Jingyu Liu, Huaiyu Cai, Wenyue Hao, Tingtao Zuo, Zhongwei Jia, Yi Wang, Xiaodong Chen. Intravascular ultrasound image segmentation combining polar coordinate modeling and a neural network[J]. Opto-Electronic Engineering, 2023, 50(1): 220118

Search by keywords or author
- Opto-Electronic Engineering
- Vol. 50, Issue 1, 220118 (2023)
Abstract
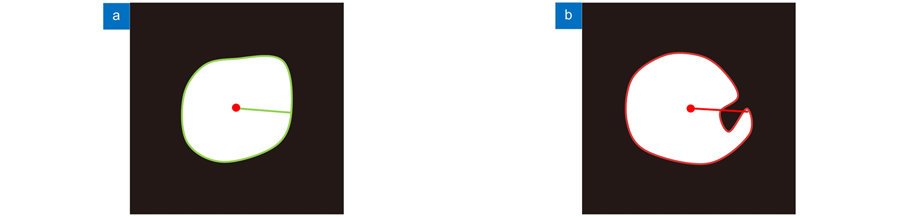
Set citation alerts for the article
Please enter your email address