Abstract
Digital holography records the entire wavefront of an object, including amplitude and phase. To reconstruct the object numerically, we can backpropagate the hologram with Fresnel–Kirchhoff integral-based algorithms such as the angular spectrum method and the convolution method. Although effective, these techniques require prior knowledge, such as the object distance, the incident angle between the two beams, and the source wavelength. Undesirable zero-order and twin images have to be removed by an additional filtering operation, which is usually manual and consumes more time in off-axis configuration. In addition, for phase imaging, the phase aberration has to be compensated, and subsequently an unwrapping step is needed to recover the true object thickness. The former either requires additional hardware or strong assumptions, whereas the phase unwrapping algorithms are often sensitive to noise and distortion. Furthermore, for a multisectional object, an all-in-focus image and depth map are desired for many applications, but current approaches tend to be computationally demanding. We propose an end-to-end deep learning framework, called a holographic reconstruction network, to tackle these holographic reconstruction problems. Through this data-driven approach, we show that it is possible to reconstruct a noise-free image that does not require any prior knowledge and can handle phase imaging as well as depth map generation.1 Introduction
Since its invention in 1948, holographic imaging has been a powerful technique in recording the diffracted wavefront of a three-dimensional (3-D) scene.1 A significant step forward from analog holography is to record digitally the interference pattern with an electronic sensor and to reconstruct the object numerically, including the amplitude and phase information, with a computer.2 Due to its noninvasive and label-free properties, digital holography (DH) has been applied to biological imaging,3,4 air quality monitoring,5 and surface characterization,6,7 to name just a few application areas.
Numerical reconstruction in DH is commonly based on the Fresnel–Kirchhoff integral,8 which, however, cannot be directly implemented due to its complexity. Simplifying it results in several numerical algorithms, such as the Fresnel approach,9 paraxial transfer function approach (also called convolution method, or CONV),10 and nonparaxial transfer function approach (also called angular spectrum method, ASM for short).11 More recently, compressed sensing12 has also been studied for holographic reconstruction.
Many of these methods share in common the need for detailed knowledge about the experimental setup, such as the wavelength of the laser, pixel pitch of the camera, and the object distance. The last one is normally estimated through autofocusing algorithms, many of which are computationally intensive and time-consuming.13,14 Additional steps, such as phase shifting15 and filtering in the frequency domain,16 are also necessary to suppress the zero-order and twin images, before using Fresnel propagation or Fourier transform to reconstruct the wavefront.
Sign up for Advanced Photonics TOC. Get the latest issue of Advanced Photonics delivered right to you!Sign up now
Phase imaging in DH presents additional challenges for the reconstruction process. The wavefront is first reconstructed using ASM or CONV, then the phase is obtained by calculating the angle of the complex amplitude. However, it is usually wrapped within and has aberration due to the objective or reference beam.17 To obtain the true phase, unwrapping algorithms such as the weighted least square fitting technique,18 the Goldstein branch-cut approach,19 and the quality-guided method20 have to be used, yet they are normally slow and too sensitive to give a successful result.21 To avoid phase unwrapping and to compensate for the phase aberration, one can opt for either additional hardware,17,22,23 which can involve bulky and expensive optical components, or algorithms that make strong assumptions about the imaging process.24,25
In many microscopy applications, it is highly desirable to obtain images in which the entire 3-D object is in focus and the depth information is shown to the user. These are known as extended focused imaging (EFI) and depth map (DM) reconstruction.26 DH, compared to conventional optical microscopy, is particularly suited for these tasks since it can record 3-D information in a single hologram. Current computational algorithms are based on selecting different portions in sharp focus from a stack of reconstructed images,27 solving a regularized minimization problem that may converge slowly,28 or using additional hardware.29 The situation is thus similar to phase imaging, where neither bulky optical hardware nor computationally intensive algorithms seem to be the best approach.
In recent years, deep learning has emerged as a rapidly developing technique that benefits various application areas such as image processing, computer vision, and natural language processing.30,31 This powerful tool has also been shown to be useful to holography. In Ref. 32, Nguyen et al. proposed to use deep learning for phase aberration compensation in digital holographic microscopy.32 A simplified U-net, which is trained only for binary background detection, works as an intermediate tool to preprocess the unwrapped aberrated phase images. This is followed by Zernike polynomial fitting, the ASM method, and phase unwrapping for a final reconstructed phase image. In Ref. 33, a deep neural network is trained for twin-image and self-interference artifacts elimination in lens-free in-line DH.33 The in-focus backpropagation of the hologram is fed into the network for training. Despite its success in noise removal, the network only accepts reconstructed complex wavefront (reconstructed amplitude and phase in two channels separately), thus conventional reconstruction algorithms are still required beforehand. The prediction quality also significantly drops for defocused reconstruction that is out of the depth-of-field (DOF) of the system, which is only . More recently, Wu et al.34 demonstrated the use of deep learning for performing autofocusing and phase-recovery to extend the DOF in an on-chip holographic microscope. However, not only is the backpropagated hologram fed into the trained network as input but also a conventional autofocusing method, known as “Tamura of the gradient,”35 and a conventional reconstruction method, ASM, have to be employed before obtaining a reconstructed image.
Recently, we proposed to tackle the autofocusing by treating it as a classification problem36 and furthermore, a regression problem37 handled in an effective way using a learning-based nonparametric method. The object is then reconstructed with the distance that is predicted from the raw hologram, using the CONV method. It is natural to ask: inasmuch as the object distance can be obtained from the hologram directly, can we go down the road to holographic image reconstruction? Apart from reconstruction directly from the hologram, can we also achieve different reconstruction tasks with one single algorithm, without the need to design specific ones for different tasks? Motivated by this, in this paper, we propose an end-to-end deep learning-based framework, called a holographic reconstruction network (HRNet), for numerical reconstruction in DH. By adopting this end-to-end learning strategy, raw holograms are directly fed into the network as input for training. As such, the network automatically learns internal representations of the necessary processing steps in holographic reconstruction and builds up a connection in pixel-level between a raw hologram and its backpropagation. In contrast to previous approaches, in this way, the network can output a noise-free reconstruction without the necessity to know any physical parameters of the imaging system or to implement any further auxiliary processing. Apart from demonstrating the usefulness of this method in reconstructing amplitude objects, we also show its use in recovering quantitative phase and significantly extending the DOF by reconstructing the EFI and DM for a multisectional object. Furthermore, we quantitatively compare the proposed method with the conventional ones for each modality, and the results demonstrate that the proposed method outperforms traditional methods significantly.
2 Method
Intrinsically, a hologram captured by a camera is a two-dimensional (2-D) intensity image recording the whole information of a 3-D scene. Reconstructing the object’s complex wavefront is to extract useful information hidden in the interference pattern, or in other words, to map the hologram to its amplitude and/or phase. Mathematically, deep learning is capable of approximating any continuous functions if the number of fitting parameters can grow indefinitely.38 This great flexibility, together with the development of many effective training algorithms in this field, motivates us to employ this new powerful tool to find the mapping for holographic reconstruction in a new manner.
For many deep learning-based tasks, the network depth is of crucial importance. A deeper network has more fitting parameters and can enrich the level of features representing the data; yet, along with more layers is the problem of a vanishing/exploding gradient. To ease the training of a deep neural network, the method called deep residual learning can be used, which explicitly adds an identity mapping between layers to significantly speed-up computation.39 Nevertheless, as a general principle for any application, there is a delicate balance between having a deeper network and avoiding excessive computational load. Taking serious consideration of this trade-off between performance and training load, in accordance with the generic residual learning principle, we design a deep residual network of moderate depth, HRNet, to achieve end-to-end holographic image reconstruction. The network architecture is shown in Fig. 1 (see Sec. 6 for detailed analysis).
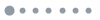
Figure 1.(a) Schematic of the deep learning workflow and the structure of HRNet. It consists of three functional blocks: input, feature extraction, and reconstruction. In the first block, the input is a hologram of either an amplitude object (top), a phase object (middle), or a two-sectional object (bottom). The third block is the reconstructed output image according to the specific input. The second block shows the structure of HRNet; (b) and (c) elaborate the detailed structures of the residual unit and the subpixel convolutional layer, respectively.
In Fig 1(a), the framework consists of three functional blocks: input, feature extraction, and reconstruction. In the first block, the input is a hologram of either an amplitude object (top), a phase object (middle), or a two-sectional object (bottom). For each reconstruction, respective datasets are prepared and the network is trained separately. The second block, HRNet, consists of three basic units. The first unit is a convolutional layer of 32 feature maps of size , with a batch normalization (BN) layer, which normalizes the output in each hidden layer, and a nonlinear activation layer using a rectified linear unit (ReLU), which is defined as .40 The second unit is the residual unit, which is denoted as “ResUnit (),” with a depth of . This residual unit consists of a max-pooling layer, two identical layers composing of a convolutional layer with feature maps of size , a BN layer, and a ReLU layer. The input of each ResUnit is identically mapped and added to its output for skip connection. The residual unit is then repeated six times with different depths. Note that the max-pooling layer, which is denoted as “max pool” and would prevent the network from overfitting, only exists in the dashed ResUnit. This is because max-pooling would divide the size of image in each dimension by half, leading to odd dimensions and thus difficulty in the subsequent upsampling operation. The third unit in HRNet is a subpixel convolutional layer denoted as “Sub-Pixel Conv.” Rather than conventional transposed convolution methods that have numerous trainable parameters, here we utilize the recent subpixel convolution method for upscaling the reduced intermediate image to its original size.41 It consists of a “” convolutional layer, a BN layer, a ReLU layer, and a periodic shuffling operation. After a regular convolutional layer, a specific type of image reshaping, periodic shuffling, is performed to build a high-resolution image in a single step. Since the image size is downsampled by a factor of 8 in each dimension due to max-pooling, the periodic shuffling here is to rearrange the elements of a tensor to a tensor of shape . By doing so, an image with the original resolution is recovered, which is why the earlier convolutional layer in front of the periodic shuffling has a depth of 64. This parameter-free resizing operation can save computational load and time significantly, compared to commonly used U-Net architecture. For detailed explanation of this method, we refer readers to Ref. 41. In the last block, according to respective input data, the network gives the corresponding reconstructed images.
Mathematically, suppose for the first convolutional layer, the input hologram data are denoted as . The function to be learned at this layer can then be expressed as where and represent the learnable weights and biases at the first layer, and denotes the convolution operation.
As for the ’th intermediate ResUnit, given the input , the function to be learned at the ’th layer is where and represent the weights and biases abstracted from the two convolutional layers in one ResUnit at layer , and the pooling layer is omitted here for simplicity of notation.39
To alleviate computational load and prevent overfitting, HRNet contains three max-pooling layers, thus the input image size is downsampled with a scale factor of 8 along the forward propagation in the network. Therefore, the same upscaling factor of 8 is necessary in the subpixel convolutional layer. At this layer, only the first step, 2-D convolution, has parameters to be updated along training, whereas the second step is parameter-free, reducing the number of trainable parameters of the network. Therefore, the function at this layer can be expressed as where and represent the feature maps and biases at the final layer, and PS represents the periodic shuffling operation. Finally, with these functional layers, learning an end-to-end mapping function requires estimating network parameters . This is achieved through minimizing the loss function between the predicted images and the ground-truth images . Given a set of holograms and their corresponding ground-truth images as labels, we define the loss function as pixelwise mean squared error where denotes the number of images in a minibatch. The training process stops after finishing all preset epochs. Test set is then fed into the network to test the performance of the network in predicting new data.
3 Experimental Results and Comparisons
The DH setup used in this paper consists of a typical lens-free Mach–Zehnder interferometer. Apart from the standard components in an interferometer, additionally, two linear motion controllers (Newport, CONEX-LTA-HL) are used to control the movement of the object axially and laterally in the object arm.37 The reference beam and object beam propagating along two arms separately interfere at the hologram plane, and thus a fringe pattern is generated and recorded by a detector. In addition, adjustments on the angle between the reference beam and object beam and exposure of detector are done by manual operations on the mirror and camera. Note that no objective lens is included in the setup, leading to a unit magnification of the system. Three different kinds of objects are selected and placed at the object plane as samples. The amplitude objects, as shown in Fig. 2(a), are various areas of a negative USAF 1951 test target (Thorlabs R3L3S1N). For each holographic acquisition, a small local area on the target is imaged and recorded with unit magnification in the transmission mode. The second sample, which is a phase-only object, is a customized groove with tiny structures made on an optical wafer using lithography. In Fig. 2(b), it is imaged using a microscope with a objective lens. The third one is a two-sectional object consisting of a transparent triangle and a transparent rectangle on the proximal and distal planes to the camera. The axial distance between the two discrete sections is 5 mm, as shown in Fig. 2(c).
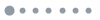
Figure 2.(a) The USAF test target and its local areas as amplitude objects. (b) A customized groove on an optical wafer as the phase object. (c) A homemade two-sectional object consisting of a transparent triangle and a rectangle located at different axial positions.
The proposed HRNet model follows the train-validate-test scheme. The collected hologram data are randomly split into three subsets with a ratio of 80:10:10 for training, validation, and testing. Before training the network, all the weights and biases are initialized using truncated normalization method with a standard deviation of 0.1 and the biases are initialized with a constant of 1. Considering the training time and memory limitation, in every iteration, only a small batch of 10 holograms, called a minibatch, of the entire training set is fed into the network. Each hologram has a size of , which is cropped from the original . The loss function, Eq. (4), is minimized using the Adam optimizer,40 which is an extension of the stochastic gradient descent optimization method. A critical parameter in the optimizer, known as the learning rate, controls the gradient descent velocity in optimization. It is empirically set to be 0.01, and as the training progresses, it decays exponentially with a rate of 0.9. In each minibatch training, the weights and biases are automatically updated after one iteration of optimization. The proposed network is implemented using TensorFlow and all the experiments are performed in a Ubuntu 16.04.2 environment with an Intel Core i7 920 processor (2.67 GHz, 8 cores), 24 GB of RAM, and an Nvidia GTX 760.
3.1 Amplitude Object
As described above, for each acquisition, a small local area on the resolution chart is imaged and digitally stored as a hologram. After recording one hologram, either the chart is laterally or axially moved by adjusting the motion controller, or the incident angle of two beams is slightly changed by rotating the mirror, to create a new hologram. The axial position is set around 295 mm (may differ in a range of ). The exposure time and gain of the camera are set as 10 ms and 2, respectively, to ensure the maximal pattern contrast. By doing so, more than 10,000 holograms are collected as the dataset. For each hologram, its corresponding ground-truth image is required as the label image to feed into the HRNet simultaneously for supervised training. The label image is obtained by numerically backpropagating the raw hologram using the CONV method, and then the noisy reconstructed image is carefully and manually cleaned for artifacts removal. In Fig. 3, we provide an example showing four of the testing holograms. The label images of holograms in Fig. 3 are shown in Figs. 4(a)–4(d).
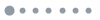
Figure 3.Experimentally collected testing holograms of amplitude objects.
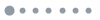
Figure 4.(a)–(d) Ground-truth images and reconstructed images of holograms in Fig. 3 using (e)–(h) HRNet, (i)–(l) ASM, and (m)–(p) CONV.
The training stage for the amplitude object is stopped after iterations of minibatch training, which equals 25 epochs, meaning that the network is trained for around 25 times throughout the training subset. As the network is getting trained, representative features of the holograms are learned by the network, leading to reduction of loss and update of weights and biases. Test subset, which is never seen before by the network, is then fed into the network for prediction. The machine-predicted reconstructions of holograms in Fig. 3 are shown in Figs. 4(e)–4(h). They are visually identical to the ground-truth images, illustrating the successful reconstruction with the proposed method from raw holograms.
Furthermore, we compare HRNet with conventional approaches, ASM and CONV. For the conventional ones, parameters of the optical setup, such as the laser wavelength (632.8 nm), pixel pitch of the camera (), and object distance (around 295 mm) have to be known a priori. In addition, the 0 and spectra need to be removed in the frequency domain. Since the angle between the two beams is also changed in acquisition, determining the positions of the band-pass filter has to be done manually. In Figs. 4(i)–4(p), the reconstructed images using ASM and CONV are shown. Although by and large the conventional methods can also correctly reconstruct the object, it can be observed that there is significant noise in the background and artifacts around the object, whereas the predicted images by HRNet are noise-free. The superior performance of using deep learning for holographic reconstruction is evident.
Lastly, to make a quantitative comparison among the three methods, the peak signal-to-noise ratio (PSNR) and structural similarity (SSIM)42 between the reconstructed and ground-truth images are calculated and given in Table 1. The score is the average value among the individual subset. From the table, we can see that ASM and CONV have a similar performance in both PSNR and SSIM. Although in computational time the three methods are rather close, the proposed HRNet outperforms them markedly in reconstruction quality. These results illustrate that the deep learning method can significantly improve the reconstructed image quality better than conventional methods by a substantial margin.
Measure | Methods | Amplitude dataset |
Validation | Test |
PSNR (dB) | ASM | 17.66 | 19.64 |
CONV | 19.68 | 20.54 |
HRNet | 25.99 | 24.62 |
SSIM | ASM | 0.20 | 0.19 |
CONV | 0.26 | 0.26 |
HRNet | 0.92 | 0.91 |
Time (s) | ASM | 1.56 | 1.49 |
CONV | 1.35 | 1.72 |
HRNet | 1.14 | 1.21 |
Table 1. Comparison of reconstruction performance for the amplitude object among ASM, CONV, and HRNet.
3.2 Phase Object
Apart from reconstructing an amplitude object, we also quantitatively reconstruct the phase object in Fig. 2(b) using the proposed HRNet. Since the groove is customized by design, the 3-D information of the sample is thus known a priori (length and width are 1.1 and 0.1 mm, and the thickness is ). The data collection process is similar to that of the amplitude object, and finally we collect 2500 holograms in which the phase object is located at different spatial positions. Several holograms used for testing are presented in Fig. 5 [without the magnification as shown in Fig. 2(b)].
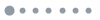
Figure 5.Experimentally collected testing holograms of the phase object.
As the thickness of the groove (140 nm) is already known, given the wavelength (HeNe laser source, Thorlabs HNL100L-EC, ), the refractive indices of the material (fused silica, ) and the ambiance (air, ), the sample’s true phase can be calculated by , which is around 2 rad. The ground-truth quantitative phase image is then acquired by manually cleaning the initial phase image, which is obtained by conventional phase unwrapping and aberration compensation.25,43 The label images of Fig. 5 are shown in Figs. 6(a)–6(d). The hologram and label data are split following the same scheme to train the HRNet, and the training process is stopped after 25 epochs. The trained network is then to predict holograms in test subset, and the output images of holograms in Fig. 5 are presented in Figs. 6(e)–6(h). Note that the quantitative phase image is the direct output of the network, with which phase unwrapping and aberration are avoided.
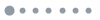
Figure 6.(a)–(d) Ground-truth images and reconstructed quantitative phase images of holograms in Fig. 5 using (e)–(h) HRNet, (i)–(l) PCA, and (m)–(p) DE. The unit of the color bar is radian.
In addition, we compare the performance of HRNet with commonly used phase aberration compensation approaches, PCA25 and double exposure (DE).17 The former assumes that the phase aberration has only linear and spherical components. The latter requires an additional reference hologram, in which the object is removed from the optical path. This reference hologram should be recorded instantly after the object hologram in order to avoid random fluctuation of laser, read noise of camera, shot noise, and vibration of the ambiance. In addition, phase unwrapping has to be used after compensating the phase aberration. Here we use least squared fitting for both approaches to obtain the true phase. Reconstructed quantitative phase images using PCA and DE are shown in Figs. 6(i)–6(l) and 6(m)–6(p), respectively. Quantitative measurements of PSNR and SSIM of the three methods are given in Table 2.
Measure | Methods | Phase dataset |
Validation | Test |
PSNR (dB) | PCA | 10.12 | 9.53 |
DE | 8.94 | 8.68 |
HRNet | 30.35 | 30.49 |
SSIM | PCA | 0.13 | 0.11 |
DE | 0.12 | 0.10 |
HRNet | 0.96 | 0.96 |
Time (s) | PCA | 1.96 | 1.93 |
DE | 2.09 | 2.15 |
HRNet | 1.06 | 1.20 |
Table 2. Comparison of reconstruction performance for the phase object among PCA, DE, and HRNet.
As can be seen, the phase images obtained by PCA and DE are full of artifacts, especially at the corners. The object is even difficult to observe in Figs. 6(i) and 6(m). In contrast, HRNet reconstructs the best phase image that is free from noise and artifacts. Not surprisingly, in Table 2, HRNet has the best scores of PSNR and SSIM. These results clarify the significant improvement of the proposed method in reconstructing the quantitative phase image from a raw hologram of a phase object in an end-to-end manner. It is noteworthy that the conventionally generated phase images have a PSNR and SSIM about 10 dB and 0.1, resulting in significant improvements of HRNet by 20 dB and 0.85. It is understandable that conventional methods give rather small values since we calculate the PSNR and SSIM between the reconstruction and the binary ground-truth image. Thus, the difference at every pixel would accumulate and lead to a large error and a low similarity. In contrast, the improvements for amplitude reconstruction, in which the ground-truth images are grayscale, are only around 6 dB and 0.7 for PSNR and SSIM.
It is also worth noting that, for the conventional approaches, not only do the same parameters used for amplitude reconstruction in Sec. 3.1 have to be known, but also additional phase aberration compensation algorithm and phase unwrapping algorithm are needed. In contrast, these requirements are avoidable for HRNet. Phase aberration is automatically compensated, and afterward the aberration-free phase is also automatically unwrapped during the forward propagation of input hologram along the network. We also note that, in practice, DH is usually used for measuring the phase quantitatively in biology and microelectronics. For these cases, the ground-truth information may not be available before measurement, and thus the method described here for creating the label image cannot be adopted. However, in some specific applications such as malaria-infected red blood cells detection44 and microelectronics surface defects detection,45 the sample is basically deterministic. Thus, the true phase information of the sample can be acquired using iterative (Gerchberg–Saxton algorithm, ptychographical iterative engine) or noniterative (transport of intensity equation) phase retrieval approaches a priori.46 Once the label image is acquired, the network can then be trained and needs to be trained only once with the holograms and the label images. Afterward, the well-trained network can then be used to predict for detecting new malaria-(non)infected red blood cells or micro/nanostructures.44 The proposed method is thus potentially applicable for quantitative phase imaging, and pushing this proof-of-concept study for practical applications is straightforward.
3.3 Two-Sectional Object
Apart from the single-sectional object discussed above, multisectional samples are not rare in DH.26 We make a two-sectional sample, as shown in Fig. 2(c), to verify the capability of the proposed framework and totally we collect 2000 holograms by spatially shifting the object. Several testing holograms are presented in Fig. 7 for example. In Figs. 7(a) and 7(c), the triangle and the rectangle are located at 280 and 285 mm. And they are at 285 and 290 mm in Fig. 7(b), and at 277 and 282 mm in Fig. 7(d). To get rid of defocus noise and to achieve 3-D imaging, an all-in-focus image and a DM are desired. Therefore, here we realize the two reconstruction modalities, EFI and DM, using HRNet. With EFI and DM, it is easy to obtain the sectioning images by setting a proper threshold, and thus the network is not particularly trained for sectioning image reconstruction here. However, we would like to emphasize that training HRNet for sectioning is straightforward.
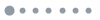
Figure 7.Experimentally collected testing holograms of the two-sectional object.
Similar method in Sec. 3.1 is utilized to generate ground-truth images for EFI and DM. Then, the network is separately trained for EFI and DM in consideration of the training speed and memory. The training is stopped after 25 epochs and testing set is fed into the network. For comparison, conventional methods for EFI and DM reconstruction based on self-entropy (SEN), variance (VAR), and Tenenbaum gradient (TEN)26 are selected for comparison. To implement these methods, an estimated range where the two sections may be located, for example, 270 and 295 mm, has to be known a priori. Within this range, sequential numerical reconstruction (16 reconstructions) is performed and these metrics are calculated for every pixel within a window (). Reconstructed images using these four methods and quantitative comparison results are given in Fig. 8 and Table 3. Not surprisingly, HRNet notably outperforms conventional methods in both visualization and quantitative measurements. Although conventional methods can basically reconstruct the EFI and DM, due to the coarse distance sampling and unavoidable noise in the experiments, pervasive artifacts exist at the background in EFI, and the focal distances of the two sections are indistinguishable in DM. In addition, since the HRNet is free from sequential numerical reconstruction, the feedforward prediction is fast (as given in Table 3, around 1 s), whereas the other three methods need a much longer processing time (normally for a coarse sequential reconstruction within the given range in our setting). As such, HRNet can not only provide a high-quality estimation of EFI and DM but also a substantial decrease in computation time, compared to conventional cumbersome metric-based methods.
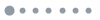
Figure 8.Ground-truth: (a)–(d) EFI and (e)–(h) DM. HRNet, reconstructed: (i)–(l) EFI and (m)–(p) DM. Entropy, reconstructed: (q)–(t) EFI and (u)–(x) DM. T-gradient, reconstructed: (y)–(ab) EFI and (ac)–(af) DM. Variance, reconstructed: (ag)–(aj) EFI and (ak)–(an) DM. The color bar shows the depth in DM; the unit is mm.
Measure | Methods | EFI | DM |
Validation | Test | Validation | Test |
PSNR (dB) | SEN | 16.82 | 15.92 | 12.66 | 12.78 |
VAR | 15.44 | 14.69 | 12.78 | 11.92 |
TEN | 16.03 | 15.86 | 11.82 | 12.24 |
HRNet | 35.64 | 35.72 | 37.81 | 36.70 |
SSIM | SEN | 0.28 | 0.27 | 0.80 | 0.80 |
VAR | 0.10 | 0.11 | 0.82 | 0.82 |
TEN | 0.14 | 0.10 | 0.80 | 0.80 |
HRNet | 0.97 | 0.97 | 0.97 | 0.98 |
Time (s) | SEN | 380.30 | 392.68 | 390.03 | 391.36 |
VAR | 384.38 | 386.52 | 390.58 | 388.82 |
TEN | 376.76 | 383.66 | 398.29 | 394.37 |
HRNet | 1.35 | 1.30 | 1.04 | 1.42 |
Table 3. Comparison of EFI and DM reconstruction performance for the two-sectional object among SEN, VRA, TEN, and HRNet.
4 Discussions
Here, we further explore the capability of the trained network under various situations. As the amplitude object is the most common case in DH, and other reconstruction modalities are based on amplitude reconstruction, the following experiments and discussions are performed under this situation.
4.1 Different Incident Angles
As explained in Sec. 3.1, for conventional holographic reconstruction methods, normally the spectrum needs to be manually selected and retained in order to remove the 0 and spectra in off-axis holography. As such, whenever the incident angle between the two beams changes, either due to new experiment or adjustment of the fringe contrast, manual operations are needed for reconstruction. Since we are tackling holographic reconstruction from raw holograms in a nonparametric fashion, it is critical to test the performance of the network under the situation of different incident angles. Therefore, we record holograms of different angles and feed them into the trained network (which did not see holograms of these angles in training). In Fig. 9, two holograms captured under different angles and their corresponding frequency spectra are shown. We can see that the spectra of the two holograms are fairly different, as annotated with the red markers. Note that the hologram in Fig. 9(a) has a different fringe contrast, but this kind of hologram also appears in the previous training set.
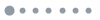
Figure 9.(a) and (b) Holograms. (c) and (d) Frequency spectra. (e) and (f) Reconstructed images under different angles.
As can be seen from Figs. 9(e) and 9(f), although the holograms are recorded under different angles, the network can still output reconstructed images in good quality, illustrating that the network is capable of reconstruction regardless of variation in the incident angles. In other words, even if the mirrors in a setup have a slight rotation, the proposed method can still perform well.
4.2 Different Axial Distances
In Sec. 3.1, the training data consist of holograms recorded at several discrete longitudinal distances. In reality, however, it is impossible and unnecessary to place objects at every single position and collect data. Therefore, it is critical to consider how well the network can perform if an object is located at distances different from those in the training set. To test this, we retrain the network with holograms of which the object is located at 295 mm. Then, we feed holograms recorded with distances of 303 and 280 mm into the trained network for reconstruction. In Fig. 10, we show the testing holograms and reconstructed images by the network. Although the network is trained with only one particular distance, it can still give a good output for different distances. This experiment demonstrates that the network has learned the underlying characteristics of holograms, and the object can be reconstructed in a straightforward way without the need to search for the object distance by autofocusing.37
![Holograms [(a) and (b)] and reconstructed images [(c) and (d)] under different axial distances.](/Images/icon/loading.gif)
Figure 10.Holograms [(a) and (b)] and reconstructed images [(c) and (d)] under different axial distances.
5 Conclusions and Future Work
To conclude, an end-to-end learning architecture, HRNet, is presented for numerical reconstruction in DH. Various reconstruction modalities, including amplitude reconstruction, quantitative phase imaging, EFI, and DM reconstruction, are demonstrated to verify its efficacy and superiority in image quality. With a single network architecture, or equivalently a single algorithm, various reconstruction modalities can be implemented with different training data. This all-in-one characteristic avoids time-consuming computation and intermediate algorithm design. Furthermore, it is easy to retrain a well-trained network with new data to extend or refine the performance of the network. We believe that the proposed framework has considerable potential and wide applicability in object detection, particle tracking, and super-resolution, making DH more accessible and leading to exciting new applications.
Note that the present data collection strategy and the network training have some limitations. The dataset consists of multiple similar objects, which are different parts of the resolution target. Although effective, it would be of greater interest to extend this method to microscopic samples. As mentioned before, an advantage of the learning-based technique is that the well-trained network can be retrained when new data are available. When new microscopic samples are available, it is straightforward to retrain the well-trained network for extension. Therefore, data collection of microscopic specimens with DHM configuration is crucial and will be our main work in the future.
6 Appendix A: Details of the Proposed Network
The detailed parameters of the proposed HRNet are given in Table 4.
Layer number | Layer type | Configuration | Number of parameters |
Layer 1 | 2-D convolution | 3 × 3 × 32 + BN + ReLU | 3 × 3 × 32 = 288 |
Layer 2 | ResUnit (64) | Max-pooling: 2 × 2 3 × 3 × 64 + BN + ReLU 3 × 3 × 64 + BN + ReLU | Parameter-free 3 × 3 × 32 × 64 = 18,432 3 × 3 × 64 × 64 = 36,864 |
Layer 3 | ResUnit (64) | 3 × 3 × 64 + BN + ReLU 3 × 3 × 64 + BN + ReLU | 3 × 3 × 64 × 64 = 36,864 3 × 3 × 64 × 64 = 36,864 |
Layer 4 | ResUnit (128) | Max-pooling: 2 × 2 3 × 3 × 128 + BN + ReLU 3 × 3 × 128 + BN + ReLU | Parameter-free 3 × 3 × 64 × 128 = 73,728 3 × 3 × 128 × 128 = 147,456 |
Layer 5 | ResUnit (128) | 3 × 3 × 128 + BN + ReLU 3 × 3 × 128 + BN + ReLU | 3 × 3 × 128 × 128 = 147,456 3 × 3 × 128 × 128 = 147,456 |
Layer 6 | ResUnit (256) | Max-pooling: 2 × 2 3 × 3 × 256 + BN + ReLU 3 × 3 × 256 + BN + ReLU | Parameter-free 3 × 3 × 128 × 256 = 294,912 3 × 3 × 256 × 256 = 589,824 |
Layer 7 | ResUnit (256) | 3 × 3 × 256 + BN + ReLU 3 × 3 × 256 + BN + ReLU | 3 × 3 × 256 × 256 = 589,824 3 × 3 × 256 × 256 = 589,824 |
Layer 8 | Subpixel convolution | 3 × 3 × 64 + BN + ReLU + periodic shuffling | 3 × 3 × 256 × 64 = 147,456 |
Total parameters | | | 2,857,248 |
Table 4. Detailed description of the layers and parameters of the proposed HRNet (biases are ignored in the computation).
References
[1] D. Gabor. A new microscopic principle. Nature, 161, 777-778(1948).
[2] U. Schnars et al. Digital Holography and Wavefront Sensing(2015).
[3] A. C. Chan, K. K. Tsia, E. Y. Lam. Subsampled scanning holographic imaging (SuSHI) for fast, non-adaptive recording of three-dimensional objects. Optica, 3, 911-917(2016).
[4] F. Merola et al. Tomographic flow cytometry by digital holography. Light Sci. Appl., 6, e16241(2017).
[5] Y. Wu et al. Air quality monitoring using mobile microscopy and machine learning. Light Sci. Appl., 6, e17046(2017).
[6] E. Cuche, P. Marquet, C. Depeursinge. Simultaneous amplitude-contrast and quantitative phase-contrast microscopy by numerical reconstruction of Fresnel off-axis holograms. Appl. Opt., 38, 6994-7001(1999).
[7] Y. Pourvais et al. Microstructural surface characterization of stainless and plain carbon steel using digital holographic microscopy. J. Opt. Soc. Am. B, 34, B36-B41(2017).
[8] M. Born, E. Wolf. Principles of Optics: Electromagnetic Theory of Propagation, Interference and Diffraction of Light(1999).
[9] P. Picart, J. Leval. General theoretical formulation of image formation in digital Fresnel holography. J. Opt. Soc. Am. A, 25, 1744-1761(2008).
[10] J. W. Goodman. Introduction to Fourier Optics(2017).
[11] T. M. Kreis, M. Adams, W. P. Jüptner. Methods of digital holography: a comparison. Proc. SPIE, 3098, 224-233(1997).
[12] D. J. Brady et al. Compressive holography. Opt. Express, 17, 13040-13049(2009).
[13] Z. Ren, N. Chen, E. Y. Lam. Automatic focusing for multisectional objects in digital holography using the structure tensor. Opt. Lett., 42, 1720-1723(2017).
[14] H. A. Ilhan, M. Doğar, M. Özcan. Digital holographic microscopy and focusing methods based on image sharpness. J. Microsc., 255, 138-149(2014).
[15] I. Yamaguchi, T. Zhang. Phase-shifting digital holography. Opt. Lett., 22, 1268-1270(1997).
[16] E. Cuche, P. Marquet, C. Depeursinge. Spatial filtering for zero-order and twin-image elimination in digital off-axis holography. Appl. Opt., 39, 4070-4075(2000).
[17] T. Colomb et al. Total aberrations compensation in digital holographic microscopy with a reference conjugated hologram. Opt. Express, 14, 4300-4306(2006).
[18] D. C. Ghiglia, L. A. Romero. Robust two-dimensional weighted and unweighted phase unwrapping that uses fast transforms and iterative methods. J. Opt. Soc. Am. A, 11, 107-117(1994).
[19] R. M. Goldstein, H. A. Zebker, C. L. Werner. Satellite radar interferometry: two-dimensional phase unwrapping. Radio Sci., 23, 713-720(1988).
[20] M. Zhao et al. Quality-guided phase unwrapping technique: comparison of quality maps and guiding strategies. Appl. Opt., 50, 6214-6224(2011).
[21] D. C. Ghiglia, M. D. Pritt. Two-Dimensional Phase Unwrapping: Theory, Algorithms, and Software, 4(1998).
[22] D. Parshall, M. K. Kim. Digital holographic microscopy with dual-wavelength phase unwrapping. Appl. Opt., 45, 451-459(2006).
[23] W. Qu et al. Digital holographic microscopy with physical phase compensation. Opt. Lett., 34, 1276-1278(2009).
[24] L. Miccio et al. Direct full compensation of the aberrations in quantitative phase microscopy of thin objects by a single digital hologram. Appl. Phys. Lett., 90, 041104(2007).
[25] C. Zuo et al. Phase aberration compensation in digital holographic microscopy based on principal component analysis. Opt. Lett., 38, 1724-1726(2013).
[26] Z. Ren, N. Chen, E. Y. Lam. Extended focused imaging and depth map reconstruction in optical scanning holography. Appl. Opt., 55, 1040-1047(2016).
[27] P. Ferraro et al. Extended focused image in microscopy by digital holography. Opt. Express, 13, 6738-6749(2005).
[28] X. Zhang, E. Y. Lam, T.-C. Poon. Reconstruction of sectional images in holography using inverse imaging. Opt. Express, 16, 17215-17226(2008).
[29] M. K. Kim. Wavelength-scanning digital interference holography for optical section imaging. Opt. Lett., 24, 1693-1695(1999).
[30] Y. LeCun, Y. Bengio, G. Hinton. Deep learning. Nature, 521, 436-444(2015).
[31] D. Shen, G. Wu, H.-I. Suk. Deep learning in medical image analysis. Annu. Rev. Biomed. Eng., 19, 221-248(2017).
[32] T. Nguyen et al. Automatic phase aberration compensation for digital holographic microscopy based on deep learning background detection. Opt. Express, 25, 15043-15057(2017).
[33] Y. Rivenson et al. Phase recovery and holographic image reconstruction using deep learning in neural networks. Light Sci. Appl., 7, 17141(2018).
[34] Y. Wu et al. Extended depth-of-field in holographic image reconstruction using deep learning based auto-focusing and phase-recovery. Optica, 5, 704-710(2018).
[35] Y. Zhang et al. Edge sparsity criterion for robust holographic autofocusing. Opt. Lett., 42, 3824-3827(2017).
[36] Z. Ren, Z. Xu, E. Y. Lam. Autofocusing in digital holography using deep learning. Proc. SPIE, 10499, 104991V(2018).
[37] Z. Ren, Z. Xu, E. Y. Lam. Learning-based nonparametric autofocusing for digital holography. Optica, 5, 337-344(2018).
[38] G. Cybenko. Approximation by superpositions of a sigmoidal function. Math. Control Signals Syst., 2, 303-314(1989).
[39] K. He et al. Deep residual learning for image recognition, 770-778(2016).
[40] I. Goodfellow, Y. Bengio, A. Courville. Deep Learning(2016).
[41] W. Shi et al. Real-time single image and video super-resolution using an efficient sub-pixel convolutional neural network, 1874-1883(2016).
[42] Z. Wang et al. Image quality assessment: from error visibility to structural similarity. IEEE Trans. Image Process., 13, 600-612(2004).
[43] J. Bioucas-Dias et al. Absolute phase estimation: adaptive local denoising and global unwrapping. Appl. Opt., 47, 5358-5369(2008).
[44] A. Anand et al. Automatic identification of malaria-infected RBC with digital holographic microscopy using correlation algorithms. IEEE Photonics J., 4, 1456-1464(2012).
[45] M. A. Schulze et al. Semiconductor wafer defect detection using digital holography. Proc. SPIE, 5041, 183-193(2003).
[46] M. Mir et al. Quantitative phase imaging. Prog. Opt., 57, 133-217(2012).