Abstract
With rapid advancement and deep integration of artificial intelligence and the internet-of-things, artificial intelligence of things has emerged as a promising technology changing people’s daily life. Massive growth of data generated from the devices challenges the AIoT systems from information collection, storage, processing and communication. In the review, we introduce volatile threshold switching memristors, which can be roughly classified into three types: metallic conductive filament-based TS devices, amorphous chalcogenide-based ovonic threshold switching devices, and metal-insulator transition based TS devices. They play important roles in high-density storage, energy efficient computing and hardware security for AIoT systems. Firstly, a brief introduction is exhibited to describe the categories (materials and characteristics) of volatile TS devices. And then, switching mechanisms of the three types of TS devices are discussed and systematically summarized. After that, attention is focused on the applications in 3D cross-point memory technology with high storage-density, efficient neuromorphic computing, hardware security (true random number generators and physical unclonable functions), and others (steep subthreshold slope transistor, logic devices,etc.). Finally, the major challenges and future outlook of volatile threshold switching memristors are presented.1. Introduction
From smart home[1], smart city[2] to smart planet[3], artificial intelligence (AI) plays an important role that will greatly change people’s daily lives and human civilization. With numerous flows of data streamed from internet-of-things (IoT) devices that are increasing by a billion per year, the ability to process data in an effective manner is urgently demanded. Consequently, the artificial intelligence of things (AIoT) has emerged as a promising technology attracting much attention from industry and academia[4]. Deep integration of AI and IoT allows the connected physical objects (intelligent devices) not only exchange information with each other, but also process data and make decisions at the edge. In AIoT systems, IoT devices act as the “body” that can interact with real environments (light, sound, gas molecules,etc.), collecting data and creating a network for transmitting critical information to the processing center, while AI is the “brain” that can store information and process data by machine learning. Nowadays, AIoT has been integrated into our surroundings and own wide applications in various fields[5-10] like social networks, industry, agriculture, architecture, health care,etc. It is of great significance to push the development of AIoT technology.
However, the AIoT system is facing several challenges to be solved. (1) High storage-density of memory chip is increasingly demanded. Thanks to the development of 3D integration technology[11], more memory units with smaller space and lower cost become possible. (2) Advanced computing technology with high energy efficiency is desired for timely and effective data-processing. Neuromorphic computing technology such as deep neural network (DNN) and spiking neural network (SNN) appears in recent years[12]. The employment of DNN and SNN can contribute to the realization of in-memory computing and in-sensor computing, which improve the energy efficiency in the edge devices like smart phones, wearable sensors, and unmanned vehicles. (3) Hardware security has to be considered during data communication and information sharing. The increased security threats to the internet and sensor networks must be simultaneously addressed to improve data confidentiality[13].
The solutions to the above challenges in AIoT cannot be separated from the exploration of emerging nano-devices. One of the most promising candidates is the memristor, which was proposed by Chua in 1971 as the fourth elemental circuit component[14]. Memristors have advantages of simple structure, high 3D integration potential, high compatibility with complementary metal oxide semiconductor (CMOS) and low power consumption. According to the retention time of low resistance state (LRS), memristors can be divided into volatile and nonvolatile types. As for nonvolatile memristors, LRS can be maintained after the removal of voltage bias. Nevertheless, the volatile memristors would spontaneously return back to HRS from LRS after the removal of the external electric field in milliseconds to nanoseconds. Volatile memristors, also known as threshold switching (TS) devices, have broad applications in the various fields[15-20] of perceptron, memory array, neuromorphic computing, true random number generation (TRNG),etc. As shown inFig. 1, volatile TS memristors help to enable the entire process of data and information collection, storage, processing, and communication in AIoT systems.
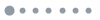
Figure 1.(Color online) Overview of volatile TS memristors enabling the entire process of data and information collection, storage, processing, and communication in AIoT systems.
In the review, we introduce the device, switching mechanisms, and applications of volatile TS memristors. In Section 2, volatile TS memristors are classified into three categories: metallic conductive filament (CF) type, amorphous chalcogenide-based ovonic threshold switching (OTS), and metal-insulator transition (MIT) type. Their materials, structures, switching mechanisms, and characteristics are summarized. The applications of volatile TS memristor in selectors, artificial neurons, nociceptors, and hardware security are discussed in details in Section 3. In the last section, the challenges and perspectives are presented.
2. Categories and physics
The volatile TS memristor is one kind of two-terminal devices with sandwich structures (electrode/dielectric/electrode), where the dielectric layer plays a key role in resistive switching. A great number of materials have been explored as the switching layer in the past few decades including oxides[21-29], 2D films[30-32], perovskites[33], chalcogenides[34-36] and others[37-39]. In fact, there is no difference in structure and materials between volatile and nonvolatile memristors, and even one device could exhibit both volatile and nonvolatile properties at different compliance currents[39]. According to the switching mechanisms, volatile TS memristors can be divided into three categories: metallic conductive filament, OTS and MIT. Their typical structures andI–V characteristics are presented inFig. 2 and switching mechanisms will be discussed below in detail.
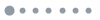
Figure 2.(Color online) Categories and switching mechanisms of volatile TS memristors.
2.1. Metallic conductive filament type
Volatile TS devices principally enabled by metallic conductive filaments are also called diffusive memristors, which have attracted tremendous attention because of the simple device structure, easy fabrication, and high compatibility with CMOS lines[40,41]. Metallic CF formation involves the following three successive processes: (I) oxidation of the metal atoms into mobile cations (X → Xn+ +ne−) at the interface between the active electrode (Ag or Cu) and dielectrics under an influence of external electric field, (II) migration of cations towards the inert electrode, (III) reduction of cations (Xn+ +ne− → X) and the nucleation of active metal nanocluster. When the discrete nanoclusters grow and connect the electrodes, the filament forms and the device turns into low resistance state. The transition from HRS to LRS occurs within a finite time duration, known as delay time. The delay effect is believed to be a consequence of ionic or atomic motion induced filament growth, and delay time is dependent on the amplitude and width of the applied voltage pulse. Yanget al.[42,43] performed an experimental observation of filament growth viain-situ high-resolution transmission electron microscopy (HRTEM) technology, and constructed the relationship between filament evolution and the kinetic parameters of dielectrics including ion mobility (μi) and redox rate (Г). The growth modes can be roughly categorized into four types: (1) an inverted cone shape with growing from the inert electrode when bothμ andГ are high, (2) a forward cone shape with discrete nanoclusters growing from the active electrode when bothμ andГ are low, (3) nucleation inside the dielectric and re-connection with the source whenμ is low butГ is high, and (4) a branched structure growing from the inert electrode whenμ is high butГ is low.
Once the filaments form, the interplay between filament and surrounding environments determines their evolution. With the decrease or removal of the external electric field, the filament tends to rupture spontaneously, leading to the transition from LRS to HRS within a finite time duration (relaxation time). To have a better understanding on the spontaneous rupture features of diffusive memristors, researchers have paid huge efforts to study the relevant physics[44-48]. Among them, the theory of interfacial energy minimization is widely adopted. Wanget al.[45] observed the spontaneous relaxation in Au/SiOxNy:Ag/Au device usingin-situ HRTEM, and it was demonstrated that minimizing the interfacial energy between Ag nanoparticles and the dielectrics served as the driving force for these diffusive memristors. The material systems exhibiting a substantial relaxation dynamics are those with large contact angles[49], such as MgOx:Ag, SiOxNy:Ag, HfOx:Ag. The spontaneous rupture of filaments is dominated by the surface diffusion of metal atoms. Apart from the interfacial energy minimization, Songet al.[50] proposed that there was steric repulsion between Ag filaments and TiO2 matrix in Ag/TiO2 systems. To minimize the steric repulsion, Ag 5s electrons in filaments are transferred to the Ti 3d states, leading to the oxidation (rupture) of Ag filaments and the formation of localized Ti3+ ions. In addition, Ambrogioet al.[51] indicated that the tensile stress existed in the dielectrics, changing the migration barriers to/from the active electrode and accelerating the atoms back to the active electrode, which resulted in the spontaneous rupture of the filament.
2.2. OTS type
In the 1960s, Ovshinsky[52] systematically described the OTS phenomenon and patented his discovery, which opened up a new field of research. The OTS phenomenon was found in many disordered materials, particularly amorphous chalcogenides with band gap between 0.6 and 1.4 eV.Fig. 2 shows a typicalI–V curve of the OTS glass, in which the device initially presents off-current at the level of nA. When the applied voltage exceeds the threshold valueVth, the device switches into LRS and turns back to the original HRS if the voltage decreases lower than the hold valueVhold. The switching process is highly repeatable and theI–V characteristics remain almost unchanged with millions of cycles in a well-processed OTS device. In some amorphous chalcogenides, Joule heating induced crystallization of glass may happen, and unexpected memory switching (MS) occurs where LRS remains after removing the applied voltage. They are also called phase-change materials and widely used in the next-generation information storage products such as PCRAM. To fabricate the OTS device without an MS effect, amorphous chalcogenides with high activation energy of crystallization are advised.
To date, various theories have been developed to explain the OTS behavior. The field-induced nucleation model[53] points out that the electric field reduces the energy barrier of nucleation and the critical nucleus size, promoting the spontaneous growth of crystal nucleus. Once the electric field is removed, the energy barrier recovers and the as-grown crystalline nucleus become unstable and quickly dissolve. The nucleation model, even though similar to the growth of conductive filaments, does not totally comply with the experimental facts. The crystallization rate of OTS materials is usually extremely fast (~nanoseconds), and the nucleus size easily grows large enough over the critical value, inevitably resulting in the memory switching. The number of the filaments in the devices are also far less than the required defect density (~ 1019 cm−3) for the TS behavior[54].
A more competent model to explain the OTS phenomenon is the electronic effect instead of the atomic movement. In early years, researchers believed that the voltage bias could heat the semiconductor and activate electrons to the conduction band, increasing the current density which results in higher temperature and more conducting electrons, leading to a “thermal breakdown”. Later, Ielminiet al.[55] revealed that Joule heating is not a mandatory process in the OTS devices. It is well known that the band gap in the amorphous semiconductors is filled by localized trap states due to the Anderson localization of electronic wave functions and defective homopolar bonds, and the conducting electrons are easily trapped in these defect states. The external voltage could reduce the energy barriers to activate the trapped electrons, and thus increase the conductivity, similar to the trap-limited Poole-Frank mechanism. This pure electronic effect can explain the fast and durable switching of OTS devices well. However, the theory was recently challenged by a few research groups who discovered that there are subtle structural and bonding changes upon the TS process. Guoet al.[56] found that some homopolar filaments formed in the glass and Ratyet al.[57] believed that the electric excitation drives the formation of the meta-valent bonds (a new type of chemical bond in PCMs proposed by Wuttiget al.[58,59]). The switching mechanisms in OTS devices are still elusive and need in-depth explorations.
2.3. MIT type
The MIT in vanadium oxides (VOx) and niobium oxide (NbOx) has been extensively studied for almost one century[60], but the exact mechanism remains a challenge in the contemporary condense matter physics, impeding the applications of these materials on modern electronic devices. The transition of VO2, the archetypal material, takes place at about 341 K, where VO2 transforms from a high-temperature metallic rutile structure (TiO2-type) to a low-temperature insulating monoclinic phase. The transition can be triggered by various perturbations, such as temperature, light, pressure and chemical doping. In electronic devices, the MIT phenomenon occurs under an influence of external electric field and leads to resistive switching, as presented inFig. 2.
There has been a debate over the mechanism of the transition: structure distortion (Peierls type)[61] versus electron correlation (Mott-Hubbard type)[62]. The metallic phase at high temperature is naturally due to the overlap of the valence band and conduction band, leading to free electron transport. The controversy mostly concentrates on the low-temperature insulator. Schulzet al.[63] performed unconstrained ab-initio molecular dynamics simulations (single-electron approximation) on the insulating phase of VO2, and found that the low-temperature ground-state structure indeed exists and it opens the band gap because of the Peierls distortion. They concluded that the insulating behavior could be simply ascribed to structure transition that leads to the change of electronic structure, and thus the strong electron–electron correlation becomes obsolete to account. However, the vast majority of literature[64,65] pointed out that the low-temperature VO2 is a Mott-Hubbard insulator, raising strong opposition to the above “band insulator” view. The theory contributes insulating feature to the Coulomb repulsion between electrons instead of the lack of free electrons in the conduction bands, and the transition is a consequence of strong correlative interaction between electrons rather than structure distortion. Recently, the above two mechanisms could be simultaneously investigated in the same MIT materials[66,67].
Concerning the electric field-induced transition in electronic devices, the mechanism is also under heavy debate in the scientific community. Joule heating due to current flow is an obvious candidate for explaining the transition[68-70]. However, it has been argued that the electric field applied in the process may induce the transition non-thermally without heating the materials to its critical temperature. Kalcheimet al.[71] demonstrated that a purely non-thermal electrical MIT can occur in both VO2 and V2O3 nanowires. The mechanism behind the non-thermal effect was identified as field-assisted carrier generation leading to a doping-driven MIT. Later, Valleet al.[72] used in-operando optical reflectivity to capture the growth dynamics of the metallic phase with space and time resolution. They demonstrated that growth of the metallic phase during the field-driven MIT can be explained just by considering the effect of Joule heating, but the transition of the first metallic domains remained unclear.
3. Roles of volatile TS devices in AIoT applications
3.1. Selectors
To meet the demand of high-density storage in AIoT systems, resistive memory technology with 3D cross-point architecture appears and attracts much attention[45,73]. Resistive memory devices have extremely high densities and ultra-low power consumption due to its simple two-terminal structure and reducing device size as small as 4F2 (F is the minimal feature size). However, the cross-point array is always suffering from the sneak path current from neighbor cells during write or read operations, as shown inFig. 3(a). The problem would reduce the read accuracy and increase power consumption, which hinder the large-scale integration. Consequently, selectors are integrated with memory devices at each cross-point cell to deal with the sneak current problem[19]. Among various types of selectors, two-terminal selectors are more prone to 3D cross-point integration, which is considered as one-selector-one-resistor (1S1R) configuration (Fig. 3(b)). To limit the sneak current from the unselect or half-select memory element during both read and write operation, an ideal selector should have high non-linearity (on/off ratio) and ultra-low leakage current (Ioff~ 1 pA), as presented inFig. 3(c). In addition, the selector should be compatible with the memory (PCRAM or RRAM), including threshold voltageVth, resistance, polarity and endurance. Herein, selectors based on three-types of TS devices are discussed in detail below.
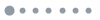
Figure 3.(Color online) Cross-point memory technology in 1S1R configuration. (a) Schematic illustration of sneak path current in cross-point array during read operation. “0” means off-state, while “1” stands for on-state. (b) Memory with integration of selector to limit the sneak current. (c) Requirements of an ideal selector in cross-point memory.
3.1.1. CF-based selectors
As an emerging memory technology, RRAM is often used for embedded or standalone applications. To reach a high integration density, the most important characteristics of selectors are high selectivity (lowIoff and largeIon), low operation voltage, and steep switch slope. The TS devices dominated by metallic CF formation and spontaneous rupture can meet these strict requirements. Taking TaOx RRAM[74] as an example, the reset operation happens at the current of 50μA, implying that the CF selectors should maintain volatility above 50μA. Other performance and reliability metrics should also match with the state-of-the-art RRAMs, such as switching speed (less than 10 ns), endurance (over 1012), threshold voltage, uniformity, and bidirectional switching.
Until now, there have been various dielectrics demonstrating superior threshold switching characteristics with the active top electrode (Ag or Cu), such as HfO2, ZrO2, SiO2, TaOx, MoS2, SiTe alloys, amorphous Si, amorphous C,etc. Their performances are listed inTable 1. Among them, oxides are widely exploited due to their high compatibility with RRAM and relatively reliable performances. Midyaet al.[75] achieved an extremely high non-linearity (1010) with an Ag/HfO2/Pd device, as shown inFigs. 4(a)–4(e). The device had an ultra-low leakage current at the level of 0.1 pA, and threshold switching happened at ~0.15 V with slope less than 1 mV/decade. The on-current also reached 1 mA. The Ag/HfO2/Pd selector was integrated with TaOx RRAM andI–V curves of the 1S1R cells were exhibited inFig. 4(e). The switching on and off speed was 75 and 250 ns, respectively. However, the endurance was unsatisfactory (~108 cycles). The cycle-to-cycle (C2C) and device-to-device (D2D) uniformity ofVth were poor. These days, in order to improve the performance of metallic CF-based selectors, numerous methods are proposed to regulate and control the CF evolution.
Table Infomation Is Not Enable
![(Color online) Performance enhancement of CF-based selectors from active electrode engineering. (a) SEM image and cross-sectional TEM image of Pd/Ag/HfO2/Ag/Pd/TaOx/Ta2O5/Pd devices. (b) DCI–V characteristic of the Ag/HfO2/Pd selector yields a selectivity of 1010. (c) The device shows a switching slope of 1 mV/decade. (d) switching speed and endurance performance of the selectors. (e) DCI–V characteristic of the vertically integrated selector and RRAM (1S1R). (f) Cross-sectional TEM image of the Ag nanodots/HfO2/Pt device. (g) Threshold switching behavior at different compliance currents from 100 nA to 1 mA. (h) The device has an extremely small switching slope of <1 mV/decade. (i) Read resistance following turn-on operation with compliance currents. (j) DCI–V characteristic of the integrated 1S1R device before and after 108 cycles. (k) Highly improved stable TS in AgTe alloy device with 104 DC cycles. (l) Endurance of the AgTe alloy TS device (109 cycles). (m) Cross-sectional TEM image of the AgGeSe/Al2O3/Pt device. (n) The selector with a 100 nm feature size shows repeatable TS characteristics at a compliance current of 1 mA, providing a 12.7 MA/cm2 on-current density. Reproduced with permission from (a–e) Ref. [75] Copyright 2017 Wiley-VCH, (f–j) Ref. [77] Copyright 2019 Wiley-VCH, (k, l) Ref. [80] Copyright 2020 IEEE Publishing, (m-o) Ref. [81] Copyright 2021 IEEE Publishing.](/Images/icon/loading.gif)
Figure 4.(Color online) Performance enhancement of CF-based selectors from active electrode engineering. (a) SEM image and cross-sectional TEM image of Pd/Ag/HfO2/Ag/Pd/TaOx/Ta2O5/Pd devices. (b) DCI–V characteristic of the Ag/HfO2/Pd selector yields a selectivity of 1010. (c) The device shows a switching slope of 1 mV/decade. (d) switching speed and endurance performance of the selectors. (e) DCI–V characteristic of the vertically integrated selector and RRAM (1S1R). (f) Cross-sectional TEM image of the Ag nanodots/HfO2/Pt device. (g) Threshold switching behavior at different compliance currents from 100 nA to 1 mA. (h) The device has an extremely small switching slope of <1 mV/decade. (i) Read resistance following turn-on operation with compliance currents. (j) DCI–V characteristic of the integrated 1S1R device before and after 108 cycles. (k) Highly improved stable TS in AgTe alloy device with 104 DC cycles. (l) Endurance of the AgTe alloy TS device (109 cycles). (m) Cross-sectional TEM image of the AgGeSe/Al2O3/Pt device. (n) The selector with a 100 nm feature size shows repeatable TS characteristics at a compliance current of 1 mA, providing a 12.7 MA/cm2 on-current density. Reproduced with permission from (a–e) Ref. [75] Copyright 2017 Wiley-VCH, (f–j) Ref. [77] Copyright 2019 Wiley-VCH, (k, l) Ref. [80] Copyright 2020 IEEE Publishing, (m-o) Ref. [81] Copyright 2021 IEEE Publishing.
Firstly, the device can be optimized from the aspect of the active electrode, which works as metal reservoirs of the filaments. Ag or Cu thin films as active electrodes cause the stochastic filament formation and stuck-on issues, which leads to poor cycle endurance. Therefore, Huaet al.[76] proposed highly ordered Ag nanodots with diameter of ~15 nm to replace Ag thin films. With the help of rapid thermal annealing (RTA) at 500 °C for 30 s[77], the Ag nanodots/HfO2/Pt device exhibited excellent threshold switching characteristics, as shown inFigs. 4(f)–4(j). The on-current reached 1 mA and the endurance was improved to 108 cycles. The mechanisms were believed to be the formation and rupture of multiple weak filaments. Ag nanodot structure restricted the silver atoms/clusters distribution at the interface of the top electrode and HfO2 dielectrics, inhibiting the excessive migration of silver. The RTA treatment could contribute to pre-diffusion of Ag atoms into the HfO2 matrix, which induced multiple narrow channels. The formed channels maintained weak filament formation at high compliance current. Apart from nanodots, nanotip electrodes are also demonstrated to be helpful in improving device performances. The compound electrode is another common method. Firstly reported by Gouxet al.[78] in 2011, a CuTe electrode helped improve the performance of TS devices. Due to larger Cu-Te bonding energy, Cu ions preferred to diffuse back to the active electrode when removing the external electric field, which could impair filament stability. An optimum composition range was determined considering thermal stability and surface morphology[79]. Based on a similar mechanism, Banerjeeet al.[80] used AgTe alloys as the active electrode and obtained higher endurance >109 cycles (Figs. 4(k) and4(l)). Later, Wanet al.[81] prepared AgGeSe compounds as the top electrode and achieved the highest on-current density ~12.7 MA/cm2 in the CF-based TS device (Figs. 4(m)–4(o)). Different from active electrodes, the inert electrode has a relatively indirect impact on the characteristics of CF selectors. Grisafeet al.[82] investigated the influence of inert metal work function on the threshold voltage. The work function difference between top and bottom electrodes could induce a built-in electric field, which could offset external bias. TheVth value increased as the work function of the inert electrode decreased. This effect offers a method to modulate the operating voltage of CF-based selectors.
Secondly, the interface between the electrodes and dielectric layer can be modified to improve device performances. According to the working mechanism of CF-based selectors, the interfaces greatly affect the redox reactions, charge transfer and ion migration. In the traditional active electrode/dielectrics/inert electrode structure, the oxidization and migration processes of active atoms are highly stochastic and the injection amount of active metal atoms is hard to control. Hence, a barrier layer is always inserted into the interface between the active electrode and dielectric layer to block active metal atoms. The most commonly used materials are TiN[27] due to the high impermeability.Figs. 5(a)–5(d) show performances of the Ag/TiN/HfO2/Pt device established by Grisafeet al.[82]. The stuck-on issues were solved and endurance was improved with the adoption of diffusion barrier. The thicker TiN layer caused higher forming voltage (Fig. 5(b)), which indicated that a proper injection amount of active metal atoms was important for performance improvement. Although the TiN barrier layer can effectively block the active atoms, it is still challenging to control the size and distribution of filaments precisely. Thanks to the unique characteristics of 2D materials, Zhaoet al.[83] used defective graphene to have a better control of the filament formation process. As shown inFig. 5(e), the control device Ag/SiO2/Pt transformed into non-volatile memory at anICC larger than 100μA. The Ag/concentrated-defect graphene (CDG)/SiO2/Pt device exhibited non-volatile behaviors at a relatively low current (1μA). However, the device with discrete-defect graphene (DDG) could maintain volatility at current up to 500μA. Besides, the device could exhibit bidirectional switching characteristics.
![(Color online) Performance enhancement of CF-based selectors from interface engineering. (a) False colored SEM and cross-sectional TEM images of the Ag/TiN/HfO2/Pt device. (b) Forming voltage dependence on TiN diffusion barrier thickness for 25 devices. (c)I–V characteristics of the selector with 3 nm TiN barrier. (d) Endurance comparison of the selectors with and without the TiN barrier. (e) Cell structure and typical DCI–V characteristics of the Ag/defective-Graphene/SiO2/Pt devices. Reproduced with permission from (a–d) Ref. [82] Copyright 2019 IEEE Publishing, (e) Ref. [83] Copyright 2018 Wiley-VCH.](/Images/icon/loading.gif)
Figure 5.(Color online) Performance enhancement of CF-based selectors from interface engineering. (a) False colored SEM and cross-sectional TEM images of the Ag/TiN/HfO2/Pt device. (b) Forming voltage dependence on TiN diffusion barrier thickness for 25 devices. (c)I–V characteristics of the selector with 3 nm TiN barrier. (d) Endurance comparison of the selectors with and without the TiN barrier. (e) Cell structure and typical DCI–V characteristics of the Ag/defective-Graphene/SiO2/Pt devices. Reproduced with permission from (a–d) Ref. [82] Copyright 2019 IEEE Publishing, (e) Ref. [83] Copyright 2018 Wiley-VCH.
Thirdly, the optimization of the dielectric layer is also an effective strategy since the cations migrate and react inside this layer. Plenty of methods can modify the microscopic structure of the dielectrics. For instance, doping is a common method. Yooet al.[44] doped amorphous Si with hydrogen in an Ag/a-Si/Pt device. Hydrogen not only removed sneak paths in amorphous Si but also enhanced Ag diffusivity, which induced lower off-current and higher hold voltage. To realize bi-directional switching, active metal atoms are often directly doped into the dielectric layer as ion reservoir, as shown inFig. 6(a). In this way, both positive or negative voltage can induce filament formation. Recently, Sahotaet al.[84] achieved highly reliable selection behavior with controlled Ag doping of nano-polycrystalline ZnO layer (Fig. 6(b)). 0.01 pA of leakage current was obtained and at the same time, C2C and D2D variation ofVth was less than 10% (Figs. 6(c) and6(d)). The reduced variations were attributed to anisotropic diffusion of Ag ions in the well-developed preferredc-axis oriented (002) crystalline ZnO (wurtzite) phase, in addition to restricted Ag ion influx modulated by doping. Multilayer structure is another simple and effective method. In a study of back-end-of-the-line (BEOL) compatible selectors, Luoet al.[85] inserted an undoped HfO2 layer into a Cu/Cu:HfO2/Pt device. The tunneling layer reduced the leakage current by more than 5 orders of magnitude, which could greatly increase the array density. Yinet al.[87] constructed homogeneous bilayer oxide TS device Ag/HfOy/HfOx/Pt wherex <y, as shown inFig. 6(e). The device exhibited electroforming-free properties, low threshold switching voltage of ~0.28 V, high on-current of ~300μA, and extremely sharp switching slope of ~0.6 mV/dec. The coupling mechanism between crystallite kinetics of the homogeneous interface and the evolution of Ag CFs was revealed (Fig. 6(f)). Migration of oxygen vacancy (VO) induced not only phase transformation of hafnium oxide from amorphous to monoclinic but also the rotation of grains, which interfered with the bonding strength of Ag filaments, assisted the evolution of Ag filaments, and generated selective effect. Heterogeneous bilayer oxides with different ion mobility are also proposed and exhibit good threshold switching characteristics, for example, Cu/GeTe/Al2O3/Pt[28]. More recently, tri-layer homogeneous oxides of HfOx/HfOy/HfOx (x<y) were reported by Luet al.[86], showing high non-linearity >1010 and high on-current up to 3 mA (Figs. 6(g) and6(h)). It was revealed that the discrete O–O bonds existed in the oxygen-rich HfOy layer, which could oxidize Ag atoms and result in weak filaments. The filament formation and rupture mainly happened in the HfOy layer and the interfaces between dielectrics could impair filament stability, which helps to increase the on-current.
![(Color online) Performance enhancement of CF selectors from dielectric engineering. (a) Repeatable highly nonlinear threshold switchingI–V characteristics for devices with different host lattices doped with silver. (b) Cross-sectional SEM image of the Pt/Ag:ZnO/Pt selector device. (c) Normal probability plot of Vth variation for selector devices in the case of device-to-device, sample-to-sample and batch-to-batch. (d) Transient characteristics of the selector devices with AC pulse test showing switching speed and relaxation time. (e) Cross-sectional TEM images and composition results of the Ag/HfOy/HfOx/Pt selector. (f) Schematic demonstration of the coupling mechanism between crystallite kinetics and Ag filaments. (g) Cross-sectional TEM image and DCI–V characteristic of the HfOx/HfOy/HfOxselector. (h) Schematic illustrations of the proposed TS model. Reproduced with permission from (a) Ref. [45] Copyright 2017 Springer Nature, (b–d) Ref. [84] Copyright 2021 IEEE Publishing, (e, f) Ref. [87] Copyright 2018 ACS Publishing, (g, h) Ref. [86] Copyright 2022 Wiley-VCH.](/Images/icon/loading.gif)
Figure 6.(Color online) Performance enhancement of CF selectors from dielectric engineering. (a) Repeatable highly nonlinear threshold switchingI–V characteristics for devices with different host lattices doped with silver. (b) Cross-sectional SEM image of the Pt/Ag:ZnO/Pt selector device. (c) Normal probability plot of Vth variation for selector devices in the case of device-to-device, sample-to-sample and batch-to-batch. (d) Transient characteristics of the selector devices with AC pulse test showing switching speed and relaxation time. (e) Cross-sectional TEM images and composition results of the Ag/HfOy/HfOx/Pt selector. (f) Schematic demonstration of the coupling mechanism between crystallite kinetics and Ag filaments. (g) Cross-sectional TEM image and DCI–V characteristic of the HfOx/HfOy/HfOxselector. (h) Schematic illustrations of the proposed TS model. Reproduced with permission from (a) Ref. [45] Copyright 2017 Springer Nature, (b–d) Ref. [84] Copyright 2021 IEEE Publishing, (e, f) Ref. [87] Copyright 2018 ACS Publishing, (g, h) Ref. [86] Copyright 2022 Wiley-VCH.
Herein, we have discussed many effective methods for performance improvement and some devices exhibit quite satisfying characteristics. However, CF-based selectors are still far from industrial application. Poor uniformity limits the operation accuracy in a dense crossbar array. As filaments rupture spontaneously, the turn-off speed (hundreds of nanoseconds) is relevantly slow compared to RRAM. Endurance is also not sufficient to match with RRAM since excess active metal atoms can easily cause stuck-on issues. Another challenge is the fabrication process. Although some methods mentioned above can improve performance greatly, they are not efficient and suitable for wafer-scale fabrication, such as nano-dots and defective graphene. High-quality selectors with compatible materials and simple structures are still demanded.
3.1.2. OTS selectors
OTS selectors usually provide large on-currentIon to drive the phase transition in PCRAM cells, in which the current should be large enough to heat and melt the phase-change material. Meanwhile, the highly densified 3D structure requires large selectivity and lowIoff as well. Besides the above critical parameters, an applicable OTS selector should present high endurance (several times more switching cycles than PCRAM materials), comparable switching speed to the PCRAM cell, and high stability to sustain high temperature in the BEOL process. To this end, the chalcogenide materials which contain S, Se, and Te elements stand out due to their excellent performance and full compatibility to PCRAM materials[88,89]. The doped chalcogenide glasses with high crystallization temperature remain amorphous when the electrical current breaks down. Strong covalent elements typically forming tetrahedral sp3 bonds (C, Si, As, etc.) are usually favorable to be added in the chalcogenides because the mixed amorphous alloys are rendered low fragility and slow crystallization rate.
The thermal stability of binary GexSe1–x-based selectors can exceed 350 °C, andVth can be adjusted by changing the thickness and compositions. The device shows the high on-current density of 23 MA/cm2 with a fast-switching speed of 2 ns and withstands 108 writing cycles. However, theIoff and selectivity are still not satisfactory. To this end, Navarroet al.[91] fabricated the Ge-rich Ge–Se compounds by co-sputtering of Ge and Ge30Se70 targets. In principle, the increase of Ge content results in more defect states, which can be easily excited by electric fields[110,111]. The Ge58Se42 selector showed an improved selectivity (~105), lowIoff (<10−10 A), and good device endurance up to 109. But the switch speed (~50 ns),Jon (1.5 MA/cm2), andVth (3.4 V) remain insufficient. It was demonstrated that the performance of the Ge–Se OTS device could be tailored by changing the stoichiometry and there was a trade-off betweenVth and the crystallization temperature (Tc)[90]. To break the performance limit of Ge–Se materials, the strong covalent dopants[92-96] such as N, C, and As were used to enhance the amorphous network and further increaseTc. For example, N doping could both reduceIoff and improve the thermal stability (up to 600 °C). Addition of N will introduce strong Ge–N bonds to replace the homopolar Ge–Ge bonds, enhancing the barrier to break these bonds and weakening the diffusion of elements. The Ge–Se–N OTS device[92] shows lowIoff (2 nA), high selectivity of 105, high on-current density of 23 MA/cm2, and endurance of 108 cycles. The As-doped Ge-Se appears to be more balanced in all parameters. Ab initio simulations found that As ions can act as donors to pump electrons to the shallow states which originate from the nonbonding electrons in the defective tetrahedral clusters. The Ge–Se–As device presentedJon of 11.1 MA/cm2,Vth of 2.5 V, and selectivity of 106 (as shown inFigs. 7(a) and7(b))[93].
![(Color online) Te-based and GeSe-based OTS selectors. (a) Schematic of the TiN/Ge-Se/W device structure in an As-doped GeSe OTS selector. (b) The OTSI–V curves of the GeSe-based device. (c) Four Te-based OTS materials show good thermal stability. (d) TheJ–V characteristics of the W/C-Te/W device with good selectivity of >105. (e) Cross-sectional TEM images of single-element Te device. (f)JON parameters versus electrode diameter in various OTS devices. Reproduced with permission from (a, b) Ref. [104] Copyright 2018 IEEE Publishing, (c, d) Ref. [93] Copyright 2019 ACS Publishing, (e, f) Ref. [110] Copyright 2021 Science Publishing.](/Images/icon/loading.gif)
Figure 7.(Color online) Te-based and GeSe-based OTS selectors. (a) Schematic of the TiN/Ge-Se/W device structure in an As-doped GeSe OTS selector. (b) The OTSI–V curves of the GeSe-based device. (c) Four Te-based OTS materials show good thermal stability. (d) TheJ–V characteristics of the W/C-Te/W device with good selectivity of >105. (e) Cross-sectional TEM images of single-element Te device. (f)JON parameters versus electrode diameter in various OTS devices. Reproduced with permission from (a, b) Ref. [104] Copyright 2018 IEEE Publishing, (c, d) Ref. [93] Copyright 2019 ACS Publishing, (e, f) Ref. [110] Copyright 2021 Science Publishing.
Te-based glasses are also one of the most developed materials family, such as GeTe4[108], GeTe6[97], B–Te[105], and C–Te[109], showing large on-current density (Jon >10 MA/cm2), relatively large selectivity (Ion/Ioff>105), and ultrafast switching speed (several nanoseconds). The first Te-based selector material GeTe6, unfortunately, has poor thermal stability with low crystallization temperature (Tc) of ~300 °C and the phase separation issue is rather prominent after several hundreds of cycles. Reducing the content of Te and adding Si into compounds could improve thermal stability due to the formation of strong Si-Te bonds. Kooet al.[102] replaced Ge by Si, demonstrating a low Ioff of 1 nA, high selectivity of 106, and fast switching speed of 2 ns. But at high temperature around 300°C, the leakage current will increase by one order of magnitude. Alternatively, C0.35Te0.65, B0.25Te0.75 and Al0.3Te0.7 selectors[104-107] also present good selectivity (>105) and lowIoff(<5 nA). The thermal stability of C–Te and B–Te based devices can reach up to 450 °C, but they suffer from lowVth (~0.7 V) and insufficient endurance (< 108).Figs. 7(c) and7(d) shows the thermal stability of several Te-based binary OTS devices. The high-entropy-like materials such as Ge–As–Te–Si and Ge–As–Te–Si–N OTS materials[98-101] could overcome the above drawbacks. It made the As-contained materials the state-of-the-art OTS selectors in modern 3D phase-change memory products. However, the alloying of As is not environment-friendly and they also suffer from the chemical composition complexity which are not compatible with CMOS lines.
Recently, Shenet al.[110] reported a single-element tellurium (Te) OTS device, exhibiting largeJON (>11 MA/cm2), fast switching speed (<20 ns), and high endurance (109 cycles), as shown inFigs. 7(e) and7(f). In-situ HRTEM investigations were carried out for monitoring the switching process of the TiN/Te/TiN devices, and the crystalline-liquid-crystalline phase transition mechanism was confirmed in the pure elemental-Te switch. It was demonstrated that the device performance matched well with those of GST-based PCRAM cells, which did not deteriorate severely after series connection with PCRAM cells. The intrinsically homogeneous composition and BEOL compatibility of the Te device not only cut the time-consuming material-optimization process but also enable aggressive size downscaling toward sub-10-nm dimensions for realizing 3D memory chips with the highest density. Hence, simplifying the composition as well as new device structures becomes the next battlefield for the OTS research.
3.1.3. MIT selectors
Although MIT devices have a fairly high on-state current (>1 mA) and relatively high endurance (>108), small on/off ratio (~100) limits their developments in the selector field. Meanwhile, VO2, one of the main MIT materials with a transition temperature of only 341 K possesses poor thermal stability. The other main material, NbO2, has multiple oxidation states[112] limiting the NbO2-based MIT selector.
Many efforts have been made to improve the performance of MIT devices. One reliable option is doping such as Al and Ti elements[113-115]. Metal impurities help to decrease the leakage current. They also induce oxygen vacancy pathways in the electroforming process, which improve the uniformity. In addition, the precise control of the oxygen content in the film can also help performance enhancements of NbOx-based MIT devices. Luoet al.[116] and their subsequent work[117] performed an in-depth study on the effect of oxygen flux in magnetron sputtering, and achieved extremely high endurance (~1012), fast switching speed at the level of 10 ns, and large on-current density (4.8 MA/cm2).
In summary, the three types of selectors have distinct characteristics. CF-based selectors have the highest selectivity and lowest leakage current, which are promising for 1S1R memory technology, but they have the poorest endurance and uniformity due to the intrinsic stochasticity, which greatly limit the applications. Improvements of endurance and on-current are still in development. OTS selectors have very high compatibility with PCM devices, but the selectivity and leakage current have to be improved. The advantages of MIT selectors are fast switching speed, but they have the smallest selectivity. Devices with CMOS compatible materials and simple structures should be considered to reduce fabrication cost. In addition, co-design of selector and memory should be paid more attention because an inappropriate match can cause severe performance degradation.
3.2. Neuromorphic computing
3.2.1. Artificial neuron
With the restriction of the von Neumann bottleneck and the ending of Moore’s law, search for advanced computing technologies is in high demand. Therefore, brain-inspired neuromorphic computing technologies with non-von Neumann architectures appear and play important roles in AIoT systems. They attract much attention from industry and academia owing to its parallel computing capability and lower energy consumption. Hardware implementation is an important step during the development of neuromorphic computing. Among various nueromorphic devices, artificial neuron is one of the crucial issues.
In a biological system, as shown inFig. 8(a), dendrites work as the input of the neuron which receive and integrate the signals from other neurons through the synapses, while the axon works as the output of the neuron, connected with the dendrites of the nearby neurons through synapses[118]. To describe the behavior of neurons, many mathematical models have been established, such as integrate-and-fire (IF)[119], leaky integrate-and-fire (LIF) and Hodgkin–Huxley (HH) model[120]. The HH model systematically describes the dynamic behavior of the membrane potential in terms of various ion channels of the soma and dendrites, which is very complicated for hardware implementation. The IF model, however, gives a concise description of biological behavior,i.e. the neuron receives input from the presynaptic neuron and then generates excitatory or inhibitory postsynaptic currents. The membrane potential will gradually increase and the action potential is triggered when the membrane potential reaches a threshold value. The LIF model solves the problem that the membrane potential keeps constant when the input is withdrawn, which is contrary to the actual biological behavior. Thus, the LIF model is widely applied in the current SNN.
![(Color online) (a) Schematic diagram of biological neurons. Presynaptic currents are integrated in neurons. When the membrane potential reaches a certain threshold, an action potential is generated. (b) The circuit of LIF neuron. The capacitor acts as the membrane and implements integration effect via the charging process. The resistor is used to limit the current through the VO2-based MIT device, which is the output signal of the neuron. (c) LIF neurons with voltage spike output. (d) Neurons based on single volatile TS memristors and its voltage input and current output. (e) HH neurons based on two VO2 devices, detailed simulation of biological neuronal cell membrane ion channels with over 30 neural dynamic characteristics. (f) The device used to achieve neuron intrinsic plasticity and the frequency of the output spike of this neuron versus the strength of the presynaptic input signal. (g) FeFET-based LIF neurons with inhibitory synaptic inputs, and their pulse input and output signals. (h) LIF neuron circuit with spiking frequency adaptation, and the output spiking waveform (frequency becomes stable over time). Reproduced with permission from (b) Ref. [122] Copyright 2016 IEEE publishing, (c) Ref. [123] Copyright 2017 IEEE publishing, (d) Ref. [124] Copyright 2018 Wiley-VCH, (e) Ref. [127] Copyright 2018 Springer Nature, (f) Ref. [129] Copyright 2021 IEEE publishing, (g) Ref. [130] Copyright 2019 IEEE publishing, (h) Ref. [131] Copyright 2022 IEEE publishing.](/Images/icon/loading.gif)
Figure 8.(Color online) (a) Schematic diagram of biological neurons. Presynaptic currents are integrated in neurons. When the membrane potential reaches a certain threshold, an action potential is generated. (b) The circuit of LIF neuron. The capacitor acts as the membrane and implements integration effect via the charging process. The resistor is used to limit the current through the VO2-based MIT device, which is the output signal of the neuron. (c) LIF neurons with voltage spike output. (d) Neurons based on single volatile TS memristors and its voltage input and current output. (e) HH neurons based on two VO2 devices, detailed simulation of biological neuronal cell membrane ion channels with over 30 neural dynamic characteristics. (f) The device used to achieve neuron intrinsic plasticity and the frequency of the output spike of this neuron versus the strength of the presynaptic input signal. (g) FeFET-based LIF neurons with inhibitory synaptic inputs, and their pulse input and output signals. (h) LIF neuron circuit with spiking frequency adaptation, and the output spiking waveform (frequency becomes stable over time). Reproduced with permission from (b) Ref. [122] Copyright 2016 IEEE publishing, (c) Ref. [123] Copyright 2017 IEEE publishing, (d) Ref. [124] Copyright 2018 Wiley-VCH, (e) Ref. [127] Copyright 2018 Springer Nature, (f) Ref. [129] Copyright 2021 IEEE publishing, (g) Ref. [130] Copyright 2019 IEEE publishing, (h) Ref. [131] Copyright 2022 IEEE publishing.
Artificial neurons based on CMOS circuits suffer from problems like low integration density and high power consumption[121]. Fortunately, the introduction of volatile memristive devices greatly simplifies the circuits and improves efficiency due to their similar properties with the biological neuron. In 2016, Linet al.[122] demonstrated an LIF neuron using VO2-based MIT device, which is integrated in series with a resistor and in parallel with a capacitor (Fig. 8(b)). The capacitor acted as the membrane and achieved integration effect via the charging process, while the resistor was used to limit the current across the MIT device. When the membrane potential reached the threshold, the neuron would output current spikes. Later, the neurons were modified to achieve voltage spiking output (Fig. 8(c))[123]. To establish neurons with low energy consumption, CF-based TS devices of Ag/FeOx/Pt[124] were employed to construct a compact artificial neuron without peripheral circuits due to its ultra-lowIoff and ultra-narrow pulse tunability (Fig. 8(d)). Recently, Caoet al.[125] proposed Hf0.2Zr0.8O2 anti-ferroelectric film to achieve neurons with low energy consumption (37 fJ/spike) and high reliability (>1012 cycles). The above LIF neurons can achieve at least three basic functions: membrane potential leakage and integration, threshold-excited spiking action potential, and an input-modulated output spike frequency. Nevertheless, they do not characterize in detail the intrinsic properties of biological neurons. Thus, Pickettet al.[126] reported an HH neuron using two NbO2-based devices as ion channels of biological neurons. Subsequently, further optimization was made by Yiet al.[127] where the neuron possessed all three types of excitability and almost all biological neuronal dynamics (Fig. 8(e)). These years, more attention is focused on modifications of the LIF model to achieve more biological neuron functions with lower hardware cost and energy consumption. Liuet al.[128] achieved output frequency adaption (homeostasis function) by connecting an analog memristor under the influence of continuous input pulses in series to the neuron circuit. Later, Weiet al.[129] reported the similar function more reliably using an additional transistor circuit (Fig. 8(f)). With continuous pulse stimulation, the output spike frequency will stabilize at a constant value. Compared to LIF neurons without frequency adaption, the recognition accuracy was improved by 3 percentage points in the simulation based on MNIST dataset. Luoet al.[130] added an inhibitory pulse input by using a transistor as a voltage controlled leaky channel in FeFET-based LIF neurons, as shown inFig. 8(g), resulting in an accuracy of 93% in the network simulation. Most of the above optimization efforts rely on external components, which is clearly detrimental to the circuit area. Wuet al.[131] proposed a combined device, as shown inFig. 8(h). It consisted of a TaOx non-volatile memristor and a VOx volatile threshold switching memristor. By regulating the conductance of TaOx layer, it was possible to modulate the excitation threshold of the neurons. Wanget al.[132] reported a similar scheme where the delay of the output spike relative to the input pulse could be modulated by changing the initial state of the device. It is important to maintain the accuracy of the entire network even when some of the synapse devices suffer from stuck-on failures.
3.2.2. Fully memristive neural network
Artificial neural network arrays based on memristive synapse devices and CMOS neurons have been widely reported[133-136], and even the corresponding chips have been produced[137]. However, in order to achieve high density and high efficiency, fully memristive neural networks are still demanded. In 2018, Wanget al.[138] realized fully memristive neural networks employing volatile and non-volatile memristors, as shown inFig. 9(a). The neuron consisted of an CF-based TS memristor and a capacitor in parallel to implement a simple LIF function. Duanet al.[139] and Liet al.[140] also demonstrated the inference process of small-scale memristive neural networks. These works demonstrated the potential of memristive neural networks for unsupervised or supervised learning and pattern classification compared with CMOS solutions with a large number of transistors, but it also left several questions.
![(Color online) (a) An 8 × 8 artificial neural network integrated by Pt/HfOx/Pd non-volatile memristors and Pt/Ag/SiO2:Ag/Ag/Pt MCF-TS memristors. (b) Schematic of the constructed fully memristive Temporal Coding 256 × 5 SNNs and the hardware implementation. (c) Artificial neuron integrated with dendrite and soma, where Pt/TiOx/Ti volatile memristor is used as dendrite and Pd/NbOx/Nb/Pd MIT device is used to generate action potential. (d) A simple neural network demonstration, using the same device in the synaptic and neuronal parts, and showingI–V sweeping operating in resistive switching mode and threshold switching mode, respectively. Reproduced with permission from (a) Ref. [138] Copyright 2018 Springer Nature, (b) Ref. [143] Copyright 2022 Wiley-VCH, (c) Ref. [141] Copyright 2020 IEEE publishing, (d) Ref. [144] Copyright 2022 Wiley-VCH.](/Images/icon/loading.gif)
Figure 9.(Color online) (a) An 8 × 8 artificial neural network integrated by Pt/HfOx/Pd non-volatile memristors and Pt/Ag/SiO2:Ag/Ag/Pt MCF-TS memristors. (b) Schematic of the constructed fully memristive Temporal Coding 256 × 5 SNNs and the hardware implementation. (c) Artificial neuron integrated with dendrite and soma, where Pt/TiOx/Ti volatile memristor is used as dendrite and Pd/NbOx/Nb/Pd MIT device is used to generate action potential. (d) A simple neural network demonstration, using the same device in the synaptic and neuronal parts, and showingI–V sweeping operating in resistive switching mode and threshold switching mode, respectively. Reproduced with permission from (a) Ref. [138] Copyright 2018 Springer Nature, (b) Ref. [143] Copyright 2022 Wiley-VCH, (c) Ref. [141] Copyright 2020 IEEE publishing, (d) Ref. [144] Copyright 2022 Wiley-VCH.
The first and foremost problem is the power consumption of the hardware network, although volatile TS memristor-based neurons have advantages in terms of area and energy consumption compared to CMOS circuits, facilitating large-scale integration. Zhanget al.[141] exploited the feature that the first output spike of a neuron has different delays under different input signal strength to change the common spiking frequency coding to the first spike arriving time coding. Only the first excitation spike is valid, and all other outputs will be suppressed, which is also consistent with synaptic spiking-time-dependence-plasticity (STDP), as shown inFig. 9(b). Both Wanget al.[142] and Liet al.[143] took the approach of filtering the tiny signals, which means that only the strong enough signals can excite neurons. Among them, Liet al.[143] used TiOx-based volatile memristors as dendrites instead of the common parallel capacitor or parasitic capacitance of neural components to filter out the weaker presynaptic current, as shown inFig. 9(c). The energy consumption is only 5.6% of the GPU while processing the same task. By avoiding the accumulation of unimportant current information on neurons, this scheme not only improves the energy efficiency of the whole neural network, but also improves the recognition accuracy.
Nevertheless, it is worth noting that the above fully memristive neural networks use at least 2 or even more than 2 types of memristors, which inevitably brings some problems such as the interaction of multiple materials and the mismatch of different kinds of devices. Moreover, the above memristive neural networks only demonstrated the inference process of single layer network without afferent neurons, have not addressed the question of whether afferent neurons and interneurons’ output spikes can drive postsynaptic devices. Thus, heterogeneous integrated neural networks using different kinds of devices may have lurking perils in terms of synergy. The brand-new V/HfWOx/Pt memristor proposed by Fuet al.[144], as shown inFig. 9(d), can work in both non-volatile resistive switching mode and volatile threshold switching mode, so it can be used as a synaptic device or to construct artificial neurons. Yuet al.[145] also reported similar homogeneous integration schemes, which effectively solves the mismatch of device parameters and improves the reliability of the entire hardware network. Of course, there are still many problems limiting the development of fully memristive neural networks, including slight changes in the conductance of synapse devices under the influence of continuous output spikes from anterior neurons, and degradation of neuron devices under the continuous stimulation of presynaptic current, etc. Therefore, the road to fully memristive neural networks has a long way to go.
3.2.3. Artificial sensory neuron
The sensory system is an important part of AI, for example, ANN can be used for real-time image processing and pattern classification in the field of autonomous driving, robotics,etc.[146,147]. But traditional AI sensory system relies on complex sensors, signal processing circuits, processors and memory, which means a lot of energy is consumed while processing real-time external information at excessive hardware cost[148]. It’s clearly detrimental to the edge devices of the IoT for real-time information processing.
Fortunately, volatile TS memristor based sensory neurons can encode external information into spikes, which can be directly exploited by SNNs, helping system reduce unnecessary power consumption[22,149-158]. In fact, many sensory spiking neurons, some also called nociceptors, have been currently reported to respond to external stimuli, including mechanical force[156-158], light[152], heat[154], curvature[157], etc. Examples of neurons that can perceive mechanical forces, light and heat are shown in theFigs. 10(a)–10(c). Interestingly, their circuit structure is almost similar to that of the previously reported LIF model spiking neurons. The difference is that the resistor originally connected in series with the neuron is replaced by a tunable resistor that can be adjusted by different stimuli, which will result in different output spiking frequency response from the neuron depending on the voltage division between the sensory resistor and the neuron device under a fixed input voltage amplitude. These works usually require an additional power supply, which has to occupy a certain area and generate static power consumption. Zhanget al.[156] and Songet al.[155] solved this problem in force-sensing neurons and heat-sensing neurons by using piezoelectric nanogenerator and thermocouple, respectively. However, as a simulated human sensory system, sensory neurons at this stage tend to be used as nociceptors,i.e. the spiking responses are available as soon as the stimulus input, which corresponds to the stimulus maladaptation of biological sensory neurons. In fact, humans can adapt to a certain intensity of external stimuli without reacting. Moreover, current visual neurons can only respond to light intensity, and then when it comes to image recognition or spatial perception tasks, neurons that can only process one-dimensional information are more than adequate. Therefore, it is necessary to urgently develop sensory neurons with richer functions, better perception ability and more comprehensive perceptual information.
![(Color online) (a–c) The environmental input signal are mechanical force, light, and heat, respectively. The sensory neurons, and their output signal characteristics are also shown. Essentially, the load resistors of the common LIF neurons are replaced with various types of sensing resistors. (d) A 20 × 20 sensory neuron array for object classification simulation, which shows 8 objects with different shapes and temperatures, having 400 afferent neurons, 50 hidden layer neurons, and 8 output neurons. The final result is a reliable classification. Reproduced with permission from (a) Ref. [154] Copyright 2022 Wiley-VCH, (b) Ref. [152] Copyright 2022 Wiley-VCH, (c) Ref. [157] Copyright 2022 Springer Nature, (d) Ref. [158] Copyright 2022 Wiley-VCH.](/Images/icon/loading.gif)
Figure 10.(Color online) (a–c) The environmental input signal are mechanical force, light, and heat, respectively. The sensory neurons, and their output signal characteristics are also shown. Essentially, the load resistors of the common LIF neurons are replaced with various types of sensing resistors. (d) A 20 × 20 sensory neuron array for object classification simulation, which shows 8 objects with different shapes and temperatures, having 400 afferent neurons, 50 hidden layer neurons, and 8 output neurons. The final result is a reliable classification. Reproduced with permission from (a) Ref. [154] Copyright 2022 Wiley-VCH, (b) Ref. [152] Copyright 2022 Wiley-VCH, (c) Ref. [157] Copyright 2022 Springer Nature, (d) Ref. [158] Copyright 2022 Wiley-VCH.
As a kind of afferent neuron, current works have demonstrated their ability to convert analog signals into SNN-applicable spikes, but relative reporter, which demonstrates sensory neurons with SNN synaptic devices as well as output neurons, is rare. Zhuet al.[158] showed a small in-sensor computing network at the simulation level that allows accurate recognition of the shape, weight, and temperature of objects, as shown inFig. 10(d). However, there is still no hardware level demonstration, which we think is the inevitable trend of future development. While there is certainly the same difficulty of integrating different hardware together as in FHMNNs, some solutions to help the FHMNNs implementation have been proposed in the previous section, which can be a guideline for implementing in-sensor computing at the hardware level.
3.3. Hardware security
With the rapid development of AIoT, massive privacy information and encrypted data is stored and communicated in the edge. How to protect the data with low cost becomes a serious problem. Hardware-intrinsic security primitives such as true random number generator (TRNG) and physical unclonable function (PUF) units can be the solutions, which recently attract great attentions. Many researchers have proposed various devices (SRAM, STT-MRAM, RRAM) to achieve TRANG or PUF. Among them, random raw noises are used as an entropy source, and then a post-processing circuit is combined to generate random signal. These methods suffer from the problem of high energy consumption and large device area, which is not appropriate for the edge devices. The volatile TS memristors feature intrinsic stochasticity, high density and low energy consumption, which have great potential to break the dilemma. Consequently, volatile TS memristor-based TRNG and PUF becomes promising hardware security primitives of the AIoT system and deserve in-depth explorations.
3.3.1. True random number generator
As shown inFig. 11(a), random numbers generated by PRNG are driven by mathematical algorithms. Though the very-long-period pseudo random sequence could be designed, it is still predictable due to the defect of algorithm and the social engineering attack. Instead, TRNGs are driven by random parameters, such as thermal noise, random oscillations,etc. These random parameters are usually derived from the physical mechanism of the device, or the oscillator. It produces an unpredictable and fully random sequence.
![(Color online) (a) Schematic illustration of TRNG and its difference with PRNG. (b) OTS-based TRNG using the Weibull distribution of on-time to generate random bits. (c) One of the mainstream TRNG structures containing comparator, AND, clock and counter. (d) NbOx-based TRNG circuits. (e) The circuit design of a recent reported TRNG based on CF-TS devices. (f) 15 excitation pulses are applied to the diffusive memristor (V1). The stochastic fire spikes (V2) are sent to a comparator and will induce the random number of voltage pulses at node 3 (V3). V3 will be then sent to a one-bit counter as the clock signal. The output of the one-bit counter represents the odd or even of the fire spikes. “1” and “0” represent that the numbers of fire spikes are ODD and EVEN, respectively. (g) Demonstration of the basic conception of PUF. (h) Hardware implementation of the PUF. (i) Read-conductance of each device at 0.5 V in the array. (j) Operation principle of the PUF in the array. (k) The uniqueness (inter-PUF) and reliability (intra-PUF) performances of the TS-based PUF. Reproduced with permission from (b) Ref. [159] Copyright 2019 IEEE publishing, (c) Ref. [160] Copyright 2017 Springer Nature, (d) Ref. [163] Copyright 2021 Springer Nature, (e, f) Ref. [81] Copyright 2022 Wiley-VCH, (h–k) Ref. [164] Copyright 2021 IEEE publishing.](/Images/icon/loading.gif)
Figure 11.(Color online) (a) Schematic illustration of TRNG and its difference with PRNG. (b) OTS-based TRNG using the Weibull distribution of on-time to generate random bits. (c) One of the mainstream TRNG structures containing comparator, AND, clock and counter. (d) NbOx-based TRNG circuits. (e) The circuit design of a recent reported TRNG based on CF-TS devices. (f) 15 excitation pulses are applied to the diffusive memristor (V1). The stochastic fire spikes (V2) are sent to a comparator and will induce the random number of voltage pulses at node 3 (V3). V3 will be then sent to a one-bit counter as the clock signal. The output of the one-bit counter represents the odd or even of the fire spikes. “1” and “0” represent that the numbers of fire spikes are ODD and EVEN, respectively. (g) Demonstration of the basic conception of PUF. (h) Hardware implementation of the PUF. (i) Read-conductance of each device at 0.5 V in the array. (j) Operation principle of the PUF in the array. (k) The uniqueness (inter-PUF) and reliability (intra-PUF) performances of the TS-based PUF. Reproduced with permission from (b) Ref. [159] Copyright 2019 IEEE publishing, (c) Ref. [160] Copyright 2017 Springer Nature, (d) Ref. [163] Copyright 2021 Springer Nature, (e, f) Ref. [81] Copyright 2022 Wiley-VCH, (h–k) Ref. [164] Copyright 2021 IEEE publishing.
The inherent variability in threshold voltage results in a bimodal distribution of on/off states which can be easily converted into digital bits. Chaiet al.[159] found that the GeSe-based OTS device had an equal probability in on or off states when the amplitude and width of the applied voltage pulse was 2.7 V and 1μs, respectively (Fig. 11(b)). Although a random bit stream of 0 and 1 can be generated, the TRNG approach owns few disadvantages. The method greatly depends on the exact distribution of the measured parameters (Vth or ton), and the set threshold values (amplitude and width of pulse) must be based on amounts of statistical data, which easily induce inaccuracy if the sample size is not big enough. In addition, the poor D2D uniformity makes the determination of threshold values hard and leads to inaccuracy. To avoid the insufficiency, Jianget al.[160] introduced the voltage-to-time conversion scheme and used switching time of diffusive memristors as entropy sources in their work (Fig. 11(c)). After that, the TRNG containing flip-flops or counters has gradually become one of the mainstream structures[161,162]. Although the accuracy problem is solved, the generated random bit rate is too low to match with the computer system. To increase the throughput of TRNG, Kimet al.[163] reported a self-clocking TRNG based on the MIT device, as shown inFig. 11(d). The randomness was processed by the T flip-flop and output as binary bits. The throughput was improved to 40 kb/s. Recently, Luet al.[81] designed a TRNG based on Ag/TiN/HfOx/HfOy/HfOx/Pt CF-TS device (Fig. 11(e)). The comparator sent a high-level output to the 1-bit counter when the voltage of firing spikes exceeded the reference value (Fig. 11(f)), and then the 1-bit counter flipped, which converted the number of even or odd firing spikes to random binary bits. The throughput was enhanced to 108 kb/s and passed the standard statistical test package developed by the National Institute of Standards and Technology (NIST). The entropy source, throughput, energy consumption, endurance, and NIST test of the TS-based TRNG works are listed inTable 2.
Table Infomation Is Not Enable
The three types of TS devices have different switching mechanisms, which make their own advantages and disadvantages in throughput, energy consumption, and endurance. Due to ultralow leakage current, CF-based TS devices are good at energy consumption control, while their throughput and endurance are limited by the slow switching speed and poor cycle endurance. In contrast, MIT devices have faster switching speed, which result in faster throughput. However, the thermal and phase instability of MIT materials (VOx and NbOx) block their endurance and reliability. As for OTS devices, they have relatively better switching speed and endurance, but bad power consumption control. There are some suggestions for TRNG performance enhancements from device perspectives. Firstly, if the application has higher requirements on the throughput, and attention should be paid to the switching time of the device, and the swing of the oscillation voltage (threshold voltage - hold voltage), which are negatively correlated with throughput, and thus, OTS and MIT devices will be more suitable. Secondly, when energy consumption is the crucial indicator of the application, CF-based TS devices are recommended for TRNG design. Meanwhile, the leakage current and operation current are also the main parameters to be considered, which are benefited to the control of energy consumption. Lastly, if the endurance is significant in the application, OTS and MIT devices are suggested to be used in design. And the pulse endurance of the device is very important, which is positively relevant to the TRNG endurance.
Considering the serial structure of the entropy source and post-processing circuit of the TS-based TRNG, there are also several details worth attention in terms of circuit design. The entropy source generation circuit is usually composed of TS devices, while the post-processing circuit is usually composed of CMOS-based digital or analog chips. Therefore, the speed and endurance bottlenecks of TS device-based TRNG mainly lie in the entropy source generation circuit, while the energy consumption limitation primarily lies in the post-processing circuit. Firstly, the throughput of the TRNG could be promoted by increasing the rate of operations that stimulate entropy source or by increasing the number of random bits generated per operation. Secondly, endurance could also be improved by increasing the number of random bits generated per operation. Lastly, if designers want to reduce the overall energy consumption of the TRNG, it is recommended to choose low-power chips or to reduce the size of the post-processing circuit. In summary, not any type of TS device is almighty for TRNG design. Different types of TS devices can bring different performance advantages such as low energy consumption, high throughput, and high durability in TRNG. Designers need to select devices according to actual application scenarios. In the future, performance breakthroughs of TS devices are also expected to push the developments of TRNG.
3.3.2. Physical unclonable function
For the information security, the digital data is protected through confidentiality and authentication technologies. The TRNG can work as a random key generator for the authentication, but these keys still need to be stored in digital memory, which could be dangerous. The PUF can solve the problem to act as both key generator and key storage. The basic concept of PUF is illustrated inFig. 11(g). It takes advantage of the unavoidable fluctuation during the manufacturing process, which means there will not be two identical devices. In other words, a PUF is the fingerprint of a physic object. Mathematically, the configurable part of the PUF is generally calledchallenge, which can also regard as the input. The particular measurement of the physical state under a challenge is calledresponse. When a challenge is imposed on the PUF, each unit of the PUF will give a sub-response to the challenge. As the challenge changes, so does the response. There are slight technique differences in the manufacturing process, which makes it challenging to observe the topography differences of the device and reproduce the differences, and generate random fluctuations in the device performance. Even if fabricated with the same process parameters, it is almost impossible to manufacture a device with identical performance. Therefore, identifiability and physical unclonability are two core properties to be satisfied in PUF units.
Recently, PUF units based on volatile TS devices attract great attention due to the intrinsic stochasticity that the device can randomly switch to LRS or stay at HRS under a certain voltage pulse. Dinget al.[164] reported a PUF based on the MIT device, where the hardware system contained TS device array and peripheral circuits, as shown inFig. 11(h).Fig. 11(i) exhibited a random distribution of the read-conductance at 0.5 V in a 32 × 32 array. According to the PUF operating principle inFig. 11(j), the selecting signal of row-column pair is the challenge, and the current on each selected wire is the sum of main cell leakage, non-linearity, and sneak current through half-selected or unselected cells. Due to the random distribution of conductance, the respective sums of the read currents of the two columns were not equal. By comparing the current magnitudes of two selected columns with a comparator, a response corresponding to the challenge could be obtained. As shown inFig. 11(k), the mean value of inter-die and intra-die Hamming distance is 0.49971 and 0.00039 respectively, which is close to the ideal value and the uniqueness of proposed PUF was verified.
There is a corresponding relationship between the performances of the PUF, such as uniformity, uniqueness, reliability, endurance, and strength, and the performances of TS devices, such as randomness of entropy source, C2C and D2D variation, endurance, and scale of the array. However, there are several problems to be solved for the TS-based PUF. Firstly, the array scale is still small, which makes challenge-response pairs (CRPs) too few to guarantee security. Secondly, due to the limited endurance of the TS device, it is hard to store the key for a long time. It might induce the response generated at the beginning to be different from that after several operations, which means it cannot be reproduced. Hence, improving the stability of the TS device and the scale of the TS array will be of significance for TS-based PUF applications.
3.4. Other applications
3.4.1. Steep subthreshold slope transistor
Metal oxide semiconductor field-effect-transistor (MOSFET) is an important type of electronic device in the large-scale integrated circuit industry. The power consumption becomes one of the main challenges as the dimensions scale down. To achieve high energy-efficiency in MOSFET, the transistor should have a steep subthreshold slope (SS = dVg/dlogId,i.e. the gate voltage required to increase the drain current by ten times in sub-threshold regime, in the unit of mV/decade), low leakage current, and low working voltage. However, the sub-threshold slope of conventional MOSFETs is limited to 60 mV/decade due to the thermionic carrier injection mechanism. The lowest limit of SS value has caused to exponentially increase the off-state leakage current with monotonically decreasing the threshold voltage, and thereby, it is difficult to control the passive power consumption in integrated circuit and it also influence the further decrease of operation voltages. Several solutions have been proposed in the material and device community, including impact ionization MOSFET based on avalanche breakdown, tunnel FET based on band-to-band tunneling, hyper-FET based on the metal-to-insulator transition in VO2[165-168]. Nevertheless, these methods either cannot achieve an ultralow subthreshold slope or demand high drain voltages. As listed inTable 1, the CF-based TS devices possess ultra-low leakage current and steep switching slope less than 1 mV/decade. Consequently, the configuration of CF-based TS device in series with the drain of the MOSFET is suggested to construct steep subthreshold slope transistors. Songet al.[169] connected a AgTe/TiO2-based TS device in the series with the drain of a transistor in 2016 and the SS value of 5 mV/decade was obtained. The leakage current was also at a small value ~1 pA. After that, some other TS devices used for steep subthreshold slope transistor were reported successively, such as W/Cu2S/W[170], Ag/HfO2/SiO2/p-Si[171], Ag/Ti/HfO2/Pt[172]. However, all the above TS-based transistors exhibit the subthreshold slope at the level of ~5 mV/decade. It is still an urgent demand to further develop transistors with an ultra-steep subthreshold slope.
3.4.2. volatile memristor as logic device
Boole logic is part of the basic of digital circuit and the device realization of it is an important issue in electronics. In literature, non-volatile memristor devices are always used for Boole logic application. A recent study by Parket al.[173] revealed that the TS devices also could be used for realization of Bool logic. They fabricated Ag/HfOx:N/Pt/Ti device to accomplish the AND and OR logic in a 2 × 2 array. The inputs are the two separated voltages applied on the two TS devices, and the output is the voltage measured at the shared top electrode of the devices. The state “1” and state “0” represent that the voltage is 0.35 and 0 V separately. For AND operation, only if the input of the two devices are both “1” (0.35 V), will the output be state “1” (0.35 V). Unfortunately, only the functions of AND and OR logic were accomplished in this work. Realization of all the sixteen Boole logic function with TS devices is to be continued.
4. Conclusions and perspectives
Volatile threshold switching memristors play an import role in AIoT systems, enabling the entire processes of data and information collection, storage, analysis and communications. In the review, three types of TS devices are discussed in details from the views of materials, switching mechanisms and applications. The distinctive characteristics of CF-based TS, OTS and MIT devices are summarized inFig. 12 including on/off ratio, leakage current (Ioff), on-state current (Ion), threshold voltage (Vth), switching speed, uniformity (σ/μ), and endurance. The CF-based TS devices present excellent on/off ratio, smallVth, and ultra-low leakage current, while OTS and MIT devices exhibit good switching speed and on-current. However, the performance enhancements are still urgently demanded for the commercialization of TS devices. The suggestions are shown below.
![(color online) Selected performance of three types of TS devices. The selected values are the maximum or minimum values of the performances in Table 1 from Refs. [75,84,86,90,93,104,116,181,183,188,189]. Switching energy is roughly estimated from voltage pulse measurements,E = VIt whereV andt are the amplitude and width of the applied voltage pulse, respectively,I is the current response to the pulse[27].](/Images/icon/loading.gif)
Figure 12.(color online) Selected performance of three types of TS devices. The selected values are the maximum or minimum values of the performances in Table 1 from Refs. [75,84,86,90,93,104,116,181,183,188,189]. Switching energy is roughly estimated from voltage pulse measurements,E = VIt whereV andt are the amplitude and width of the applied voltage pulse, respectively,I is the current response to the pulse[27].
(1)On-off ratio. On/off ratio enhancement includes the reduction of leakage current and the increase of on-current. CF-based TS devices have a high on/off ratio because of the ultra-low leakage current (even at the level of 0.01pA), which results from the highly insulating properties of dielectrics when the filament is dissolved. In order to fulfill the requirements of selectors in cross-point 1S1R memory technology, the on-current should reach at least 1 mA. The main idea for on-current enhancement is to guide the formation of multiple weak filaments at the high compliance current. There are various strategies from the aspects of active electrode, interface and dielectrics. Changing the active electrode structure (for example, nanodots/nanotips) or material component (for example, alloys or compounds) instead of active metal thin films can control and modulate the strength of filaments. Employment of multi-layer (bilayer or trilayer) dielectrics can constrain the filament formation in the specific switching layer. In addition, defective graphene film with nanoscale holes at the interface between active metal electrode and dielectrics work as atom filter and constrain the position and size of the filaments, which greatly raises the on-current. As for OTS and MIT devices, small on/off ratio and high leakage current are the main challenges for the selector applications. After electroforming (soft breakdown) process, the resistance of dielectric becomes lower than the initial state and cannot recover. Therefore, a more insulating dielectric (also known as electron barrier), for example, Al2O3, is always added in the switching layer to reduce the leakage current.
(2)Switching speed. The switching speed includes both the delay time of turn-on and relaxation time of turn-off under the voltage pulses. The high switching speed of the OTS and MIT devices is induced by the extremely fast crystallization rate and fast phase transition, respectively. But the mobility of the active metal ions in the dielectrics limits the switching speed, especially the turn-off speed of CF-based TS devices. To accelerate the spontaneous rupture process, some effective methods are proposed. The relaxation time has linear dependence on the amplitude and width of the applied voltage pulse. In addition, it is also related to the nucleation barrier energyW0 of the dielectric materials from the view of nucleation theory. Since theW0 value is related to surface tension, searching for materials possessing high interface energy against metallic filaments would be the key to modulate relaxation time.
(3)Uniformity. All the three types of TS devices have poor uniformity and large variation of operation parameters (for example,Vth,Ioff) due to the stochastic atomic arrangements during the processes of filament formation and rupture. The intrinsic stochasticity of TS devices is good for some specific applications. For example, the requirement of the random entropy source makes TS devices the perfect unit for the security application like TRNG and PUF. However, the stochasticity has a negative effect on selector and neuromorphic devices. CF-based TS devices show large variation ofVth value (σ/μ, standard deviation divided by average value) even up to 20%. To reduce the stochastic formation and rupture of filaments, the main solution is to restrict the filament formation in specific positions from the view of electrode and dielectric modification. The variation of 20% could be reduced to less than 5%, but the employed methods are not compatible with CMOS and memory devices, for example, chemical synthesis of Ag nanoparticles.
(4)Endurance. The reliable characterization of endurance is the first issue to be solved. Lanzaet al.[174] claim that most endurance tests based on resistanceversus cycle plots contain very few data points (even<20), which is highly inaccurate and unreliable because it cannot reliably demonstrate that the device effectively switches in every cycle and it ignores C2C and D2D variability. They constructed endurance plots showing one data point per cycle and resistive state and combined data from multiple devices. According to the characterization rule, the endurance of MIT and OTS devices is usually less than 1010 cycles, while CF-based TS devices show relatively poor endurance less than 108 cycles due to the excessive injection of active metal ions after several operations leading to the stuck-on issues. Diffusion barrier layers such as TiN are always used to inhibit the excessive injection. In addition, alloy or compound electrodes are also proved to be effective for endurance enhancement. High endurance ensures that the response of the devices after repetitive operations can still be consistent, which is valuable for the security applications and large memory array.
For the practical implementation of memristors-based system, the devices would be required for mass production using mainstream manufacturing technology. Most of the present peripheral circuits/components for neuromorphic computing and hardware security are based on the current CMOS technology. Realizing the excellent integration and compatibility between TS and CMOS devices/techniques is very important. The above materials and methods have been reported for improving performances of TS devices. However, some of the methods are not compatible with the CMOS technology, which would not be accepted by the IC industry. Apart from device optimization, there is no uniform theory on switching mechanisms of TS devices due to the lack of effective characterizations.In-situ HRTEM characterization and first principles calculation are helpful to observe and understand the evolution of metallic conductive filaments. However, when the filament composition becomes complex especially with various performance optimization schemes, the traditional evolution model of Ag or Cu conductive filaments is obviously insufficient to meet the demand. For example, multi-metal (CuAg) filaments and oxygen vacancy-metal coupling filaments (Vo-Ag) lack effective observation methods and theoretical models. With a combination of materials and physics theories, breakthrough can be carried out from the views of electronic structure and crystal structure of materials. Therefore, we should further investigate the switching mechanisms of TS devices and find proper materials or methods that are suitable for commercial applications.
At last, the research of TS devices cannot be separated from the basic requirements of AIoT applications, including data and information collection, storage, processing and communication. To solve the sneak current problem, an ideal selector should be scalable and stackable with high nonlinearity, low leakage current (~pA), high on-current (>100μA), fast switching speed (~ns), excellent endurance (>1010), and good uniformity. The selectors reported in recent years are only prominent in some aspects even in the single device. To realize the large-scale memristive storage array, in-depth studies on the material system and mechanisms of TS-based selectors are indispensable. For artificial neurons, a simple circuit design, which can exhibit superior performance without capacitor, is desired for high density and efficiency. Employment of the parasitic capacitance of two-terminal TS devices could be a good scheme, but operation voltage, endurance and uniformity should be paid more attention for device performance enhancements. As for TRNG or PUF applications, the device optimization should be aimed at high-frequency operation (>1 GHz), high endurance, low energy consumption for each random bit (~fJ range), and small device area (~nm range). At the circuit level, smaller area occupation and simple design are more desirable. Finally, novel multi-functional applications are expected in future, which should be closely based on the feature of spontaneous decay and stochasticity. We are looking forward that volatile TS devices occupying a crucial role in the AIoT era.
References
[1] R Morteza, S Maryam, H K Mostafa. Fog-based smart homes: A systematic review. J Netw Comput Appl, 153, 102531(2020).
[2] J Jose, A Peralta, W Christian et al. A systematic survey of internet of things frameworks for smart city applications. Sustain Cities Soc, 83, 103949(2022).
[4] Z Zhang, F Wen, Z Sun et al. Artificial intelligence-enabled sensing technologies in the 5G/internet of things era: from virtual reality/augmented reality to the digital twin. Adv Intell Syst, 4, 2100228(2022).
[5] J Li, G Yang. Network embedding enhanced intelligent recommendation for online social networks. Future Gener Comput Syst, 119, 68(2021).
[6] C Yang, H Chen, E Chang et al. Current advances and future challenges of AIoT applications in particulate matters (PM) monitoring and control. J Hazard Mater, 419, 126442(2021).
[7] Y Liao, N Yu, G Zhou et al. A wireless multi-channel low-cost lab-on-chip algae culture monitor AIoT system for algae farm. Comput Electron Agr, 193, 106647(2022).
[8] D Caleb, C Albert, D Amos. Artificial intelligence in green building. Automat Constr, 137, 104192(2022).
[9] M C Chiu, W M Yan, A B Showkat et al. Development of smart aquaculture farm management system using IoT and AI-based surrogate models. J Agr Food Res, 9, 100357(2022).
[10] D Jiang, G Li, C Tan et al. Semantic segmentation for multiscale target based on object recognition using the improved Faster-RCNN model. Future Gener Comput Syst, 123, 94(2021).
[12] J Zhu, T Zhang, Y Yang et al. A comprehensive review on emerging artificial neuromorphic devices. Appl Phys Rev, 7, 011312(2020).
[13] F Alqahtani, Z Al-Makhadmeh, A Tolba et al. TBM: A trust-based monitoring security scheme to improve the service authentication in the Internet of Things communications. Comput Commun, 150, 216(2020).
[14] L O Chua. Memristor-the missing circuit element. IEEE Trans Circuit Theory, 18, 507(1971).
[15] J Ge, S Zhang, Z Liu et al. Flexible artificial nociceptor using a biopolymer-based forming-free memristor. Nanoscale, 11, 6591(2019).
[16] M Xu, X Mai, J Lin et al. Recent advances on neuromorphic devices based on chalcogenide phase-change materials. Adv Funct Mater, 30, 2003419(2020).
[17] R Carboni, D Ielmini. Stochastic memory devices for security and computing. Adv Electron Mater, 5, 1900198(2019).
[18] G Rajendran, W Banerjee, C Anupam. Application of resistive random-access memory in hardware security: A review. Adv Electron Mater, 7, 2100536(2021).
[19] R Aluguri, T Y Tseng. Memory technologies devices for 3D stackable cross point RRAM arrays. J Electron Dev Soc, 4, 294(2016).
[20] R Wang, J Yang, J Mao et al. Recent advances of volatile memristors: devices, mechanisms, and applications. Adv Intell Syst, 2, 2000055(2020).
[21] W Chen, H J Barnaby, M N Kozicki. Volatile and non-volatile switching in Cu-SiO2 programmable metallization cells. IEEE Electron Dev Lett, 37, 580(2016).
[22] J H Yoon, Z Wang, K M Kim et al. An artificial nociceptor based on a diffusive memristor. Nat Commun, 9, 417(2018).
[23] X Zhao, R Wang, X Xiao et al. Flexible cation-based threshold selector for resistive switching memory integration. Sci China Inform Sci, 61, 060413(2018).
[24] Y Park, U B Han, M K Kim et al. Solution-processed flexible threshold switch devices. Adv Electron Mater, 4, 1700521(2018).
[25] S Saitoh, K Kinoshita. Oxide-based selector with trap-filling-controlled threshold switching. Appl Phys Lett, 116, 112101(2020).
[26] C Sung, S Lim, H Hwang. Experimental determination of the tunable threshold voltage characteristics in a AgxTe1–x/Al2O3/TiO2-based hybrid memory device. IEEE Electron Dev Lett, 41, 713(2020).
[27] Y F Lu, H Li, T Wan et al. Low-power artificial neurons based on Ag/TiN/HfAlOx/Pt threshold switching memristor for neuromorphic computing. IEEE Electron Dev Lett, 41, 1245(2020).
[28] H Li, X Huang, J Yuan et al. Controlled memory and threshold switching behaviors in a heterogeneous memristor for neuromorphic computing. Adv Electron Mater, 6, 2000309(2020).
[29] M Kumar, H S Kim, J Kim. A highly transparent artificial photonic nociceptor. Adv Mater, 31, 1900021(2019).
[30] Y Shi, X Liang, B Yuan et al. Electronic synapses made of layered two-dimensional materials. Nat Electron, 1, 458(2018).
[31] M Wang, S Cai, C Pan et al. Robust memristors based on layered two-dimensional materials. Nat Electron, 1, 130(2018).
[32] D Dev, A Krishnaprasad, M S Shawkat et al. 2D MoS2-based threshold switching memristor for artificial neuron. IEEE Electron Dev Lett, 41, 936(2020).
[33] J Yang, R Wang, Z Wang et al. Leaky integrate-and-fire neurons based on perovskite memristor for spiking neural networks. Nano Energy, 74, 104828(2020).
[34] L Wang, W Cai, D He et al. Performance improvement of GeTex-based Ovonic threshold switching selector by C doping. IEEE Electron Dev Lett, 42, 688(2021).
[35] E Ambrosi, C Wu, H Y Lee et al. Reliable low voltage selector device technology based on robust SiNGeCTe arsenic-free chalcogenide. IEEE Electron Dev Lett, 43, 1673(2022).
[36] J Kim, M Kang, W Kim et al. Impact of Ag doping on subthreshold conduction in amorphous Ga2Te3 with threshold switching. J Alloy Comp, 913, 165176(2022).
[37] J Bian, Y Tao, Z Wang et al. A stacked memristive device enabling both analog and threshold switching behaviors for artificial leaky integrate and fire neuron. IEEE Electron Dev Lett, 43, 1436(2022).
[38] H Huang, R Yang, Z H Tan et al. Quasi-Hodgkin-Huxley Neurons with leaky integrate-and-fire functions physically realized with memristive devices. Adv Mater, 31, 1803849(2019).
[39] C Zhang, J Shang, W Xue et al. Convertible resistive switching characteristics between memory switching and threshold switching in a single ferritin-based memristor. Chem Commun, 52, 4828(2016).
[40] D Kim, B Jeon, Y Lee et al. Prospects and applications of volatile memristors. Appl Phys Lett, 121, 010501(2022).
[41] Y Sun, C Song, S Yin et al. Design of a controllable redox-diffusive threshold switching memristor. Adv Electron Mater, 6, 2000695(2020).
[42] Y Yang, P Gao, S Gaba et al. Observation of conducting filament growth in nanoscale resistive memories. Nat Commun, 3, 732(2012).
[43] Y Yang, P Gao, L Li et al. Electrochemical dynamics of nanoscale metallic inclusions in dielectrics. Nat Commun, 5, 4232(2014).
[44] J Yoo, J Park, J Song et al. Field-induced nucleation in threshold switching characteristics of electrochemical metallization devices. Appl Phys Lett, 111, 063109(2017).
[45] Z Wang, S Joshi, E S Sergey et al. Memristors with diffusive dynamics as synaptic emulators for neuromorphic computing. Nat Mater, 16, 101(2017).
[46] S Oh, S Lee, H Hwang. Improved turn-off speed and uniformity of atomic threshold switch device by AgSe electrode and bipolar pulse forming. J Electron Dev Soc, 9, 864(2021).
[47] F Ye, F Kiani, Y Huang et al. Diffusive memristors with unifor and tunable relaxation time for spike generation in event-based pattern recognition. Adv Mater, 34, 2204778(2022).
[48] S A Chekol, S Menzel, R W Ahmad et al. Effect of the threshold kinetics on the filament relaxation behaviour of Ag-based diffusive memristors. Adv Funct Mater, 32, 2111242(2022).
[49] A M Stoneham. Systematics of metal-insulator interfacial energies: A new rule for wetting and strong catalyst-support interactions. Application Surf Sci, 14, 249(1983).
[50] J Song, J Woo, A Prakash et al. Threshold selector with high selectivity and steep slope for cross-point memory array. IEEE Electron Dev Lett, 36, 681(2015).
[51] S Ambrogio, S Balatti, S Choi et al. Impact of the Mechanical stress on switching characteristics of electrochemical resistive memory. Adv Mater, 26, 3885(2014).
[52] S R Ovshinsky. Reversible electrical switching phenomena in disordered structures. Phys Rev Lett, 21, 1450(1968).
[53] M Nardone, V G Karpov, D C S Jackson et al. A unified model of nucleation switching. Appl Phys Lett, 94, 103509(2009).
[54] W Zhang, R Mazzarello, M Wuttig et al. Designing crystallization in phase-change materials for universal memory and neuro-inspired computing. Nat Rev Mater, 4, 150(2019).
[55] D Ielmini, A L Lacaita, D Mantegazza. Recovery and drift dynamics of resistance and threshold voltages in phase-change memories. IEEE Transaction Electron Dev, 54, 308(2007).
[56] Y R Guo, F Dong, C Qiao et al. Structural signature and transition dynamics of Sb2Te3 melt upon fast cooling. Phys Chem Chem Phys, 20, 11768(2018).
[57] J Y Raty, P Noé. Ovonic threshold switching in Se-rich GexSe1−x glasses from an atomistic point of view: The crucial role of the metavalent bonding mechanism. Phys Status Solidi-R, 14, 1900581(2020).
[58] M Wuttig, V L Deringer, X Gonze et al. Incipient metals: functional materials with a unique bonding mechanism. Adv Mater, 30, 1803777(2018).
[59] M Zhu, O Cojocaru-Mirédin, A M Mio et al. Unique bond breaking in crystalline phase change materials and the quest for metavalent bonding. Adv Mater, 30, 1706735(2018).
[60] M Imada, A Fujimori, Y Tokura. Metal-insulator transitions. Rev Modern Phys, 70, 1039(1998).
[61] R M Wentzcovitch, W W Schulz, P B Allen. VO2: Peierls or Mott-Hubbard? A view from band theory. Phys Rev Lett, 72, 3389(1994).
[62] T M Rice, H Launois, J P Pouget. Comment on "VO2: Peierls or Mott-Hubbard? A view from band theory". Phys Rev Lett, 73, 3042(1994).
[63] W W Schulz, R M Wentzcovitch. Electronic band structure and bonding in Nb3O3. Phys Rev B, 48, 16986(1993).
[64] D Lee, B Chung, Y Shi et al. Isostructural metal-insulator transition in VO2. Science, 362, 1037(2018).
[65] V R Morrison, R P Chatelain, K L Tiwari et al. A photoinduced metal-like phase of monoclinic VO2 revealed by ultrafast electron diffraction. Science, 346, 445(2014).
[66] S Biermann, A Poteryaev, A I Lichtenstein et al. Dynamical singlets and correlation-assisted Peierls transition in VO2. Phys Rev Lett, 94, 026404(2005).
[67] M W Haverkort, Z Hu, A Tanaka et al. Orbital-assisted metal-insulator transition in VO2. Phys Rev Lett, 95, 196404(2005).
[68] A Zimmers, L Aigouy, M Mortier et al. Role of thermal heating on the voltage induced insulator-metal transition in VO2. Phys Rev Lett, 110, 056601(2013).
[69] S Cheng, M Lee, X Li et al. Operando characterization of conductive filaments during resistive switching in Mott VO2. Pro National Acad Sci USA, 118, e2013676118(2021).
[70] S Kumar, Z Wang, N Davila et al. Physical origins of current and temperature controlled negative differential resistances in NbO2. Nat Commun, 8, 658(2017).
[71] Y Kalcheim, A Camjayi, J D Valle et al. Non-thermal resistive switching in Mott insulator nanowires. Nat Commun, 11, 2985(2020).
[72] J D Valle, N M Vargas, R Rocco et al. Spatiotemporal characterization of the field-induced insulator-to-metal transition. Science, 373, 907(2021).
[73] C Li, M Hu, Y Li et al. Analogue signal and image processing with large memristor crossbars. Nat electron, 1, 52-59(2018).
[74] X Li, H Wu, B Gao et al. Electrode-induced digital-to-analog resistive switching in TaOx-based RRAM devices. Nanotechnology, 27, 305201(2016).
[75] R Midya, Z Wang, J Zhang et al. Anatomy of Ag/Hafnia-based selectors with 1010 nonlinearity. Adv Mater, 29, 1604457(2017).
[76] Q Hua, H Wu, B Gao et al. A threshold switching selector based on highly ordered Ag nanodots for X-point memory applications. Adv Sci, 6, 1900024(2019).
[77] Y Li, J Tang, B Gao et al. High-uniformity threshold switching HfO2-based selectors with patterned Ag nanodots. Adv Sci, 7, 2002251(2020).
[78] L Goux, K Opsomer, R Degraeve et al. Influence of the Cu-Te composition and microstructure on the resistive switching of Cu-Te/Al2O3/Si cells. Appl Phy Letts, 99, 053502(2011).
[79] K S Woo, J Kim, J Han et al. A high-speed true random number generator based on a CuxTe1−x diffusive memristor. Adv Intelligent Syst, 3, 2100062(2021).
[80] W Banerjee, I V Karpov, A Agrawal et al. Highly-stable (<3% fluctuation) Ag-based threshold switch with extreme-low OFF current of 0.1 pA, extreme-high selectivity of 109 and high endurance of 109 cycles. IEEE International Electron Devices Meeting, 28.4.1(2020).
[81] T Q Wan, Y F Lu, J H Yuan et al. 12.7 MA/cm2 on-current density and high uniformity realized in AgGeSe/Al2O3 selectors. IEEE Electron Dev Lett, 42, 613(2021).
[82] B Grisafe, M Jerry, J A Smith et al. Performance enhancement of Ag/HfO2 metal ion threshold switch cross-point selectors. IEEE Electron Dev Lett, 40, 1602(2019).
[83] X Zhao, J Ma, X Xiao et al. Breaking the current-retention dilemma in cation-based resistive switching devices utilizing graphene with controlled defects. Adv mater, 30, 1705193(2018).
[84] A Sahota, H S Kim, J Mohan et al. Highly reliable selection behavior with controlled Ag doping of nano-polycrystalline ZnO Layer for 3D X-Point framework. IEEE Electron Dev Lett, 43, 21(2021).
[85] Q Luo, X Xu, H Liu et al. Cu BEOL compatible selector with high selectivity (> 107), extremely low off-current (~pA) and high endurance (>1010). IEEE International Electron Devices Meeting, 10.4. 1.(2015).
[86] Y F Lu, H Y Li, Y Li et al. A High-performance Ag/TiN/HfOx/HfOy/HfOx/Pt diffusive memristor for calibration-free true random number generator. Adv Electron Mater, 2200202(2022).
[87] J Yin, F Zeng, Q Wan et al. Self-modulating interfacial cation migration induced threshold switching in bilayer oxide memristive device. J Phys Chem C, 123, 878(2018).
[88] W Czubatyj, S J Hudgens. Thin-film Ovonic threshold switch: Its operation and application in modern integrated circuits. Electron Mater Lett, 8, 157(2012).
[89] M Zhu, K Ren, Z Song. Ovonic threshold switching selectors for three-dimensional stackable phase-change memory. MRS Bull, 44, 715(2019).
[90] B Govoreanu, G L Donadio, K Opsomer et al. Thermally stable integrated Se-based OTS selectors with >20 MA/cm2 current drive, > 3.103 half-bias nonlinearity, tunable threshold voltage and excellent endurance. Symposium on VLSI Technology, T92(2017).
[91] G Navarro, A Verdy, N Castellani et al. Innovative PCM+OTS device with high sub-threshold non-linearity for non-switching reading operations and higher endurance performance. Symposium on VLSI Technology, T94(2017).
[92] N S Avasarala, G L Donadio, T Witters et al. Half-threshold biasIoff reduction down to nA range of thermally and electrically stable high-performance integrated OTS selector obtained by Se enrichment and N-doping of thin GeSe layers. IEEE Symposium on VLSI Technology, 209(2018).
[93] G Liu, T Li, L Wu et al. Increasing trapped carrier density in nanoscale GeSeAs Films by As ion Implantation for selector devices in 3D-Stacking Memory. ACS Appl Nano Mater, 2, 5373(2019).
[94] A Verdy, G Navarro, M Bernard et al. Carbon electrode for Ge-Se-Sb based OTS selector for ultra low leakage current and outstanding endurance. IEEE International Reliability Physics Symposium, 6D.4-1(2018).
[95] A Verdy, M Bernard, J Garrione et al. Optimized reading window for crossbar arrays thanks to Ge-Se-Sb-N-based OTS selectors. IEEE International Electron Devices Meeting, 37.4.1(2018).
[96] M C Cyrille, A Verdy, G Navarro et al. OTS selector devices: Material engineering for switching performance. International Conference on IC Design & Technology, 113(2018).
[97] M Anbarasu, M Wimmer, G Bruns et al. Nanosecond threshold switching of GeTe6 cells and their potential as selector devices. Appl Phy Lett, 100, 143505(2012).
[98] A Verdy, M Bernard, N Castellani et al. Tunable performances in OTS selectors thanks to Ge3Se7-As2Te3. IEEE 11th International Memory Workshop, 1(2019).
[99] M J Lee, D Lee, S H Cho et al. A plasma-treated chalcogenide switch device for stackable scalable 3D nanoscale memory. Nat Commun, 4, 1(2013).
[100] H Y Cheng, W C Chien, I T Kuo et al. An ultra high endurance and thermally stable selector based on TeAsGeSiSe chalcogenides compatible with BEOL IC Integration for cross-point PCM. IEEE International Electron Devices Meeting, 2.2.1(2017).
[101] W C Chien, C W Yeh, R L Bruce et al. A study on OTS-PCM pillar cell for 3-D stackable memory. IEEE Trans Electron Dev, 65, 5172(2018).
[102] Y Koo, S Lee, S Park et al. Simple binary ovonic threshold switching material SiTe and its excellent selector performance for high-density memory array application. IEEE Electron Dev Lett, 38, 568(2017).
[103] J Ho Lee, G Hwan Kim, Y Bae Ahn et al. Threshold switching in Si-As-Te thin film for the selector device of crossbar resistive memory. Appl Phy Lett, 100, 123505(2012).
[104] S A Chekol, J Yoo, H Hwang. Thermally stable Te-based binary OTS device for selector application. Non-Volatile Memory Technology Symposium, 1(2018).
[105] J Yoo, D Lee, J Park et al. Steep slope field-effect transistors with B–Te-based ovonic threshold switch device. IEEE J Electron Dev Soc, 6, 821(2018).
[106] Y Koo, H Hwang. Zn1−xTex Ovonic threshold switching device performance and its correlation to material parameters. Sci Rep, 8, 1(2018).
[107] J Yoo, Y Koo, S A Chekol et al. Te-based binary OTS selectors with excellent selectivity (>105), endurance (>108) and thermal stability (>450°C). IEEE Symposium on VLSI Technology, 207(2018).
[108] V Adinolfi, L Cheng, M Laudato et al. Composition-controlled atomic layer deposition of phase-change memories and ovonic threshold switches with high performance. ACS Nano, 13, 10440(2019).
[109] S A Chekol, J Yoo, J Park et al. AC–Te-based binary OTS device exhibiting excellent performance and high thermal stability for selector application. Nanotechnology, 29, 345202(2018).
[110] J Shen, S Jia, N Shi et al. Elemental electrical switch enabling phase segregation-free operation. Science, 374, 1390(2021).
[111] S Vaziri, I M Datye, E Ambrosi et al. First Fire-free, Low-voltage (~1.2 V), and Low Off-current (~3 nA) SiOxTey Selectors. IEEE Symposium on VLSI Technology and Circuits, 324-325(2022).
[112] K T Jacob, C Shekhar, M Vinay et al. Thermodynamic properties of niobium oxides. J Chem Eng Data, 55, 4854(2010).
[113] A Chen, Y Fu, G Ma et al. The co-improvement of selectivity and uniformity on NbOx-based selector by Al-doping. IEEE Electron Dev Lett, 43, 870(2022).
[114] D S Jeon, T D Dongale, T G Kim. Low power Ti-doped NbO2-based selector device with high selectivity and low OFF current. J Alloy Compd, 884, 161041(2021).
[115] D Chen, A Chen, Z Yu et al. Forming-free, ultra-high on-state current, and self-compliance selector based on titanium-doped NbOx thin films. Ceram Int, 47, 22677(2021).
[116] Q Luo, J Yu, X Zhang et al. Nb1–xO2 based universal selector with ultra-high endurance (>1012), high speed (10 ns) and excellentVth Stability. Symposium on VLSI Technology, T236(2019).
[117] P Chen, X Zhang, Z Wu et al. High-yield and uniform NbOx-based threshold switching devices for neuron applications. IEEE Trans Electron Dev, 69, 2391(2022).
[119] L F Abbott. Lapicque’s introduction of the integrate-and-fire model neuron. Brain Res Bull, 50, 303(1999).
[120] A L Hodgkin, A F Huxley. A quantitative description of membrane current and its application to conduction and excitation in nerve. J Physiology, 117, 500(1952).
[121] D Kadetotad, Z Xu, A Mohanty et al. Parallel architecture with resistive crosspoint array for dictionary learning acceleration. IEEE J Em Sel Top C, 5, 194(2015).
[122] J Lin, S Sonde, C Chen et al. Low-voltage artificial neuron using feedback engineered insulator-to-metal-transition devices. IEEE International Electron Devices Meeting, 34.5.1(2016).
[123] X Zhang, W Wang, Q Liu et al. An artificial neuron based on a threshold switching memristor. IEEE Electron Dev Lett, 39, 308(2017).
[124] Y Zhang, W He, Y Wu et al. Highly compact artificial memristive neuron with low energy consumption. Small, 14, 1802188(2018).
[125] R Cao, X Zhang, S Liu et al. Compact artificial neuron based on anti-ferroelectric transistor. Nat Commun, 13, 7018(2022).
[126] M D Pickett, G Medeiros-Ribeiro, R S Williams. A scalable neuristor built with Mott memristors. Nat Mater, 12, 114(2013).
[127] W Yi, K K Tsang, S K Lam et al. Biological plausibility and stochasticity in scalable VO2 active memristor neurons. Nat Commun, 9, 1(2018).
[128] H Liu, T Wu, X Yan et al. A tantalum disulfide charge-density-wave stochastic artificial neuron for emulating neural statistical properties. Nano Lett, 21, 3465(2021).
[129] Q Wei, J Tang, X Li et al. Artificial neuron with spike frequency adaptation based on mott memristor. 5th IEEE Electron Devices Technology & Manufacturing Conference, 1(2021).
[130] J Luo, L Yu, T Liu et al. Capacitor-less stochastic leaky-FeFET neuron of both excitatory and inhibitory connections for SNN with reduced hardware cost. IEEE International Electron Devices Meeting, 6.4.1(2019).
[131] L Wu, Z Wang, L Bao et al. Implementation of neuronal intrinsic plasticity by oscillatory device in spiking neural network. IEEE Trans Electron Dev, 69, 1830(2022).
[132] Y Wang, H Xu, W Wang et al. A configurable artificial neuron based on a threshold-tunable TiN/NbOₓ/Pt Memristor. IEEE Electron Dev Lett, 43, 631(2022).
[133] G Indiveri, B Linares-Barranco, R Legenstein et al. Integration of nanoscale memristor synapses in neuromorphic computing architectures. Nanotechnology, 24, 384010(2013).
[134] M Prezioso, F Merrikh-Bayat, B D Hoskins et al. Training and operation of an integrated neuromorphic network based on metal-oxide memristors. Nature, 521, 61(2015).
[135] P M Sheridan, F Cai, C Du et al. Sparse coding with memristor networks. Nat Nanotechnology, 12, 784(2017).
[136] P Yao, H Wu, B Gao et al. Fully hardware-implemented memristor convolutional neural network. Nature, 577, 641(2020).
[137] W Wan, R Kubendran, C Schaefer et al. A compute-in-memory chip based on resistive random-access memory. Nature, 608, 504(2022).
[138] Z Wang, S Joshi, S Savel’ev et al. Fully memristive neural networks for pattern classification with unsupervised learning. Nature Electron, 1, 137(2018).
[139] Q Duan, Z Jing, X Zou et al. Spiking neurons with spatiotemporal dynamics and gain modulation for monolithically integrated memristive neural networks. Nat commu, 11, 1(2020).
[140] X Li, J Tang, Q Zhang et al. Power-efficient neural network with artificial dendrites. Nat Nanotech, 15, 776(2020).
[141] X Zhang, Z Wu, J Lu et al. Fully memristive SNNs with temporal coding for fast and low-power edge computing. IEEE International Electron Devices Meeting, 29.6.1(2020).
[142] Z Wang, Q Zheng, J Kang et al. Self-activation neural network based on self-selective memory device with rectified multilevel states. IEEE Trans on Electron Dev, 67, 4166(2020).
[143] X Li, Y Zhong, H Chen et al. A memristors-based dendritic neuron for high-efficiency spatial-temporal information processing. Adv Mater, 2203684(2023).
[144] Y Fu, Y Zhou, X Huang et al. Reconfigurable synaptic and neuronal functions in a V/VOx/HfWOx/Pt memristor for nonpolar spiking convolutional neural network. Adv Func Mater, 2111996(2022).
[145] J Yu, F Zeng, Q Wan et al. Memristive structure of Nb/HfOx/Pd with controllable switching mechanisms to perform featured actions in neuromorphic networks. Nano Res, 15, 8410(2022).
[146] L Mennel, J Symonowicz, S Wachter et al. Ultrafast machine vision with 2D material neural network image sensors. Nature, 579, 62(2020).
[147] C Y Wang, S J Liang, S Wang et al. Gate-tunable van der Waals heterostructure for reconfigurable neural network vision sensor. Sci Adv, 6, 6173(2020).
[148] F Zhou, Y Chai. Near-sensor and in-sensor computing. Nat Electron, 3, 664(2020).
[149] D Dev, M S Shawkat, A Krishnaprasad et al. Artificial nociceptor using 2D MoS2 threshold switching memristor. IEEE Electron Dev Lett, 41, 1440(2020).
[150] J Zhu, X Zhang, M Wang et al. An artificial spiking nociceptor integrating pressure sensors and memristors. IEEE Electron Dev Lett, 43, 962(2022).
[151] C Y Han, Z R Han, S L Fang et al. Characterization and modelling of flexible VO2 Mott memristor for the artificial spiking warm receptor. Adv Mater Int, 9, 2200394(2022).
[152] C Chen, Y He, H Mao et al. A photoelectric spiking neuron for visual depth perception. Adv Mater, 34, 2201895(2022).
[153] Y Wang, Y Gong, S Huang et al. Memristor-based biomimetic compound eye for real-time collision detection. Nat Commun, 12, 5979(2021).
[154] Q Duan, T Zhang, C Liu et al. Artificial multisensory neurons with fused haptic and temperature perception for multimodal in‐sensor computing. Adv Intell Syst, 4, 2200039(2022).
[155] Y G Song, J M Suh, J Y Park et al. Artificial adaptive and maladaptive sensory receptors based on a surface-dominated diffusive memristor. Adv Sci, 9, 2103484(2022).
[156] X Zhang, Y Zhuo, Q Luo et al. An artificial spiking afferent nerve based on Mott memristors for neurorobotics. Nat Commun, 11, 51(2020).
[157] R Yuan, Q Duan, P J Tiw et al. A calibratable sensory neuron based on epitaxial VO2 for spike-based neuromorphic multisensory system. Nat Commun, 13, 1(2022).
[158] J Zhu, X Zhang, R Wang et al. A heterogeneously integrated spiking neuron array for multimode-fused Perception and object classification. Adv Mater, 34, 2200481(2022).
[159] Z Chai, W Shao, W Zhang et al. GeSe-based ovonic threshold switching volatile true random number generator. IEEE Electron Dev Lett, 41, 228(2019).
[160] H Jiang, D Belkin, S E Savel’ev et al. A novel true random number generator based on a stochastic diffusive memristor. Nat Commu, 8, 1(2017).
[161] K S Woo, Y Wang, J Kim et al. A true random number generator using threshold-switching-based memristors in an efficient circuit design. Adv Electron Mater, 5, 1800543(2019).
[162] K S Woo, Y Wang, Y Kim et al. A combination of a volatile-memristor-based true random number generator and a nonlinear-feedback shift register for high-speed encryption. Adv Electron Mater, 6, 1901117(2020).
[163] G Kim, J H In, Y S Kim et al. Self-clocking fast and variation tolerant true random number generator based on a stochastic Mott memristor. Nat Commun, 12, 2906(2021).
[164] Q Ding, H Jiang, J Li et al. Unified 0.75 pJ/Bit TRNG and attack resilient 2F 2/Bit PUF for robust hardware security solutions with 4-layer stacking 3D NbOx threshold switching array. IEEE International Electron Devices Meeting, 39.2.1(2021).
[165] J Shin, E Ko, J Park et al. Super steep-switching (SS~2 mV/decade) phase-FinFET with Pb(Zr0.52Ti048)O3 threshold switching device. Appl Phys Lett, 113, 102104(2018).
[166] C Lee, E Ko, C Shin et al. Steep slope silicon-on-insulator feedback field-effect transistor: design and performance analysis. IEEE Trans Electron Dev, 66, 286(2019).
[167] E Ko, J W Lee, C Shin et al. Negative capacitance FinFET with sub-20-mV/decade subthreshold slope and minimal hysteresis of 0.48 V. IEEE Electron Dev Lett, 38, 418(2017).
[168] N hukla, A V Thathachary, A Agrawal et al. A steep-slope transistor based on abrupt electronic phase transition. Nat Commun, 7812(2015).
[169] J Song, J Park, K Moon et al. Monolithic integration of AgTe/TiO2 based threshold switching device with TiN liner for steep slope field-effect transistors. IEEE International Electron Devices Meeting, 25.3.1(2016).
[170] S Lim, J Yoo, J Song et al. CMOS compatible low-power volatile atomic switch for steep-slope FET devices. Appl Phys Lett, 113, 033501(2018).
[171] N Shukla, B Grisafe, R K Ghosh et al. Ag/HfO2 based threshold switch with extreme non-linearity for unipolar cross-point memory and steep-slope phase-FETs. IEEE International Electron Devices Meeting, 34.6.1(2016).
[172] S Jeong, S Han, H J Lee et al. Abruptly-switching MoS2-channel atomic-threshold-switching field-effect transistor with AgTi/HfO2-based threshold switching device. IEEE Access, 9, 116953(2021).
[173] J H Park, S H Kim, S G Kim et al. Nitrogen-induced filament confinement technique for a highly reliable hafnium-based electrochemical metallization threshold switch and its application to flexible logic circuits. ACS Appl Mater Interfaces, 11, 9182(2019).
[174] M Lanza, R Waser, D Ielmini et al. Standards for the characterization of endurance in resistive switching devices. ACS Nano, 15, 17214(2021).
[175] G Du, C Wang, H Li et al. Bidirectional threshold switching characteristics in Ag/ZrO2/Pt electrochemical metallization cells. AIP Adv, 6, 085316(2016).
[176] B Song, H Xu, S Liu et al. Threshold switching behavior of Ag-SiTe-based selector device and annealing effect on its characteristics. IEEE J Electron Dev Soc, 6, 674(2018).
[177] J Song, A Prakash, D Lee et al. Bidirectional threshold switching in engineered multilayer (Cu2O/Ag: Cu2O/Cu2O) stack for cross-point selector application. Appl Phys Lett, 107, 113504(2015).
[178] Y Sun, X Zhao, C Song et al. Performance-enhancing selector via symmetrical multilayer design. Adv Funct Mater, 29, 1808376(2019).
[179] E Ambrosi, C H Wu, H Y Lee et al. Low variability high endurance and low voltage arsenic-free selectors based on GeCTe. IEEE International Electron Devices Meeting, 28.5.1(2021).
[180] S Jia, H Li, T Gotoh et al. Ultrahigh drive current and large selectivity in GeS selector. Nat Commun, 11, 1(2020).
[181] J Lee, S Kim, S Lee et al. Improving the SiGeAsTe Ovonic Threshold Switching (OTS) Characteristics by Microwave Annealing for Excellent Endurance (> 1011) and Low Drift Characteristics. IEEE Symposium on VLSI Technology and Circuits, 320-321(2022).
[182] Z Wang, J Kang, G Bai et al. Self-selective resistive device with hybrid switching mode for passive crossbar memory application. IEEE Electron Dev Lett, 41, 1009(2020).
[183] T H Yeh, P H Chen, C Y Lin et al. Enhancing threshold switching characteristics and stability of vanadium oxide-based selector with vanadium electrode. IEEE Trans on Electron Dev, 67, 5059(2020).
[184] D Y Kang, A Rani, K J Yoo et al. Improved threshold switching characteristics of vanadium oxide/oxynitride-based multilayer selector in a cross-point array. J Alloy Compd, 922, 166192(2022).
[185] X Zhao, A Chen, J Ji et al. Ultrahigh Uniformity and Stability in NbOx-Based Selector for 3-D Memory by Using Ru Electrode. IEEE Trans Electron Dev, 68, 2255(2021).
[186] M D Pickett, R S Williams. Sub-100 fJ and sub-nanosecond thermally driven threshold switching in niobium oxide crosspoint nanodevices. Nanotech, 23, 215202(2012).
[187] T Hennen, D Bedau, J A J Rupp et al. Forming-free Mott-oxide threshold selector nanodevice showing s-type NDR with high endurance (> 1012 cycles), excellent Vth stability (5%), fast (< 10 ns) switching, and promising scaling properties. IEEE International Electron Devices Meeting, 37.5.1(2018).
[188] Y Fu, Y Zhou, X Huang et al. Forming-free and Annealing-free V/VOx/HfWOx/Pt Device Exhibiting Reconfigurable Threshold and Resistive switching with high speed (< 30ns) and high endurance (> 1012/> 1010). IEEE International Electron Devices Meeting, 12.6.1(2021).
[189] A Chen, Y He, G Ma et al. Improved uniformity and threshold voltage in NbOx-ZrO2 selectors. Appl Phys Lett, 119, 073503(2021).