CHEN Shu-yi, ZHAO Quan-ming, DONG Da-ming. Application of Near Infrared Spectroscopy Combined with Comparative Principal Component Analysis for Pesticide Residue Detection in Fruit[J]. Spectroscopy and Spectral Analysis, 2020, 40(3): 917

Search by keywords or author
- Spectroscopy and Spectral Analysis
- Vol. 40, Issue 3, 917 (2020)
Abstract
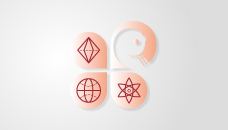
Set citation alerts for the article
Please enter your email address