.268 | 0.0052 | 9500 | 0.113 | 1217.5 | 1.69 | 1.62 | |
1965 | 1534.878 | 0.0123 | 9800 | 0.128 | 614 | 1.24 | 1.69 | |
1966 | 2898.642 | 0.0176 | 8500 | 0.139 | 967 | 1.24 | 1.34 | |
1967 | 4232.683 | 0.0091 | 6000 | 0.125 | 957.5 | 1.80 | 1.80 | |
1968 | 3114.821 | 0.0110 | 6000 | 0.126 | 1618.8 | 1.16 | 1.63 | |
1969 | 1155.35 | 0.0378 | 5000 | 0.117 | 390 | 1.87 | 1.54 | |
1970 | 1675.976 | 0.0359 | 4300 | 0.124 | 1067 | 1.35 | 1.28 | |
1971 | 1385.22 | 0.0336 | 4300 | 0.123 | 922.5 | 1.21 | 1.36 | |
1972 | 1205.667 | 0.0223 | 3900 | 0.121 | 493.5 | 0.83 | 0.92 | |
1973 | 1938.841 | 0.0282 | 3500 | 0.121 | 566.6 | 1.09 | 0.47 | |
1974 | 1164.151 | 0.0271 | 3370 | 0.121 | 610.5 | 1.22 | 1.76 | |
1975 | 3035.675 | 0.0115 | 4710 | 0.118 | 553.5 | 1.68 | 1.31 | |
1976 | 3137.325 | 0.0088 | 6090 | 0.117 | 542 | 1.47 | 1.59 | |
1977 | 1627.472 | 0.0419 | 6500 | 0.121 | 366.1 | 1.32 | 1.15 | |
1978 | 1949.756 | 0.0262 | 5500 | 0.113 | 404.5 | 2.23 | 1.12 | |
1979 | 1945.122 | 0.0199 | 5200 | 0.108 | 312.1 | 3.09 | 1.38 | |
1980 | 1183.447 | 0.0225 | 4500 | 0.120 | 483.5 | 1.30 | 1.61 | |
1981 | 3011.618 | 0.0114 | 3900 | 0.114 | 412.7 | 2.25 | 1.39 | |
1982 | 2226.911 | 0.0094 | 5900 | 0.123 | 538 | 1.53 | 1.64 | |
1983 | 3310.407 | 0.0063 | 7300 | 0.117 | 583.7 | 2.27 | 1.58 | |
1984 | 3127.667 | 0.0070 | 7400 | 0.118 | 551.5 | 1.66 | 1.68 | |
1985 | 2298.691 | 0.0110 | 7600 | 0.113 | 527.5 | 2.92 | 1.54 | |
1986 | 1207.065 | 0.0138 | 7400 | 0.115 | 529.5 | 1.90 | 0.57 | |
1987 | 694.1382 | 0.0216 | 6800 | 0.114 | 233.1 | 1.78 | 0.89 | |
1988 | 1915.215 | 0.0244 | 6400 | 0.110 | 353.5 | 2.58 | 1.53 | |
1989 | 1796.545 | 0.0156 | 4600 | 0.111 | 376.5 | 2.15 | 1.49 | |
1990 | 1233.398 | 0.0217 | 4500 | 0.111 | 363.2 | 2.31 | 1.39 | |
1991 | 429.735 | 0.0523 | 4400 | 0.121 | 453.5 | 1.24 | 0.90 | |
1992 | 1168.78 | 0.0383 | 3200 | 0.121 | 465 | 1.03 | 1.07 | |
1993 | 1285.764 | 0.0207 | 3600 | 0.115 | 460 | 1.18 | 1.25 | |
1994 | 1221.439 | 0.0394 | 3700 | 0.119 | 469 | 1.20 | 1.22 | |
1995 | 1013.087 | 0.0465 | 3000 | 0.122 | 486 | 0.96 | 1.09 | |
1996 | 1357.556 | 0.0232 | 2800 | 0.119 | 537.5 | 1.27 | 1.48 | |
1997 | 299.5125 | 0.1589 | 2750 | 0.126 | 410.5 | 1.10 | 0.94 | |
1998 | 899 | 0.0366 | 2700 | 0.122 | 398 | 0.95 | 1.10 | |
1999 | 779.2927 | 0.0468 | 2800 | 0.121 | 474 | 1.07 | 1.13 | |
2000 | 443.1463 | 0.0161 | 2600 | 0.121 | 500.5 | 1.06 | 0.91 | |
2001 | 321.3228 | 0.0232 | 2400 | 0.121 | 486 | 1.01 | 1.26 | |
2002 | 714.4472 | 0.0148 | 2000 | 0.120 | 446 | 1.08 | 1.20 | |
2003 | 1300.414 | 0.0113 | 2300 | 0.118 | 448.5 | 1.26 | 0.84 | |
2004 | 818.2926 | 0.0239 | 3600 | 0.115 | 439 | 1.34 | 0.90 | |
2005 | 897.536 | 0.0104 | 4000 | 0.115 | 528 | 1.38 | 1.17 | |
2006 | 806.008 | 0.0079 | 4500 | 0.115 | 431.5 | 1.33 | 1.24 | |
2007 | 1140.674 | 0.0059 | 4700 | 0.112 | 491 | 1.62 | 1.24 | |
2008 | 625.040 | 0.0095 | 4800 | 0.113 | 347.5 | 1.55 | 1.40 | |
2009 | 646.455 | 0.0041 | 5000 | 0.116 | 515 | 1.21 | 1.03 | |
2010 | 1166.422 | 0.0045 | 5300 | 0.118 | 518.5 | 1.05 | 0.99 | |
2011 | 934.065 | 0.0051 | 5400 | 0.119 | 668 | 1.23 | 1.04 | |
2012 | 1438.495 | 0.0057 | 5400 | 0.120 | 648.5 | 1.24 | 1.04 | |
2013 | 1219.894 | 0.0076 | 5800 | 0.118 | 533.5 | 1.27 | 1.10 |
Table 1.
Flood season’s average discharge, suspended sediment concentration, and annual measured bankfull channel geometries along the Gaocun station downwards
3.2 Parameter estimate
We estimate the unknown parameters set of stochastic discharge in the first place, and then deal with the ones of stochastic hydraulic geometries. For validating the SDEs, time range is assigned to be [1952, 2013], time interval is 1, iterative step is arranged as 1/12. A problem occurred in the application of the nonparametric estimate procedure that initial value could significantly affect the optimization result due to the use of “fmincon” function. Then our solution out of many attempts is to perform multiple calculations; every single process is initialized with the average of the previous results, thus greatly reducing the importance of initial value. After each calculation at 5,000 runs with 1,000 computer-generated sample datasets, the parameter estimation results are well obtained (Tables 2-7). Additionally, we also evaluate the deterministic Eqs.(6) with a least square fit as the control groups for ease of comparative study.
Estimate | K | b | c | $ \beta$ | ${{\sigma }_{1}}$ | $\gamma $ |
---|---|---|---|---|---|---|
Mean | 76.495 | -0.477 | 0.299 | 0.213 | 0.136 | 0.582 |
SD | 23.833 | 0.044 | 0.027 | 0.018 | 0.005 | 0.047 |
Table 2.
The estimated results of the unknown parameters set for the SDEs-Eq.(8a)
Estimate | Slope ( | Width ( | Depth ( | Velocity ( | |
---|---|---|---|---|---|
m | Mean | 0.184 | 0.771 | 0.560 | 0.424 |
SD | 0.038 | 0.218 | 0.092 | 0.068 | |
${{\sigma }_{2}}$ | Mean | 0.073 | 0.143 | 0.276 | 0.130 |
SD | 0.148 | 0.047 | 0.069 | 0.023 |
Table 3.
The estimated results of the unknown parameters set for the SDEs-Eq.(8b)
Estimate | b | c | $\beta $ | ${{\sigma }_{1}}$ | $\gamma $ | $\lambda _{u}^{[1]} $ | $\lambda _{d}^{[1]} $ | $1/\eta _{u}^{\left[ 1 \right]} $ | $1/\eta _{d}^{[1]} $ | |
---|---|---|---|---|---|---|---|---|---|---|
Mean | 23.818 | -0.505 | 0.432 | 0.312 | 0.118 | 0.494 | 0.030 | 0.020 | 0.215 | 0.573 |
SD | 4.795 | 0.025 | 0.018 | 0.002 | 0.003 | 0.003 | 0.001 | 0.004 | 0.016 | 0.047 |
Table 4.
The estimated results of the unknown parameters set for the jump-diffusion Eq. (10a)
Estimate | Slope ( | Width ( | Depth ( | Velocity ( | |
---|---|---|---|---|---|
Mean | -0.086 | 0.264 | 0.350 | 0.310 | |
SD | 0.009 | 0.043 | 0.059 | 0.046 | |
${{\sigma }_{2}}$ | Mean | 0.075 | 0.301 | 0.300 | 0.160 |
SD | 0.014 | 0.065 | 0.065 | 0.055 | |
$\lambda _{u}^{[2]} $ | Mean | 0.180 | 0.300 | 0.311 | 0.394 |
SD | 0.001 | 0.014 | 0.029 | 0.065 | |
$\lambda _{d}^{[2]} $ | Mean | 0.180 | 0.300 | 0.327 | 0.426 |
SD | 0.004 | 0.026 | 0.054 | 0.025 | |
$1/\eta _{u}^{[2]} $ | Mean | 0.059 | 0.079 | 0.180 | 0.080 |
SD | 0.001 | 0.045 | 0.025 | 0.004 | |
$1/\eta _{d}^{[2]} $ | Mean | 0.030 | 0.109 | 0.175 | 0.177 |
SD | 0.009 | 0.068 | 0.027 | 0.062 |
Table 5.
The estimated results of the unknown parameters set for the jump-diffusion Eq. (10b)
Estimate | K | b | c | $\beta $ | ${{\sigma }_{1}}$ | $\gamma $ | ${{H}^{[1]}} $ | $\lambda _{u}^{[1]} $ | $\lambda _{d}^{[1]} $ | $1/\eta _{u}^{\left[ 1 \right]} $ | $1/\eta _{d}^{[1]} $ |
---|---|---|---|---|---|---|---|---|---|---|---|
Mean | 25.14 | -0.52 | 0.42 | 0.29 | 0.11 | 0.23 | 0.55 | 0.09 | 0.06 | 0.04 | 0.15 |
SD | 3.27 | 0.03 | 0.02 | 0.01 | 0.00 | 0.00 | 0.00 | 0.00 | 0.02 | 0.00 | 0.01 |
Table 6.
The estimated results of the unknown parameters set for the fractional jump-diffusion Eq. (13a)
Estimate | Slope ( | Width ( | Depth ( | Velocity ( | |
---|---|---|---|---|---|
Mean | -0.141 | 0.704 | 0.350 | 0.880 | |
SD | 0.011 | 0.043 | 0.026 | 0.063 | |
${{\sigma }_{2}}$ | Mean | 0.090 | 0.500 | 0.640 | 0.260 |
SD | 0.015 | 0.035 | 0.055 | 0.017 | |
${{H}^{[2]}} $ | Mean | 0.471 | 0.349 | 0.471 | 0.301 |
SD | 0.054 | 0.013 | 0.026 | 0.063 | |
$\lambda _{u}^{[2]} $ | Mean | 0.374 | 0.410 | 0.361 | 0.554 |
SD | 0.072 | 0.075 | 0.026 | 0.064 | |
$\lambda _{d}^{[2]} $ | Mean | 0.380 | 0.410 | 0.367 | 0.556 |
SD | 0.095 | 0.075 | 0.023 | 0.052 | |
$1/\eta _{u}^{[2]} $ | Mean | 0.088 | 0.488 | 0.300 | 0.230 |
SD | 0.041 | 0.048 | 0.011 | 0.033 | |
$1/\eta _{d}^{[2]} $ | Mean | 0.087 | 0.453 | 0.105 | 0.170 |
SD | 0.025 | 0.064 | 0.023 | 0.028 |
Table 7.
The estimated results of the unknown parameters set for the fractional jump-diffusion Eq. (13b)
3.3 Result
With the estimated parameters, the dynamic probabilities of bankfull discharge and hydraulic geometries can be approximated by repeated computations (over 10,000 runs) on the SDEs. For the jump-diffusion cases, based on maximizing the relevance of stochastic average (the mean value of the computation results for every year) and measurements from a given stimulus intensity, the optimal jump moments can be estimated (Figures 2-4). The following reveals the calculated probability evolution results.
3.3.1 Model-1
It can be seen from
Figure 2.Comparison of the calculated and measured values of bankfull channel geometries in the Gaocun-Sunkou reach of the Lower Yellow River under the Model-1 condition
3.3.2 Model-2
Jump-diffusion model was first introduced by
Figure 3.Comparison of the calculated and measured values of bankfull channel geometries in the Gaocun-Sunkou reach of the Lower Yellow River under the Model-2 condition
3.3.3 Model-3
The research of fBm can be traced back to the early 1940s.
Figure 4.Comparison of the calculated and measured values of bankfull channel geometries in the Gaocun-Sunkou reach of the Lower Yellow River under the Model-3 condition
4 Discussion
4.1 Model comparison
Through the consistent procedure running based on SDEs, the variation of bankfull channel geometries are demonstrated to be characterized by nonlinearity, non-stationary, and abruptness. Here deterministic equilibrium curves are converted into probabilistic distribution zone, in which the significant interval and the average trend could greatly determine the research value for river management. The implementation of SDEs provides such kind of important supplement that we move on to conduct a comparable study of the different models in a quantitative way. Obviously, in case that exterior integral styles permitted, it will be satisfying to choose a model with the larger of effective probability (the average occurring probability of discrete measurements in the distributions) and the smaller of effective probability stability thickness (the interior limit range), and also the smaller of the relative error between stochastic average and measurements. Considering the great significance of the above factors for the investigation and comparison, we describe below their time-varying processes (Figures 5-6), and in consistent with Figures 2-4, we give targeted suggestions for each hydraulic geometry relation in the following parts.
Figure 5.The time-varying process of effective probabilistic stability thickness of hydraulic geometry in the Gaocun-Sunkou reach of the Lower Yellow River under the three model conditions
(1) Slope. Figures (2-4)b show that jump-diffusion models could strongly help to improve the capacity for controlling systemic slope changes, and simple Gaussian white noise model should undoubtedly be abandoned. Meanwhile judging by Figures (5-6)a, victory belongs to fractional model due to its overall advantage in effective stability thickness and effective probability, though the Model-2 enjoys a bit advantage over relative error of dataset.
Figure 6.Comparison of stochastic average with measurements in the Gaocun-Sunkou reach of the Lower Yellow River under the three model conditions
(2) Width. Similar to the slope case, according to Figures (2-4)c, intuitively, we can find the significant improvement of jump-diffusion models over simple white noise model. The fractional approach has the advantage of having little relative error, and better performance in simulating the fluctuation of stochastic average.
(3) Depth. Different to the above cases, Figures (2-4)d show the slight rising effect of fractional term in describing the dynamic changes on the basis of Poisson jump. According to the performances in the effective probabilistic zone and relative data error in Figures (5-6)c, it seems Poisson jump could take effect well only under the linear Gaussian circumstances. In other words, dynamic depth can thus be proven to be highly continuous, stable, and be affected linearly by unexpected stochastic events.
(4) Velocity. In this case, Figures (2-4)e and Figures (5-6)d show that fractional SDEs is superior to Poisson jump model, especially in the fluctuation of the simulation results, effective probability properties and relative error (
In short, fractional jump-diffusion model has best applicability in simulating the slope, width, and velocity changes, the simple jump-diffusion model is good enough for exploring depth.
4.2 Systematic application
In a certain sense river is alive, like a man, bankfull channel geometries constitute the primary organ of river body. Fundamentally, discharge plays the role of drivers and sustainers of diversified river landscape. And in many cases, there are a number of reasons to explain the nonlinearity of hydraulic geometry relations, such as the presence of gravel bars, the variability of hydraulic geometry of riffles, or an abrupt change in the resistance to flow (
Figure 7.The time-varying probability distribution of riverbed stability indices, hydraulic width/depth ratio and stream power in the Gaocun-Sunkou reach of the Lower Yellow River based on Fractional Jump-Diffusion model (13)
It can be seen from
Correlation | $\zeta $ | $\Omega $ | ||
---|---|---|---|---|
0.035 | 0.140 | 0.523 | 1 |
Table 8.
The correlation coefficients of time-varying stochastic average Zw, $\zeta $,$\Omega $ with Q
5 Conclusion
This paper considered the bankfull channel geometry problem for a stochastic time-varying state space of multi-input multi-output river system by involving random noise factors in the stochastic differential equations. Three different models that combined the Gaussian white noise, Fractional white noise, Poisson noise were evaluated respectively in the Gaocun-Sunkou reach of the Lower Yellow River to investigate the evolution of probability distribution of bankfull channel geometries here over time. Results show that this procedure effectively extends the traditional deterministic equations into the controllable probabilistic stability forms, successfully improves the understanding of systemic river evolution under limited data conditions, and, contributes much to river management and the utilization of the earth’s surface resources. Future research will continue to receive new authentication and become more perfect by avoiding the use of over-simplified noise terms.
References
[1] Handbook of Financial Econometrics: Tools and Techniques. Elsevier(2009).
[2] Rate of floodplain reworking in response to increasing storm-induced floods, Squamish River, south-western British Columbia, Canada. Earth Surface Processes & Landforms, 36, 872-884(2011).
[4] A nonlinear model for river meandering. Water Resources Research, 45, 546-550(2009).
[5] Approximation of jump diffusions in finance and economics. Research Paper, 29, 283-312(2006).
[6] Convergence and stability analysis for implicit simulations of stochastic differential equations with random jump magnitudes. Discrete and Continuous Dynamical Systems: Series B (DCDS-B), 9, 47-64(2017).
[8] Stochastic analysis of the Fractional Brownian Motion. Potential Analysis, 10, 177-214(1999).
[9] Study on particularity of hump reach evolution in Lower Yellow River. Yellow River, 37, 28-31(2015).
[10] Monte Carlo Methods in Financial Engineering. Springer(2004).
[11] Catastrophe theory as a model for change in fluvial systems. Adjustments of the Fluvial System, 13-32(1979).
[12] et alFormation and development of hump reach in Lower Yellow River. Journal of Sediment Research, 38-42(2012).
[14] et alCoupled geomorphic and habitat response to a flood pulse revealed by remote sensing. Ecohydrology, 10(2017).
[16] Spatial process simulation. Springer International Publishing, Cham, 369-404(2015).
[18] Nonlinear forecasting using nonparametric transfer function models. Wseas Transactions on Business & Economics, 157, 151-164(2009).
[19] Spatial width oscillations in meandering rivers at equilibrium. Water Resources Research, 48, 375-390(2012).
[20] Fractals: Form, chance, and dimension. Leonardo, 32, 65-66(1979).
[21] A stochastic approach for the description of the water balance dynamics in a river basin. Hydrology & Earth System Sciences, 12, 1189-1200(2008).
[22] Option pricing when underlying stock returns are discontinuous. Working Papers, 3, 125-144(1976).
[23] Effects of river flow scaling properties on riparian width and vegetation biomass. Water Resources Research, 43, W12406(2007).
[24] Non-linearity of reach hydraulic geometry relations. Journal of Hydrology, 388, 280-290(2010).
[25] Nonlinearity and unsteadiness in river meandering: A review of progress in theory and modelling. Earth Surface Processes & Landforms, 36, 20-38(2011).
[27] Theory of stochastic differential equations with jumps and applications: Mathematical and analytical techniques with applications to engineering. Springer Science &Business Media(2006).
[28] Likelihood methods for diffusions with jumps, Statistical inference in stochastic processes. Marcel Dekker Incorporated, 67-105(1991).
[29] Density estimation for statistics and data analysis. Technometrics, 29, 495(1987).
[33] River Dynamics and Integrated River Management. Berlin Heidelberg:Springer(2015).
[35] Relative scales of time and effectiveness of climate in watershed geomorphology. Earth Surface Processes and Landforms, 3, 189-208(1978).
[41] The study of the law of similarity for models of flood flows of the lower reach of the Yellow River. Beijing: Tsinghua University(1995).
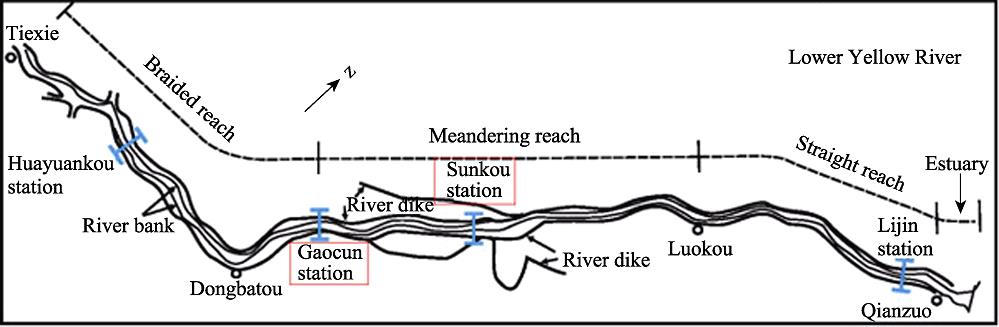
Set citation alerts for the article
Please enter your email address