Zhenbo Ren, Zhimin Xu, Edmund Y. Lam, "End-to-end deep learning framework for digital holographic reconstruction," Adv. Photon. 1, 016004 (2019)

Search by keywords or author
- Advanced Photonics
- Vol. 1, Issue 1, 016004 (2019)
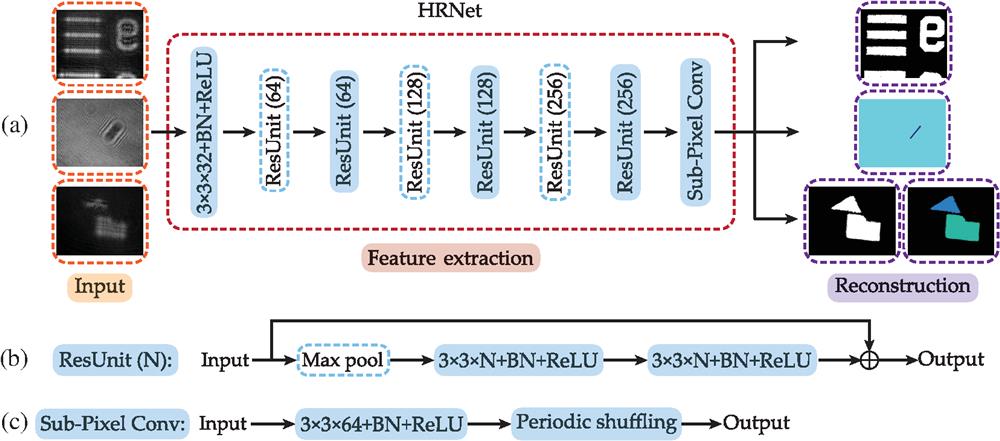
Fig. 1. (a) Schematic of the deep learning workflow and the structure of HRNet. It consists of three functional blocks: input, feature extraction, and reconstruction. In the first block, the input is a hologram of either an amplitude object (top), a phase object (middle), or a two-sectional object (bottom). The third block is the reconstructed output image according to the specific input. The second block shows the structure of HRNet; (b) and (c) elaborate the detailed structures of the residual unit and the subpixel convolutional layer, respectively.
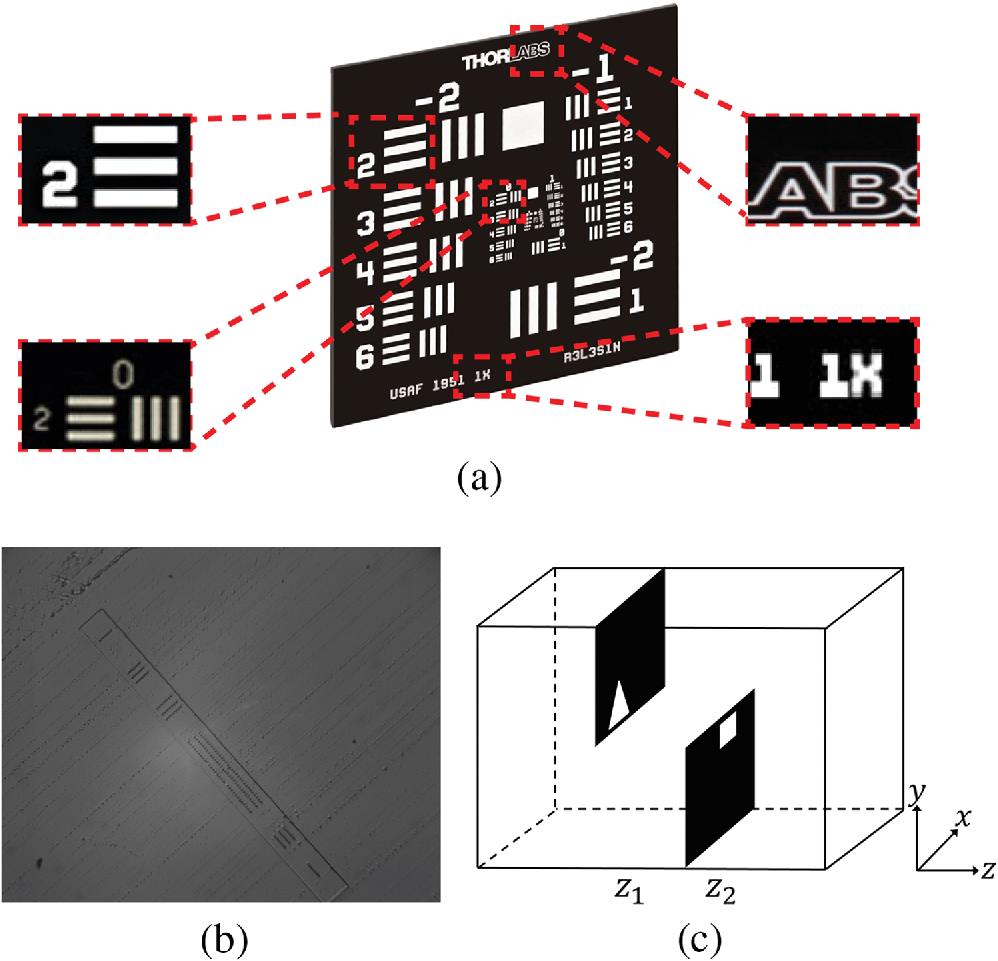
Fig. 2. (a) The USAF test target and its local areas as amplitude objects. (b) A customized groove on an optical wafer as the phase object. (c) A homemade two-sectional object consisting of a transparent triangle and a rectangle located at different axial positions.
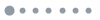
Fig. 3. Experimentally collected testing holograms of amplitude objects.
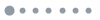
Fig. 4. (a)–(d) Ground-truth images and reconstructed images of holograms in Fig. 3 using (e)–(h) HRNet, (i)–(l) ASM, and (m)–(p) CONV.
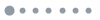
Fig. 5. Experimentally collected testing holograms of the phase object.
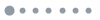
Fig. 6. (a)–(d) Ground-truth images and reconstructed quantitative phase images of holograms in Fig. 5 using (e)–(h) HRNet, (i)–(l) PCA, and (m)–(p) DE. The unit of the color bar is radian.
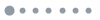
Fig. 7. Experimentally collected testing holograms of the two-sectional object.
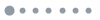
Fig. 8. Ground-truth: (a)–(d) EFI and (e)–(h) DM. HRNet, reconstructed: (i)–(l) EFI and (m)–(p) DM. Entropy, reconstructed: (q)–(t) EFI and (u)–(x) DM. T-gradient, reconstructed: (y)–(ab) EFI and (ac)–(af) DM. Variance, reconstructed: (ag)–(aj) EFI and (ak)–(an) DM. The color bar shows the depth in DM; the unit is mm.
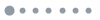
Fig. 9. (a) and (b) Holograms. (c) and (d) Frequency spectra. (e) and (f) Reconstructed images under different angles.
![Holograms [(a) and (b)] and reconstructed images [(c) and (d)] under different axial distances.](/Images/icon/loading.gif)
Fig. 10. Holograms [(a) and (b)] and reconstructed images [(c) and (d)] under different axial distances.
|
Table 1. Comparison of reconstruction performance for the amplitude object among ASM, CONV, and HRNet.
|
Table 2. Comparison of reconstruction performance for the phase object among PCA, DE, and HRNet.
|
Table 3. Comparison of EFI and DM reconstruction performance for the two-sectional object among SEN, VRA, TEN, and HRNet.
|
Table 4. Detailed description of the layers and parameters of the proposed HRNet (biases are ignored in the computation).
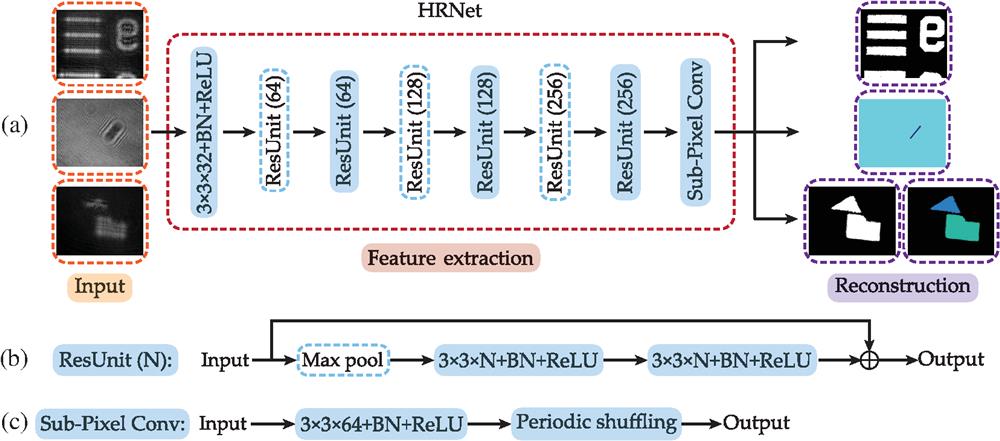
Set citation alerts for the article
Please enter your email address