Abstract
Epilepsy is a common neurological disorder that occurs at all ages. Epilepsy not only brings physical pain to patients, but also brings a huge burden to the lives of patients and their families. At present, epilepsy detection is still achieved through the observation of electroencephalography (EEG) by medical staff. However, this process takes a long time and consumes energy, which will create a huge workload to medical staff. Therefore, it is particularly important to realize the automatic detection of epilepsy. This paper introduces, in detail, the overall framework of EEG-based automatic epilepsy identification and the typical methods involved in each step. Aiming at the core modules, that is, signal acquisition analog front end (AFE), feature extraction and classifier selection, method summary and theoretical explanation are carried out. Finally, the future research directions in the field of automatic detection of epilepsy are prospected.Introduction
Epilepsy is a brain disorder that occurs due to hypersynchronous firing of neurons in the brain, an abnormal electrical activity of the brain. Epilepsy, characterized by involuntary body movements, loss of consciousness, and loss of bowel or bladder control, is the most common chronic neurological disorder[1]. According to written records, epilepsy has a long history. The word "epilepsy" appeared in 4000 BC. Up to now, about 50 million people suffer from epilepsy around the world. Sudden seizures of epilepsy may occur in various unexpected situations. For example, when the seizure occurs, if the patient is in an area near water, and the patient accidentally falls into the water due to uncontrollable body control, this could result in the serious consequence of drowning. In addition, epilepsy patients may also have speech disorders, memory loss, depression and other psychological disorders, which may even threaten the patient's health in severe cases. Due to the severity of epilepsy, it is of great clinical significance to diagnose and detect epilepsy in time to minimize its threat to human life[2].
Detecting epileptic seizures as early as possible, giving appropriate and reasonable treatment, and timely manual intervention before the seizure or recurrence, can bring more treatment possibilities to epilepsy patients and alleviate their symptoms. According to the abnormal repetitive neural discharge characteristics in the epileptic focus during the onset of epilepsy, doctors can detect the onset of epilepsy by observing the discharge phenomenon of the patient's brain. Electroencephalography (EEG) monitors the electrical function of the brain. Epilepsy can be effectively studied by generating multidimensional time series from EEG recordings that are complex, non-stationary, nonlinear, and random. EEG can be divided into diagnostic, localization, and monitoring applications. As an effective means of monitoring epileptic seizures, EEG is widely used clinically.
At present, such EEG analysis work is mainly through the doctor's visual inspection and manual labeling[3], but this kind of inspection has limitations, mainly reflected in: (1) The onset time and duration of epileptic seizures are still uncertain; it is very difficult to read and analyze the data of epileptic seizures from a large amount of EEG data, and the analysis process relies heavily on the subjective judgment of the examiner. (2) There is a large demand for professionals; there are many patients who need to be diagnosed with EEG. To confirm epilepsy patients among many patients, more diagnosticians are needed. Under the pressure of heavy workload, doctors may cause false detection and missed detection for the diagnosis of epileptic seizures. (3) It requires clinical professional diagnostic personnel to have extensive knowledge and experience in clinical diagnosis. Since the EEG will collect background noise at the same time during the acquisition process, it will increase the difficulty of manual inspection.
Generally speaking, patients with different types of illnesses have different symptoms during seizures. Therefore, it is extremely important to fully understand the onset patterns of epilepsy patients. However, the classification of epilepsy is a time-consuming and tedious task, which places a heavy burden on clinicians. In view of this, people are working hard to develop an automatic detection system to reduce the workload to assist neurologists in detecting EEG signals during epileptic seizures and relieve the burden on physicians.
A flowchart of EEG-based automatic epilepsy detection is shown in Fig. 1. The core problem of epilepsy automatic detection can be summarized into three sub-problems: signal acquisition, feature extraction and classifier design. This article will summarize the current research progress at home and abroad from these three aspects, and combine the current challenges in this field, and look forward to the future research direction, in order to provide a useful reference for research in this field. The main contributions of this paper are as follows: (1) A summary of the analog front-end (AFE) equipment based on EEG signal acquisition. (2) A comprehensive introduction to four common features of time domain, frequency domain, time-frequency domain and nonlinear analysis. (3) The classification methods in epilepsy detection are summarized in detail. (4) Suggestions and prospects are given for future research directions in the field of epilepsy automatic detection.
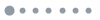
Figure 1.(Color online) A flowchart of EEG-based automatic epilepsy detection.
EEG basics
EEG is a map of electrical potential activity that demonstrates spontaneous, continuous, and rhythmic electrical potential changes in neurons in the brain. It can display the physiological, psychological and disease information of the human body. Due to its low cost and real-time characteristics, EEG has been widely used in the medical field. So far, EEG technology has already become one of the important means of clinical detection.
The EEG signal is a non-stationary signal with the characteristics of suddenness and randomness. According to research, the analysis frequency range of EEG signals is between 0.5 and 100 Hz, and most of them are concentrated in 0.5−70 Hz. According to frequency, normal EEG signals can be divided into four bands: δ, θ, α, β and γ waves, where different frequency bands of EEG reflect different states of the brain.
(1) δ wave: It is mainly distributed in the frequency range between 0.5 and 3 Hz, and the amplitude of the signal is between 20 and 200 μV. Its amplitude is often the highest, while the waveform is the slowest. This waveform is mainly concentrated in the areas of the frontal and occipital lobes and occurs mainly during deep sleep or deep anesthesia.
(2) θ wave: It is mainly distributed in the frequency range between 4 and 7 Hz, and the amplitude of the signal is between 20 and 150 μV. According to research, this band mainly appears in the frontal and parietal regions and usually appears in early childhood. This waveform may occur during sleepiness and arousal phases in older children and adults. In addition, this wave can also appear when encountering difficulties or feeling depressed, which is primarily associated with relaxation, meditation and creativity.
(3) α wave: It is mainly distributed in the frequency range between 8 and 13 Hz, and the amplitude of the signal is between 20 and 100 μV. This waveform is different from the previous waveforms in the distribution range, and exists in multiple regions of the brain, but it is mainly concentrated in the top and occipital regions of the brain. This waveform appears when the eyes are closed or relaxed, and fades away when the eyes are open or mentally drained. When the state of thinking is suddenly interrupted by external stimuli, the wave will disappear quickly. This phenomenon is usually called the wave-blocking phenomenon.
(4) β wave: It is mainly distributed in the frequency range between 14 and 30 Hz, and the amplitude of the signal is between 5 and 20 μV. This wave mainly occurs in the frontal, parietal and central regions of the brain. This wave is often associated with active, busy or anxious thinking and active attention, and is strongly associated with motor behavior, attenuating during active movement. Studies have found that this wave often occurs during the day in a state of waking consciousness. At the same time, because it is related to imaginary EEG signals, it is of great significance in the field of EEG.
(5) γ wave: It is mainly distributed in the frequency band greater than 30 Hz, and the amplitude of the signal is less than 20 μV. This wave mainly occurs in the frontal and parietal regions of the brain, during extreme hyperactivity, or when neuronal excitation is greatly increased. However, it is generally considered that this wave has little to do with the characteristics of the EEG signal, so in the research of processing EEG signals, the high frequency is usually filtered.
Epilepsy is a chronic neurological disease caused by excessive discharge of central neurons. EEG signals during epileptic seizures are quite different from normal conditions. The waveform, amplitude, frequency and other characteristics of EEG signals are often used to distinguish epileptic signals from non-epileptic signals. At present, the most important way is to use the waveform changes in the EEG to detect epilepsy signals, so as to carry out further treatment. During the course of the research, it was found that sharp waves, spike waves, and multi-spike slow waves usually appear during epileptic seizures.
EEG acquisition equipment
As early as the late 18th century, scientists demonstrated that certain animals were capable of generating bioelectric waveforms[4], and by the end of the 19th century, it was possible to measure human potentials such as electrocardiography (ECG), EEG, and electromyography (EMG), yet these techniques were not commonly used in clinical practice until the 1940s and 1950s. As the most complex organ in the human body, the brain has been a field of relentless exploration for scientists, and EEG signals are one of the critical keys to this amazing field.
The first human detection of EEG signals on the surface of the scalp was in 1924, when Hans Berger, Professor of Neurology at the University of Jena, Germany, discovered alpha brain waves from the scalp of young children. Initially, Berger used two platinum needle-like electrodes inserted into the cerebral cortex at the site of the subject's skull injury to acquire signals, but later, after repeated trials, it was demonstrated that EEG signals could be acquired through electrodes from the superficial skin of the scalp without the insertion of invasive electrodes, making this non-invasive EEG acquisition technique more suitable for wearable device applications.
In order to obtain the EEG signal, the sensor-electrode can be used to collect the signal and transmit the data to the back-end system for further processing[5]. However, since the EEG signal is an extremely weak neurophysiological signal, there are certain requirements for the amplification and anti-interference ability of the acquisition system. It is necessary to ensure that the signal-to-noise ratio (SNR) does not affect the subsequent analysis, and it must have a high enough resolution. At the same time, the EEG signal reflects the change of the instantaneous local field potential of the neuron neural activity, so the requirement for time resolution is also very high. Therefore, the hardware acquisition system must meet the requirements of high resolution and high sampling rate[6]. In high-density EEG signal acquisition scenarios, high resolution and high sampling rate acquisition will generate a large amount of data. Therefore, traditional EEG acquisition equipment choosing a wired transmission method (usually optical fiber transmission) enhances the reliability, stability, and transmission rate of data transmission, and also indirectly loses portability[7]. Nowadays, the application research on EEG has become more diverse. EEG acquisition not only needs to meet the performance requirements of big data, high reliability, and high speed, but also needs to meet the acquisition requirements of mobility, continuous monitoring, and comfortable wearing in daily life. Therefore, research on high-performance portable wireless EEG acquisition technology has great application value and practical significance[8].
The following summarizes the research status and development trend of the hardware system of EEG acquisition equipment, summarizes the basic structure of EEG acquisition equipment, and focuses on sorting out and discussing different methods for optimizing EEG acquisition equipment.
EEG acquisition equipment is a data source for various EEG applications and research. As a precision test instrument, it is necessary to ensure the safety of the user, as well as the high precision and high common mode rejection ratio (CMRR) of the system, and at the same time reduce the power consumption, volume and cost of the system as much as possible. EEG signals have low frequency and small amplitude, and are easily affected by various noises and interferences. Therefore, when designing the analog front-end circuit, the following conditions need to be met in order to accurately extract the signal:
(1) Variable gain design. A first-stage variable gain amplifier circuit is designed to avoid saturation of the output signal due to changes in EEG signals.
(2) High CMRR and input impedance. The 50 Hz power frequency interference in AC will be introduced, which is a common mode interference for the analog front-end system. Therefore, the circuit should improve the common-mode rejection ratio to suppress common-mode interference. In the skin-electrode interface, there is an interface impedance, which usually reaches the megohm level. Therefore, the input impedance of the analog front-end system must be high enough, otherwise the signal reaching the analog front-end will be seriously attenuated.
(3) Low-input referred noise. Noise performance is one of the most important indicators to be considered in the design of an EEG signal recording system. The input referred noise determines the minimum amplitude of the EEG signal that the system can record. In the design of amplifier circuits, the signal-to-noise ratio is usually used to determine the noise performance that the circuit needs to achieve. The SNR of the front-end circuit of the EEG signal recording system is required to be greater than 40 dB. For the signal recording system, the typical value of the intracranial EEG signal is 500 µVrms, so the equivalent input noise of the analog front-end circuit must be less than 5 µVrms.
(4) Low power consumption. In recent years, wearable medical devices have gradually become a development trend. Such devices require long-term, real-time monitoring and the detection of patients. Therefore, low power consumption design is required to prolong battery life. Excessive power consumption of the EEG recording circuit system will seriously heat up the implanted device, thereby increasing the temperature of the contacted brain tissue, thereby affecting normal data recording, and even causing damage to the brain tissue, which is not allowed. Low power consumption design is one of the technical bottlenecks restricting the implementation of implantable systems. Especially in the case of a large number of recording channels, the power consumption requirements for the single-channel front-end amplifier are very high, usually in the order of µW.
Traditional EEG acquisition equipment usually includes the following main components: an electrode lead interface, preprocessing circuit, analog-to-digital conversion circuit (ADC), processor circuit, storage module, communication circuit and power supply module. Some equipment will add some expansion modules for specific research, such as sensors for collecting other information[9−18]. The block diagram of a typical EEG acquisition system is shown in Fig. 2.
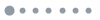
Figure 2.(Color online) Block diagram of a typical EEG acquisition system.
Electrode
The recording electrode is a sensor that converts the brain electrical activity signal of the human body into a voltage (current) signal that can be processed by the circuit. It is a bridge connecting the electrical activity of brain neurons and the circuit system. Electrodes are mainly divided into non-invasive electrodes and invasive electrodes. Non-invasive electrodes are generally used to obtain EEG signals on the surface of the scalp, mainly including wet electrodes, dry electrodes and non-contact electrodes: Wet electrodes are generally composed of conductive gel, Ag/AgCl[19]. Wet electrodes have the following advantages: allowing high-density EEG recordings, higher signal quality and less susceptible to power interference and motion artifacts than dry electrodes. However, the wet electrode itself also has some disadvantages: (1) when collecting physiological electrical signals, in order to establish a good path between the electrode and the subcutaneous tissue, the skin must be pre-treated to remove the cuticle; (2) since the conductive gel on the electrode will slowly volatilize and dry over time, the conductivity of the dried gel will be greatly reduced, so the wet electrode is not suitable for long-term signal acquisition. Dry electrodes generally have a comb-like structure and consist of micro-pillars that directly contact the scalp or slightly penetrate the epidermis of the skin[20−22]. Dry electrodes are difficult to fix on the scalp, and have stronger signal instability and higher impedance. The dry electrode is different from the wet electrode. It does not contain the electrolyte itself, so it is much more convenient and comfortable than the wet electrode. Therefore, dry electrodes are widely used in wearable medical devices. Non-contact electrodes are capacitive electrodes that measure EEG signals spaced from the skin. The use of such electrodes greatly reduces the preparation process for measuring EEG signals, but provides signals with too small amplitudes and is susceptible to motion artifacts[23, 24]. The conductivity and contact impedance values of electrodes vary depending on the electrode material, scalp condition, electrode position and other factors. Generally speaking, the contact impedance of the wet electrode is between 5–50 kΩ, the dry electrode is 1 kΩ–1 MΩ and the conductivity is both between 0.5–5 mS. It is important to note that the contact resistance of electrodes will increase over time and therefore require periodic inspection and replacement.
Invasive electrodes, also known as implantable electrodes, generally refer to that which is surgically implanted on the surface of the dura mater or cortex, or which penetrates the brain to measure and record EEG signals from individual cells or cell groups. Invasive electrodes fall into two broad categories: penetrating and non-penetrating cortical electrodes. Needle-penetrating cortical electrodes are defined as individual microfilaments, microfilament bundles, or arrays that are used for precise positioning by the recording site in the brain, which is used to measure action potentials or LFP[25−27], and non-penetrating electrodes that are generally used, for example extracranial EEG, epidural electrocorticography (ECoG) or cortical ECoG recordings[28]. Invasive electrodes capture more accurate signals but are prone to bodily reactions that cause signal degradation or loss. Generally speaking, the contact impedance of intrusive electrodes is between several hundred Ω and several MΩ, and the conductivity is between 0.1−10 mS.
Preprocessing circuit
After the signal is fed through the electrode interface, it is transmitted to the analog channel. The amplitude of EEG is only at the μV level. During the signal acquisition process, it is extremely susceptible to various interferences from the human body itself and the external environment. The analog front-end circuit needs to remove these interferences as much as possible while amplifying the signal, otherwise the quality of the collected signal will be affected. Common interference factors include electrode direct current (DC) imbalance[29], 50/60 Hz power line interference[30−37], motion artifacts[38] and circuit noise[39], etc. In order to remove the influence of interference and facilitate collection, high gain, high common-mode rejection ratio, high-impedance AFE and a filter with good performance are required.
The main function of the preprocessing circuit is to amplify and filter the original EEG signal. It is the key factor to determine the final signal quality of the EEG acquisition equipment, so it is the crucial research field of the EEG acquisition circuit part. The preprocessing circuit is mainly composed of a low-noise instrumentation amplifier (IA), a programmable gain amplifier (PGA) and a filter. The main function is to provide appropriate gain to the EEG signal while filtering out interference and noise to ensure the quality of the signal input to the ADC. Among them, the IA amplifies the EEG signals collected by the electrodes, and suppresses interference such as electrode offset voltage and low-frequency noise; the PGA selects the corresponding amplification factor according to the amplitude of the EEG signals, and adjusts the swing of the output signal. The signal-to-noise ratio is optimized; the filter filters out the frequency components outside the signal band to avoid noise aliasing in the ADC sampling process.
Instrumentation amplifier (IA)
The IA is the first-stage amplification circuit of the AFE, which provides high gain for the input signal and can attenuate the noise equivalent to the input terminal of the subsequent circuit. Therefore, in the overall architecture of the front-end circuit, usually only the low-noise design of the IA needs to be considered. However, considering the existence of offset and common-mode interference, too high gain will easily cause the output of the amplifier to be saturated. The gain of the IA is generally set at 40 dB. At the same time, the IA must have a large CMRR to suppress 50/60 Hz power line interference. Also, the IA is directly connected to the electrode, so the input impedance needs to be large enough[40−45]. Common IA includes three-op-amp structures, AC capacitive coupling structures, and current feedback amplifier structures. This section will analyze the common structures of low-noise IA and compare their advantages and disadvantages.
(1) Three-op-amp IA: The three-op-amp structure is the most common structure, usually composed of three op-amps, as shown in Fig. 3(a). The front-end gain of the IA is determined by RG, and for the convenience of user configuration, RG is generally realized outside the chip. The first-stage buffer is fully differential. If A1 and A2 are perfectly matched, the input buffer circuit will not amplify the common-mode signal. The CMRR of the circuit is mainly determined by the second-stage operational amplifier and resistor matching, so amplifier gain can be adjusted without affecting CMRR. The input impedance of the three-op-amp structure is high, the distortion is low, and the gain is configurable. However, affected by the resistance matching accuracy, the circuit CMRR generally does not exceed 80 dB. In order to improve the CMRR, laser-cutting resistance matching technology is required, which increases the cost. In addition, the circuit is driven by three op amps, and the feedback network requires the amplifier output impedance to be low enough, so the power consumption is high, and all three op amps contribute noise.
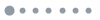
Figure 3.(Color online) Three common IA structures.
(2) AC capacitive coupling IA: As shown in Fig. 3(b) is a typical IA with capacitive coupling and capacitive feedback. The input capacitor Cin is directly connected to the electrode, which isolates the DC offset voltage of the electrode. In order to ensure the circuit has an extremely low high-pass cut-off frequency and provide a common-mode bias for the circuit, a diode-connected MOS pseudo-resistor Rp is generally added, and its parasitic transistor is used to obtain a large resistance of TΩ level. However, pseudo-resistors are easily affected by factors such as process and temperature to produce large resistance fluctuations. The noise of this structure is low and the matching accuracy of the capacitor is high. Meanwhile, the capacitive feedback network does not require additional current. However, this structure also has disadvantages; in order to achieve high gain, the input capacitance is usually large, which increases the chip area, and an additional circuit structure needs to be added to suppress noise, sacrificing power consumption.
(3) Current feedback IA: The structure of the current feedback IA is shown in Fig. 3(c), and the error signal used as feedback is in the form of the current. The resistor network and the transconductance amplifiers Gm1, Gm3 together determine the gain of the current feedback IA. Since the transconductance amplifier under the standard CMOS process is easily affected by temperature and process angle, generally the transconductance of Gm1 and Gm3 are equal, and the gain accuracy is improved through layout-matching technology. The power consumption and noise of the current feedback IA are relatively low, and it is very suitable for offset compensation design, which can effectively improve the CMRR of the circuit. However, since the output voltage is directly fed back to the gate of the Gm3 input transistor, it is difficult to achieve rail-to-rail output, which cannot meet the accuracy requirements of subsequent ADCs.
Programmable gain amplifier (PGA)
Changes in the recording site, environment, and sampling bioelectrodes will cause a wide range of changes in the amplitude of the scalp EEG signal. Therefore, a PGA is required to adjust the overall gain of the pre-circuit to avoid saturation of the output signal. Considering factors such as chip area, noise performance, and circuit power consumption for the first-stage amplifier, the gain of the preamplifier may not meet the amplification requirements of the EEG signal, so a second-stage amplifier is needed to re-amplify the pre-stage signal. The second stage amplifier needs to be designed with adjustable gain. A PGA is usually designed as a closed-loop circuit structure, and the gain of the amplifier is adjusted through a logic control circuit. Adopting a closed-loop structure, the gain accuracy of the amplifier is high, the linearity is relatively good, and the output range is also large. Therefore, it is very suitable for the front-end circuit for extracting biomedical signals such as EEG. There are currently two mainstream PGAs: variable resistor feedback PGA and variable capacitor feedback PGA. The structure of the resistive feedback PGA is shown in Fig. 4(a). Its main components are a high-gain open-loop operational amplifier, input resistor and feedback resistor. When transmitting signals of different amplitudes, the voltage at the source terminal of the MOS transistor will change, thereby affecting the on-resistance of the transistor. This will result in different gains for transmitting signals with different amplitudes, thereby affecting the linearity of the system, and in severe cases, causing signal distortion. In addition, PGAs with resistive feedback have static power consumption and are not suitable for low-power applications. A large resistor value can reduce the impact of static power consumption and MOS on-resistance on system linearity, but it will introduce thermal noise, which is not conducive to low-noise design. The structure of the capacitor feedback PGA is shown in Fig. 4(b). It is mainly composed of an open-loop amplifier, input and feedback capacitors, gain control switches and MOS pseudo-resistors. Its input capacitance and feedback capacitance will not introduce additional noise, so the noise performance of the circuit is better than that of resistance feedback PGA. In addition, since the resistance value of the MOS-Bipolar pseudo-resistor is as high as 1012 Ω, the static power consumption is negligible compared with the resistor feedback type[46−48].
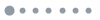
Figure 4.(Color online) Two common PGA structures.
Filter
Three types of filters are commonly used in EEG acquisition equipment: a high-pass filter, power frequency notch filter and low-pass filter.
At the forefront is the high-pass filter, whose main function is to isolate the DC component of the brain potential. It is generally believed that the frequency components of scalp EEG signals related to cognitive tasks are located at 0.5−100 Hz. The starting frequency of the high-pass filter is generally set at about 0.5 Hz, and is usually realized by an active filter. The band-pass amplitude and cut-off frequency of the active filter will not change due to different loads, and it can also dynamically compensate reactive power[49, 50].
The main function of the power frequency notch filter is to filter out the 50 Hz power frequency noise mixed in the EEG signal. In the traditional notch design, the notch with a double-T structure is the most commonly used, but this kind of notch has a practical defect, that is, it requires high precision of components. In order to achieve the ideal effect, the accuracy of resistors and capacitors is required to reach 0.1%, which is very difficult for the current capacitor-processing technology[51, 52].
The role of the low-pass filter is to filter out environmental high-frequency noise interference, mainly environmental radio waves, power supply ripple, and noise caused by active devices themselves[53, 54]. Common low-pass filters used for the analog front-end of EEG acquisition include the RC filter, switched capacitor filter and Gm-C filter.
(1) RC filter: Passive RC filters are generally implemented off-chip, which is not considered in this article. The active RC filter adds a high-gain open-loop operational amplifier. Fig. 5(a) shows a simple first-order active RC filter structure. The active RC filter has a simple structure and high linearity, but it needs to introduce large resistors and capacitors, which will increase the chip area and lead to the deterioration of circuit noise performance, and at the same time, the precision of integrated resistors and capacitors is also poor. Moreover, the MOS pseudo-resistor is not suitable for realizing a low-pass cut-off frequency of several hundred Hz due to its excessively large resistance value, and the pseudo-resistor depends too much on the process and temperature, which will lead to inaccurate time constants.
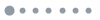
Figure 5.(Color online) Three common filter structures.
(2) Switched capacitor filter: In order to solve the area problem of the RC filter, the switched capacitor filter uses a set of switches and capacitors to be equivalent to a large resistor, and its resistance value is the ratio of the voltage change value on the capacitor to the average current in one clock cycle. Fig. 5(b) is a typical first-order, switched capacitor filter. Switched capacitor filters do not need to use large resistors and capacitors to achieve extremely low cut-off frequency, reduce the layout area, and facilitate on-chip integration. At the same time, the noise of the circuit is reduced. The low-pass cut-off frequency of the structure can be changed by the frequency of the clock, and the adjustment is simple and convenient. However, this structure also has certain disadvantages; in order to avoid aliasing, the clock frequency of the sampling switch needs to satisfy that the sampling frequency is at least twice the signal frequency. This requires the bandwidth of the amplifier to be at least twice the signal bandwidth, so power dissipation increases at high frequencies. In addition, the circuit contains a lot of sampling switches, requiring two-phase non-overlapping clocks, and the design is complicated. At the same time, there are non-ideal factors in the MOS switch, which will affect the accuracy of the sampled signal.
(3) Gm-C filter: Unlike active RC filters, Gm-C filter circuits only contain capacitors and transconductance units and do not require the use of resistors. The function of the transconductance unit is to convert the input voltage into the current. In the Gm-C filter, the basic unit of the integrator is composed of a transconductance unit and a capacitor, which are then cascaded to form a filter. Fig. 5(c) is a basic second-order Gm-C filter structure diagram. It is relatively easy for the Gm-C filter to achieve a higher cut-off frequency. The cut-off frequency can be changed by changing the transconductance of the third and fourth stages and the capacitance of the interstage capacitor, and the tuning is simple and convenient. However, the linearity of the circuit is relatively low, and it is more sensitive to parasitic capacitance. Especially the transconductance unit, its transconductance value is relatively low, and the deviation is very large at the process corner. In addition, if the transconductance of the transconductance unit is at the μS level and the required cut-off rate is to be achieved, the value of the interstage capacitance needs to be as high as the nF level. If no off-chip capacitor is used, the capacitor area will be extremely large.
Analog-to-digital conversion circuit (ADC)
The analog-to-digital conversion circuit in the acquisition module is a key part of EEG signal acquisition. EEG signals are extremely weak and are easily disturbed by noise. Good ADC performance can prevent EEG signals from being distorted due to noise coupling and improve signal-to-noise ratio, ensuring high-fidelity transmission. At the same time, the effective frequency range of the EEG signal on the surface of the scalp is 0.1−100 Hz. According to the Nyquist sampling theorem, the ADC sampling frequency is at least twice the maximum signal frequency to ensure that no aliasing occurs within the signal bandwidth. In addition, since the DC interference is not filtered out in the ADC front-end circuit, the signal for analog-to-digital conversion will be coupled into the DC component, and the minimum amplitude of the EEG signal is greater than 10 μV. In order to prevent the introduction of excessive quantization noise, the minimum resolution of the ADC should be below 1 μV. In order to ensure the quality of the acquired signal, the ADC generally chooses a device with low noise and large input impedance[55−58].
Processor module
According to different functions and applications, some EEG acquisition devices require processors to run signal-processing algorithms. The processor circuits of such devices are usually implemented by DSP, FPGA or microcomputers with high computing power. The processor sends the processed data to the upper computer through the communication interface, or directly outputs to the display screen for display, or directly controls some controlled equipment. However, some EEG devices only need the processor to execute control instructions, and send the collected EEG signal data to the host computer through the communication circuit, and the host computer runs the EEG signal processing algorithm. In order to facilitate the data management in the process, the EEG acquisition equipment usually has a storage module, and the storage space varies according to the application[59].
Integrated chip
The bulky EEG acquisition equipment in the past was mainly used for scientific research and medical treatment, and it was difficult to apply it in daily life. Since the 1990s, foreign countries have begun to study integrated chips for EEG acquisition[60]. In recent years, the rapid development of microelectronics technology has brought opportunities for the application of portable EEG acquisition equipment and even body area networks[9, 13, 15, 16, 61−70].
Qian et al. proposed a micropower low-noise neural front-end circuit capable of recording epileptic fast ripples (FR). The front-end circuit consists of a preamplifier and a 6th-order bandpass filter, which is designed for signal sensing in deep brain stimulators for epilepsy in the future. To improve the noise and power trade-off in the preamplifier, a current-splitting technique combining with an output-branch current scaling technique in a folded-cascode amplifier structure is proposed. Experimental results show that the preamplifier owns 39.4 dB DC gain, 0.36 to 1.3 kHz of −3 dB bandwidth, and 3.07 μVrms total input-referred noise and consume 2.4 μW under a 2.8 V power supply[61].
Robinet et al. proposed a 32-channel recording ASIC that can realize low-noise amplification and analog filtering functions. Meanwhile, it can also own a 12-bit ADC function and offer programmable output rates by using a serial peripheralinterface (SPI). The entire system is designed for use in a remotely powered wireless implantable ECoG recording system. The measured input-referred noise of each recording channel is 0.7 μVrms on a 0.5−300 Hz bandwidth. The ASIC was implemented in a 0.35-μm CMOS process and the total die area is 86 mm2, the analog power consumption is limited to 134 μW for per channel[62].
Zhou et al. proposed a mixed-signal AFE ASIC for an EEG acquisition system. It will be attached to the scalp via electrodes and will be used to detect electrical signals from the brain, amplify the collected signals and convert them into digital data. The EEG signals of each channel are amplified by a pre-amplifier, whose gain is from 10 to 1000. After the pre-amplifier, a two-stage low pass filter is used with an optional bandwidth of 80 or 800 Hz. The input referred noise of the whole system is 2.2 μVrms for a signal bandwidth of 1−1 kHz. The ADC has a resolution of 12 bits based on the successive approximation algorithm. The power consumption of the analog part for the ASIC is 3.5 mW under a 1.8 V supply[63]. Fig. 6 shows the block diagram of the EEG acquisition AFE.
![(Color online) Block diagram of the EEG acquisition AFE[63].](/Images/icon/loading.gif)
Figure 6.(Color online) Block diagram of the EEG acquisition AFE[63].
Yoo et al. proposed a scalable 8-channel EEG acquisition SoC for the continuous detection and recording of patient-specific seizure activity from scalp EEG. The chip integrates an 8-channel AFE, an 8-channel feature extraction processor, a classification processor and 64 kB of memory. The AFE module is powered by 1.8 V and consumes a total of 66 μW in the 8-channel operation mode. The bandwidth of 30/100 Hz is scalable, and the input reference noise is only 0.91 μVrms in the bandwidth of 0.5−100 Hz. The GBW controller is used to provide real-time gain and bandwidth feedback to the AFE to maintain accuracy[64].
Xu et al. proposed an 8-channel gel-free EEG/electrode-tissue impedance (ETI) acquisition system, which consisted of nine active electrodes (AEs) and one back-end (BE) analog signal processor. The AEs are applied to amplify the weak EEG signals, and their low output impedance can suppress artifacts and 50/60 Hz power frequency interference. The common-mode feed-forward (CMFF) scheme improves the CMRR of the AE pairs by 25 dB. The BE is applied to post-processes and digitize the analog outputs of the AEs, which can also configure them by a single-wire pulse width modulation (PWM) protocol. The input impedance of each EEG channel is 1.2 GΩ at 20 Hz, and 1.75 µVrms (0.5−100 Hz) input-referred noise, 84 dB CMRR and ±250 mV electrode offset rejection capability. The EEG acquisition system was fabricated in a standard 0.18 µm CMOS process, and the power is less than 700 µW under a 1.8 V supply[9].
Muller et al. proposed a minimally invasive 64-channel ECoG signal acquisition system. The device consists of a highly flexible, high-density 64-channel electrode array and a flexible antenna with a total chip power consumption of only 225 μW, enabling long-term stable neural recordings. The input reference noise of the AFE module can reach as low as 1.23 μVrms at a chopping frequency of 16 kHz, but the system has not been tested in a hospital environment and is slightly deficient compared to the ECoG signals acquired under conventional conditions[65].
Smith et al. proposed a technique for AFE design specific to EEG. This paper improves noise performance of a similar system and proposes an equalization technique, which can reduce the ADC dynamic range requirements and does not require a variable gain amplifier (VGA). The prototype is fabricated in 1p9m 65 nm CMOS, which can take advantage of the presented findings to realize high-fidelity, full-spectrum ECoG recording. The power consumption of the entire analog front end is 1.08 μW over a 150 Hz bandwidth and only 7 bits of ADC resolution[66].
Tohidi et al. proposed a low-power instrumentational amplifier (IA), which is designed for EEG signal acquisition targeting seizure detection. The power of the proposed structure per channel is 0.92 µW under a 0.8 V supply. Due to the use of buffer structure and impedance-enhanced loop, the input impedance reaches 160 and 16 GΩ at 1 and 10 Hz, respectively. Also, the chopping techniques lead to an input-referred noise of 1.7 µVrms over the bandwidth of 0.5−100 Hz with a noise efficiency factor (NEF) of 3.87 and a CMRR of 137 dB[16]. Fig. 7 shows the proposed instrumentational amplifier structure.
![(Color online) Proposed instrumentational amplifier structure[16].](/Images/icon/loading.gif)
Figure 7.(Color online) Proposed instrumentational amplifier structure[16].
Karimi-Bidhendi et al. proposed two ultra-low-power EEG signal acquisition analog front-end systems working in the weak inversion area. The power supply voltage of the two AFEs is only 0.4/0.6 V, and a capacitively coupled P−N complementary OTA is used to achieve power consumption of 0.216/0.69 μW and equivalent input noise of 2.19/2.3 μVrms respectively. Human tests have shown that the system can reliably record EEG and ECoG neural signals and can be used as the basis for a small implantable ultra-low-power EEG signal acquisition unit, but it still needs to further reduce the sensitivity of the front-end to environmental noise[13].
Wu et al. proposed a 16-channel AFE EEG signal acquisition device for a closed-loop seizure control system. It is consisted of 16 input protection circuits, 16 auto-reset chopper-stabilized capacitive-coupled instrumentation amplifiers (AR-CSCCIA) with band-pass filters, 16 programmable transconductance gain amplifiers, a multiplexer, a transimpedance amplifier, and a SAR ADC. The proposed AFE amplifier has 49.1/59.4/67.9-dB programmable gain and 2.02-µVrms input-referred noise in a bandwidth of 0.59−117 Hz. The power consumption of the proposed AFE amplifier is 3.26 µW of per channel, and the NEF is 3.36. The system has been successfully performed in vivo animal tests and verifies its functions. Experimental results show that the proposed AFE circuit is suitable for closed-loop seizure control devices[15]. Fig. 8 shows the system architecture of the proposed ECoG-signal acquisition system.
![(Color online) System architecture of the proposed ECoG-signal acquisition system[15].](/Images/icon/loading.gif)
Figure 8.(Color online) System architecture of the proposed ECoG-signal acquisition system[15].
Tohidi et al. proposed a low-power-consumption, high-input impedance AFE circuit design scheme for a continuous working EEG acquisition system. In addition to low-voltage low-power techniques, the design uses active electrodes to increase input impedance and a right-leg drive circuit to improve the common-mode rejection ratio (CMRR). After measurement, the input impedance is 102 GΩ@1 Hz and 5.2 GΩ@20 Hz, the equivalent input noise in the 0.5 Hz 1.2 kHz integration band is 1.5 μVrms, and the CMRR is greater than 108 dB[67].
Tang et al. proposed a multi-channel AFE ASIC for wearable EEG recording systems. Chopping stabilization (CS) and time-division-multiplexing (TDM) are combined uniformly to optimize the input-referred noise and the system-level CMRR targeting multi-channel AFE. Using this TDM/CS structure, multiple channels can share the same secondary amplifier, helping to reduce chip size and power consumption. The system includes dual feedback loops for input impedance boosting and electrode offset cancellation. The power consumption of the AFE is 24 µW at a 1 V supply voltage. The input referred noise is 0.63 µVrms in 0.5−100 Hz and the input impedance is boosted to 560 MΩ at 50 Hz. The CMRR of the amplifier and system-level AFE are 89 and 82 dB, respectively[68].
Gao et al. proposed a two-stage time-division multiplexing analog front-end (TDMAFE) for multi-channel EEG signal acquisition. They proposed a four-channel synchronous TDM technology eliminating the mismatch among the four-channel signals. To increase input impedance, an impedance-boosted chopper based on the differential difference amplifier is proposed. And to eliminate DC offset, an improved low-noise digitally controlled DC servo loop (DCDSL) is proposed. The entire AFE system is 1.2 mm2 and the power consumption is 30.6 μW under a 1.2-V supply. Post-simulation results show that the gain in the AFE ranges from 34 to 60 dB. The range of the input offset cancellation is ±300 mV. The equivalent input impedance is boosted to 1.6 GΩ. The equivalent integrated noise is 1.66 μVrms at the range of 0.1−100 Hz[69].
Huang et al. proposed an AFE amplifier (AFEA), which can be applied for EEG recording. A capacitively coupled chopper instrumentation amplifier (CCCIA) with chopper modulation is used in the proposed AFEA to suppress flicker noise within the bandwidth and achieve lower noise. In this paper, the EEG AFEA has the tunable gains of 60.4/69.6/79.2 dB. The input referred noise within the bandwidth is 0.839 μVrms, the area is 2.576 mm2 and power consumption is 83.2 μW[70]. Table 1 shows the EEG signal AFE performance summary.
Table Infomation Is Not Enable
Epilepsy signal classification technology
With the advancement of artificial intelligence technology in recent years, researchers continue to dig in the field of automatic diagnosis of epilepsy based on EEG, and have obtained many detection methods to solve the problem. This algorithm is of great help to the automatic detection of epilepsy, because it can independently learn the classification rules of the data and improve the performance of data expansion. The core issues of epilepsy detection technology are feature selection and classifier design. Feature selection aims to select useful features depicting epileptic signals; the classification stage classifies the signals according to the selected features, showing different classification results of epileptic states. The following is an introduction to the research progress of epilepsy signal classification technology from four aspects: several public datasets currently used in epilepsy detection, feature extraction and the selection of epilepsy EEG signals, an epilepsy detection algorithm based on classical machine learning and an epilepsy detection algorithm based on deep learning.
Publicly available epilepsy detection datasets
It is necessary for a lot of scientists and researchers to use a suitable dataset for assessing the performance of their proposed methods. Therefore, we need to collect a large number of EEG signals for the detection and prediction of epileptic seizures. Among the commonly used methods for detecting brain activity, EEG recording is the most popular. These records play a key role in exploring the development of machine learning classifiers, which can help to find effective methods for epilepsy seizure detection in different aspects. The existence of publicly available datasets is important because they provide a reference base to analyze experimental results and allow researchers to compare experimental results with others. In this section, we introduce several publicly available datasets that are popular in this research field.
Children Hospital Boston, Massachusetts Institute of Technology—EEG dataset
This dataset was collected in Boston Children’s Hospital, which included EEG recordings of pediatric patients with intractable seizures. Physicians monitored the participants for several days after antiepileptic drugs were stopped, to describe their seizures state and evaluate their suitability for surgical intervention.
The entire dataset includes 23 cases from 22 subjects (5 males, 3 to 22 years; 17 females, 1.5 to 19 years) (the chb21 and the chb01 were collected from the same female subject with the interval of 1.5 years)[71]. Every case (chb01, chb02, chb03, chb04, etc.) included about 9−42 continuous files of a subject. Due to the limitations of hardware conditions, there are gaps existing between consecutive files. During this gap, no EEG signals were recorded; usually, the gaps were 10 seconds or less, but sometimes longer gaps existed. All signals of this dataset were sampled at 256 Hz and 16-bit resolution by using 23−26 electrodes. During data collection, there were not any enhancement steps implemented. Most files include 23 EEG recordings, some include 24 or 26. The international 10−20 EEG electrode location and nomenclature system were used during recording. In some files, there were some other signals recorded, for example, ECG signals and vagus nerve stimulation (VNS) signals.
Long-term SWEC-ETHZ—EEG dataset
This dataset includes 18 patients of the Inselspital Bern, P1–P18. They were from the epilepsy surgery program[72]. The number of seizures for each patient ranged from 2 to 23. The total duration recorded between seizures varied from 41 to 293 h. Among the 18 patients, the number of fast and short seizures for P8 and P14 were very high, up to 70 and 60, respectively. Therefore, only 4 and 2 seizures need to be considered as their main seizures. This dataset has a total recording time of 2656 h, of which 116 seizures were labeled by an experienced board-certified epileptologist (K.S.).
Bern-Barcelona—EEG dataset
This dataset contains intracranial EEG recordings of five epileptic patients, divided into two categories. In the first category, EEG signals were collected in the epileptic area, which is called focal (F). In the second category, EEG signals were collected in the non-epileptic area which is called non-focal (N). Each category includes the 3750 pairs of recordings. The two signals of F class were collected from the epileptic signal originating channel and another adjacent channel. The signals of the N class were collected from two adjacent channels located in non-epileptic regions. All EEG recordings were filtered by using a 4th-order Butterworth filter, and the filter frequency band is from 0.5 to 150 Hz. All signals of the original dataset were recorded lasting 20 s, where the sampling frequency is 1024 Hz. Then, all signals were down-sampled to 512 Hz. At last, the median was subtracted across all channels. The original dataset did not include recordings of the epileptic seizures and recordings of 3 h after the last seizure[73].
Bonn University—EEG dataset
This dataset includes 500 recordings of 500 different patients, where each record lasts 23 s[74]. These signals were collected by using surface electrodes, and the sampling frequency is 173.61 Hz. In this dataset, each record lasts 23 s long and is split into 23 non-overlapping segments. Each segment contains 1 second of data, which is 173 samples, and other segmentation strategies cannot be considered. This method of splitting is the limitation of the dataset, and the benefit is that it allows fair comparison with previous research results. The whole dataset contains 11 500 recordings of 1 s. The entire dataset contains five categories of recordings, and each category contains the number of signals in the phase. The first class of recordings were collected from healthy subjects, and they were with their eyes open, called Z. The second class were also collected from healthy subjects, but with their eyes closed, called O. The third class is the ictal group, which were collected from epileptic subjects during a seizure, called S. The fourth class includes the interictal state from the hippocampal location of the brain, called N. And the last class includes the interictal state from the epileptogenic zone of the brain, called F.
The Freiburg—EEG dataset
This dataset includes 21 patients’ invasive EEG recordings, which with medically intractable focal epilepsy. These data were collected in the Epilepsy Center of the University Hospital of Freiburg, Germany[75]. In this dataset, 11 patients had epilepsy foci in the neocortical brain structure, 8 in the hippocampus, and 2 in both. Intracranial grid-, strip-, and depth-electrodes were used to get a high SNR, fewer artifacts, and record directly in focal regions. The EEG signals of this dataset were collected by using a Neurofile NT digital video EEG system, which owned 128 channels, 256 Hz sampling frequency, and a 16-bit ADC. There were not notch or band pass filters being used.
Feature extraction and selection
It is an important step to realize the automatic detection of epilepsy by analyzing the signal and extracting effective features as the classification basis. Reasonable and typical epileptic EEG features can fully characterize the EEG signal pattern, and effectively describe the difference between EEG signals in various states such as seizures and normal states, highlighting the difference between spikes and background signals, thereby helping the classification model to identify epileptic seizures effective screening. The quality of features seriously affects the final classification performance.
In general, the features used for epilepsy detection can be divided into the following four categories: time-domain features, time-frequency domain features, and nonlinear features.
Time-domain features are the most basic features in EEG signal processing. Groups of statistical parameters have been frequently used to discriminate between ictal and normal patterns, because it is assumed that EEG statistical distributions during a seizure and normal periods are different. These parameters are mean, variance, mode, median, skewness, and kurtosis. The minimum and maximum values are also used to quantify the range of data or the magnitude of the signal baseline. Other statistical parameters include coefficient of variation (CV) defined as the ratio of the standard deviation (SD) to the sample mean that explains the dispersion of the data in relation to the population mean. Energy, average power, and root mean squared value (RMS) are mutually relevant to amplitude measurements. The energy is a summation of a squared signal, the average power is the signal mean square, and the RMS is the square root of the average power[76−86]. Time-domain features are mainly extracted through direct observation and calculation of the original signal. Its advantage is that the calculation is simple and easy for researchers to understand intuitively. However, due to the non-stationarity of the EEG signal itself, individual differences and external dis-turbances are likely to affect the time-domain characteristics.
Frequency domain analysis is based on the assumption that EEG signals have stationary characteristics. The EEG signal contains a variety of frequency components. By transforming the EEG signal from the time domain to the frequency domain, the information of different frequencies is analyzed and features are extracted. For epileptic EEG signals, compared with the interictal period, the ictal EEG will have obvious characteristic waves such as sharp waves and spikes, and the signal frequency will change significantly. The frequency domain features of EEG signals include power spectral density, high-order spectrum, differential entropy, etc.[87−98]. However, due to the inherent non-stationarity and randomness of EEG signals, frequency domain analysis is limited in practical applications. Various parameter estimation methods can be used in the spectral feature extraction, and the accuracy of the parameters also affects the quality of the frequency domain features.
If the amount of information contained in the features is considered, neither the pure time-domain features nor the frequency-domain features can completely describe an EEG signal, and the EEG analysis based on the assumption of stationarity is not rigorous. Therefore, the researchers turned their attention to the time-frequency analysis method, and re-expressed the non-stationary EEG signal and extracted the corresponding features through time-frequency transformation and other means. Time-frequency analysis is mainly connected by short-time Fourier transform at the beginning, but because the size and shape of its window are fixed, it cannot be well adapted to EEG signals with fast frequency and time changes. The wavelet transform adaptively changes the shape of the time window through stretching and translation, so wavelet analysis is a representative method of time-frequency analysis. In addition to wavelet analysis, commonly used time-frequency domain analysis methods include wavelet packet decomposition, Gabor transform and so on[99−113].
Methods such as time domain, frequency domain or time-frequency analysis are mainly based on the theoretical analysis of linear systems. Since EEG signals have obvious nonlinear characteristics, processing methods for nonlinear signals are needed. Therefore, nonlinear dynamics theory is widely used in the field of EEG signal processing. Nowadays, nonlinear dynamic indicators such as approximate entropy, spectral entropy and sample entropy, etc. are commonly used in EEG signal analysis[114−124].
Table 2 summarizes the four types of features involved in the automatic detection of epilepsy in recent years and the corresponding references.
Table Infomation Is Not Enable
Epilepsy detection algorithm based on classical machine learning
In the epilepsy detection algorithms based on classical machine learning, data preprocessing of EEG signals, effective feature extraction of processed signals, and classification based on extracted features are the main tasks in the detection algorithm. Researchers have achieved automatic detection and the classification of epileptic signals by using time-frequency domain methods[125] and nonlinear methods[115,126], and achieved good performance test results. Most methods use artificial pre-designed ideas to extract features from EEG signals, such as using spectrum[127] as information from EEG signals[128] for automatic detection of epileptic seizures, which can obtain more accurate classification results. Fig. 9 shows a typical random forest for epileptic seizure detection.
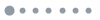
Figure 9.(Color online) A typical random forest for epileptic seizure detection.
Gotman et al.[129] selectively recorded the EEG signals during the interictal and ictal periods as samples, and used their amplitude, period and other characteristics to distinguish whether the samples were in a state of epileptic seizures. Zhang et al.[130] obtained effective features of EEG signals through frequency slice wavelet transform and achieved a classification accuracy of 98.33% using a support vector machine (SVM). Shoeb et al.[131] discussed the application of machine learning in seizure detection using the CHB-MIT dataset scalp EEG and achieved satisfactory performance. Tiwari et al.[132] used a local binary pattern (LBP) method based on key point calculations and an SVM classifier to classify epileptic seizures and no seizures and achieved an accuracy of 99.31%. Al-Hadeethi et al.[81] used the covariance matrix to reduce the dimensionality of the EEG signal, extracted its statistical features and used non-parametric tests to obtain the set with the most significant features, and used an adaptive-boosting least-squares support vector machine (AB-LS-SVM) classification model to achieve satisfactory results (>99% accuracy). Vicnesh et al.[133] extracted nonlinear features from EEG data and fed them into decision trees to classify different epilepsy categories. Wang et al.[134] proposed a three-classification algorithm based on wavelet transform and nonlinear sparse extremum learning machine (SELM), using the Daubechies wavelet to decompose the EEG data set of the University of Bonn and calculate the maximum and standard values of each sub-band, and finally got a classification accuracy of 98.4%. Sharma et al.[135] used a method for automatic detection of epileptic seizures based on time-frequency flexible wavelet transform and fractal dimension: firstly, the time-frequency flexible wavelet transform was used to decompose the EEG signal into sub-bands and calculate the fractal of each sub-band dimension, the obtained fractal dimension features were input into the least squares SVM (LS-SVM) for classification. This method achieved an accuracy rate of 98.5% for the classification of ictal and interictal periods in the epilepsy data set of the University of Bonn. Chen D et al.[136] decomposed the EEG data into 7 common wavelet families, searched in different wavelet basis functions and decomposition levels, and extracted the maximum value, mean value, variance, demeanor, skewness, and energy in each sub-band and other commonly used features, where the method achieved high accuracy (>90%) and low cost. A tool was proposed by Selvakumari et al.[137] using four features—entropy, root mean square (RMS), variance, and energy. Based on these features, the detection was done using SVM and naive Bayesian classifiers with a reported accuracy of 95.63%. Zavid and Paul[138] focused on classifying the ‘ictal’ and ‘inter-ictal’ states, where they used four features discrete cosine transformation (DCT), discrete cosine transformation-discrete wavelet transformations (DCT-DWT), singular value decomposition (SVD), and intrinsic mode function (IMF); the obtained signals are further classified by LS-SVM due to less computational cost. There are still many excellent research results, so this article will not repeat them one by one.
Table 3 summarizes the epilepsy automatic detection algorithms and corresponding references using classical machine learning in recent years.
Table Infomation Is Not Enable
Classical machine learning models mainly design effective features artificially based on signals. This stage requires researchers to have professional knowledge related to epileptic EEG signals. Although the automatic seizure detection technology based on classical machine learning has shown good accuracy using traditional signal processing (signal processing, SP) and machine learning techniques, the detection and classification of epileptic EEG signals is a difficult task. Accurately classified epileptic vs. non-ictal cases performed poorly on the three-category task of normal, ictal, and interictal[151]. This is mainly due to the following two reasons: 1) models that have obtained more accurate results for classification of binary questions are not suitable for classification of ternary questions and need to be redesigned; 2) there is less labeled data available.
Epilepsy detection algorithm based on deep learning
In recent years, with the rapid development of deep learning (DL), deep neural network (DNN) models have been widely used in various fields. As a class of machine learning methods, deep learning can automatically encode the hierarchical structure of data-independent elements and adapt to the internal structure of the data, thereby extracting deep features that are not easy to be observed and extracted. Compared with traditional feature extraction models, deep learning methods eliminate the dependence on manually extracted features. Fig. 10 shows a typical 2D-CNN for epileptic seizure detection.
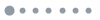
Figure 10.(Color online) A typical 2D-CNN for epileptic seizure detection.
Convolutional neural network (CNN)-like models are capable of extracting features from input data. With the continuous research of deep learning theory by researchers and the improvement of numerical computing hardware equipment, CNN has developed rapidly in recent years, and various CNN models such as AlexNet, VGG and 3D network[152] have been rapidly applied to many fields. Acharya et al.[78] implemented a 13-layer deep convolutional neural network to detect normal, pre-seizure and seizure categories. The accuracy, specificity and recall of this method were 88.67%, 90% and 95%, respectively. Jana et al.[153] proposed a combination of generating a spectrogram matrix and a one-dimensional convolutional neural network to detect epileptic seizures from EEG signals, and its accuracy demonstrated that the scheme could serve as a useful epilepsy detection technique. Avcu et al.[154] proposed a seizureNet method, which uses Fourier transform to convert the original signal into a time-frequency map, and then uses a convolutional neural network to achieve automatic detection of epilepsy. Bizopoulo et al.[155] used various networks as detection models to detect imaged EEG signals, including AlexNet, VGGNet, ResNet, DenseNet and other convolutional neural network models.
A recurrent neural network (RNN) focuses on the correlation of outputs at different times in the sequence. Among them, a long-short-term memory network (LSTM) is the most widely used model in RNN, which can fully demonstrate its superior performance in the processing of time series problems. Hu et al.[84] proposed a new method for seizure detection based on a deep two-way long short-term memory (Bi-LSTM) network, which achieved an average sensitivity of 93.61% and an average specificity of 91.85%. Thara et al.[156] used a stacked bidirectional long-short-term memory network to detect onset and interictal periods, achieving the highest accuracy of 99.08% and recall of 99.5%. The deep learning method of end-to-end learning avoids the inappropriate selection of a feature extractor and a feature subset selector to extract and select the most discriminative features, making the detection results more accurate. Roy et al.[157] adopted a 5-layer GRU network ChronoNet, and the final classification result reached 92.84%. Tsiouris et al.[158] constructed a two-layer LSTM network, using four preictal windows of different lengths for seizure prediction tasks, the LSTM model successfully predicted all 185 seizures using the previously extracted features, significantly improving the seizure prediction performance. There are still many excellent research results, so this article will not repeat them one by one.
Since the automatic detection of epileptic EEG signals has a significant effect on the clinical diagnosis of epilepsy, it is necessary to study automatic detection based on various models. Table 4 summarizes the epilepsy automatic detection algorithms and corresponding references using deep learning in recent years.
Table Infomation Is Not Enable
Epilepsy detection hardware implementation
At present, researchers have developed many complete systems for detecting and predicting epilepsy. These systems have the advantages of high accuracy and energy saving, which provide ideas and hope for the development of wearable epilepsy monitoring equipment.
Yoo et al. proposed an ultra-low power scalable EEG acquisition system on Chip (SoC) for continuous seizure detection and recording, with a fully integrated patient-specific Support Vector Machine (SVM)-based classification processor. With the SoC, a small form factor, patch-type long-term seizure monitoring and recording device can be realized. The SoC integrates eight-channel, bandwidth and gain-scalable AFE with low noise, low power instrumentation circuits, an ADC, eight-channel classification processor, and storage on a single 25 mm2 chip (0.18 μm 1P6M standard CMOS). The seizure detection processor is tested with the CHB-MIT database, and the SoC is verified with a rapid eye blink test, which shows typical accuracy of 84.4% with 2.03 μJ/classification energy efficiency[64].
Altaf et al. proposed a 16-channel noninvasive closed-loop beginning- and end-of-seizure detection SoC. The dual-channel charge recycled (DCCR) analog front end (AFE) achieves chopping and time-multiplexing an amplifier between two channels simultaneously which exploits fast-settling DC servo-loop with current consumption and NEF of 0.9 µA/channel and 3.29/channel, respectively. The dual-detector architecture (D2A) classification processor utilizes two linear support-vector machine (LSVM) classifiers based on digital hysteresis to enhance both the sensitivity and the specificity simultaneously. The pulsating voltage transcranial electrical stimulator (PVTES) automatically configures the number of pulses to control the amount of charge delivered based on skin-electrode impedance variation in efforts to suppress the seizure activity, while burning only 2.45 µW. The 25 mm2 SoC implemented in 0.18 µm CMOS consumes 2.73 µJ/classification for 16 channels with an average sensitivity, specificity, and latency of 95.7%, 98%, and 1 s, respectively[170].
Altaf et al. proposed an eight-channel patient specific scalable EEG acquisition SoC to continuously detect the patient-specific seizure electrical onset. A non-linear machine learning algorithm is employed using a 0.18 μm 1P6M standard CMOS process with an area of 25 mm2. The SoC integrates the multi-channel analog front-end with low-noise, low-power instrumentation circuits, an ADC, a classification processor, and an SRAM. The seizure detection processor is tested with CHB-MIT database, and the SoC is verified with rapid eye blink tests, which shows the sensitivity of 95.1% and 0.27 false alarm/hour while consuming 1.83 μJ/classification[171].
Lin et al. proposed a smart headband for epileptic seizure detection. The proposed headband consists of four key components: 1) an analog front-end circuitry; 2) an epileptic seizure detection tag (ESDT); 3) a bluetooth low-power chip; and 4) customized electrodes. The overall system is fully integrated into a fabric headband weighting only 50.3 g and dissipates 55.89 mW. This compact smart headband with a corresponding APP for epileptic seizure detection can mprove the patients’ quality of life by monitoring their health onditions at anytime and anywhere. The epileptic seizure detection algorithm inside ESDT is validated by using Boston Children’s Hospital’s CHB-MIT scalp EEG clinical database with the detection rate of 92.68% and the false alarm of 0.527 h−1[172].
Huang et al. proposed the first support vector machine (SVM) processor that supports on-chip active learning for seizure detection. Alternating direction method of multipliers (ADMM), minimum-redundancy maximum-relevance (mRMR), and low-rank approximation are utilized to reduce the computational complexity and memory storage by 99.4% and 90.4%, respectively. Hardware complexity is reduced by 87% through folded architecture with reconfigurable processing elements. The 4.5 mm2 SoC implemented in 40 nm CMOS, achieving the best detection performance with a 96.1% accuracy and a 0.34% false alarm rate in 0.71 s with 1.9 mW[173].
Liu et al. proposed a reconfigurable biomedical AI processor with adaptive learning. It is mainly composed of five modules: a reconfigurable neural-network engine (RNNE), various biomedical-processing engines (BPEs), an adaptive-learning engine (ALE), a reconfigurable FIR engine (RFE) and an adaptive biomedical signal compression engine (ABSCE). For an EEG seizure detection task using the Bonn database, two-stage event-driven AI processing is enabled. Compared to non-event-driven AI processing, the power consumption can be significantly reduced by 49% with accuracy of 99.84%. The 1.74 mm2 SoC implemented in 60 nm CMOS consumes 2.06 µJ/classification[174].
Zhang et al. proposed a fully programmable patient-specific closed-loop epilepsy tracking and suppression SoC. The proposed two-cycle analog front end (2C-AFE) obtains a 9.8-b effective number of bits (ENOB) with 8 × capacitive digital-to-analog converter (CAPDAC) area reduction and 4 × switching energy saving compared to a conventional 10-b SAR with an identical unit capacitor size. The entire SoC with 16 surface EEG recording channels consumes an ultra-low energy of 0.97 µJ/class and occupies a miniaturized area of 0.13 mm2/ch in 40 nm CMOS, achieving real-time concurrent seizure detection and raw EEG recording. Verified with the CHB-MIT database, the guided time-channel averaging (GTCA) neural processor achieves the vector-based sensitivity, the specificity, and the latency of 97.8%, 99.5%, and <1 s, respectively[175].
Zhu et al. proposed a model for seizure detection based on 1D-CNN, which loses only a small amount of accuracy but greatly reduces computational complexity and model size. Furthermore, a low power consumption and high efficiency based on 1D-CNN hardware accelerator architecture is proposed. It only takes 170 μs to perform a round of inference on Xilinx ZC706 evaluation platform at 200 MHz, which is much more efficient than other hardware network accelerators[176].
Chua et al. proposed SOUL: Stochastic-gradientdescent-based Online Unsupervised Logistic regression classifier. After an initial offline training phase, continuous online unsupervised classifier updates are applied in situ, which improves sensitivity in patients with drifting seizure features. SOUL was tested on two human EEG datasets: the Children’s Hospital Boston and the Massachusetts Institute of Technology (CHB-MIT) scalp EEG dataset and a long (>100 h) intracranial EEG dataset. It was able to achieve an average sensitivity of 97.5% and 97.9% for the two datasets, respectively, at >95% specificity. Sensitivity improved by at most 8.2% on long-term data when compared to a typical seizure detection classifier. SOUL was fabricated in Taiwan Semiconductor Manufacturing Company (TSMC’s) 28 nm process occupying 0.1 mm2 and achieves 1.5 nJ/classification energy efficiency, which is at least 24 × more efficient than state-of-the-art[177].
Li et al. proposed a novel low-latency parallel Convolutional Neural Network (CNN) architecture that has between 2-2800 × fewer network parameters compared to state-of-the-art (SOTA) CNN architectures and achieves 5-fold cross validation accuracy of 99.84% for epileptic seizure detection, and 99.01% and 97.54% for epileptic seizure prediction, when evaluated using the University of Bonn EEG, CHB-MIT and SWEC-ETHZ seizure datasets, respectively. They investigated the effects of non-idealities on our system and investigate quantization aware training (QAT) to mitigate the performance degradation due to low analog-to-digital converter (ADC)/digital-to-analog converter (DAC) resolution. Finally, we propose a stuck weight offsetting methodology to mitigate performance degradation due to stuck RON/ROFF memristor weights, recovering up to 32% accuracy, without requiring retraining. The CNN component of their platform is estimated to consume approximately 2.791 W of power while occupying an area of 31.255 mm2 in a 22 nm FDSOI CMOS process[178]. Table 5 summaries the performance of existing seizure detection/prediction systems.
Table Infomation Is Not Enable
Conclusion and outlook
Automatic detection of epilepsy, that is, automatic identification of epileptic seizures, can greatly reduce the burden on medical workers and reduce the uncertainty of doctors' subjective judgment of the disease. Since EEG is a low-cost, easy-to-acquire and non-invasive clinical physiological signal, EEG-based automatic detection of epilepsy is an effective means to improve the efficiency of epilepsy detection and treatment. This paper first systematically expounds the process of automatic detection of epilepsy based on EEG, and conducts a detailed and comprehensive investigation and summary of the specific methods involved in each step.
According to the above research and analysis, combined with the actual application scenario requirements of EEG-based automatic epilepsy detection, this paper looks forward to the future research direction from the following aspects:
(1) The low-power design of wearable devices is the general trend. It is necessary to study circuit design schemes suitable for different processes at lower power supply voltages, and reduce the demand for low-pass filters by optimizing the bandwidth of the front-end amplifier, thereby saving power consumption. Hardware performance such as common-mode rejection ratio, analog-to-digital conversion accuracy and speed, and dynamic range is the guarantee for the accuracy of EEG acquisition signals and the basis for subsequent EEG signal processing and criteria. For dry electrodes and non-contact electrode systems, motion artifacts will seriously affect the normal operation of the AFE circuit, and it is necessary to study the analog circuit suppression method for motion artifacts combined with electrode impedance detection technology.
(2) When using traditional machine learning methods, extracting effective classification features is the key to epilepsy detection. Seizure detection by traditional methods has achieved a high level of detection, but how to distinguish epilepsy subtypes, how to distinguish between onset and interictal periods to realize the prediction of epilepsy, is still a problem worth exploring.
(3) In recent years, with the rapid development of deep learning, a large number of automatic detection methods based on deep learning have been widely used in automatic detection of epilepsy, and achieved good results. However, this method also has many challenges. a) Deep learning often relies on a large amount of data, but limited by the data set in actual research, it is difficult to obtain a large number of training samples, which will have a great impact on the accuracy and robustness of the model. b) The existing public datasets are almost all EEG signal fragments, which are different from the continuous real-time signals in the actual scene. When solving practical problems, the neural network model trained with EEG fragment signals may not be well adapted to the real scene. c) Due to the limited computing resources in actual use, lightweight neural network models will be more practical.
(4) With the continuous development of wearable devices, in addition to the existing feature extraction for EEG signals, the fusion of other physiological features also has considerable research value. Previous studies have shown that blood oxygen saturation is related to the termination of epileptic seizures[179]. Combining such other characteristic indicators with EEG, and using machine learning methods such as multi-view for automatic detection of epilepsy, is expected to further improve the detection accuracy.
References
[1] A J Casson, D C Yates, S J M Smith et al. Wearable electroencephalography. IEEE Eng Med Biol Mag, 29, 44(2010).
[2] J R Stevens. Seizure occurrence and interspike interval. Arch Neurol, 26, 409(1972).
[3] S Noachtar, J Rémi. The role of EEG in epilepsy: A critical review. Epilepsy Behav, 15, 22(2009).
[4] M Piccolino, M Bresadola. Drawing a spark from darkness: John Walsh and electric fish. Trends Neurosci, 25, 51(2002).
[5] M Z Ilyas, P Saad, M I Ahmad. A survey of analysis and classification of EEG signals for brain-computer interfaces, 1(2015).
[6] W J R Dunseath, E F Kelly. Multichannel PC-based data-acquisition system for high-resolution EEG. IEEE Trans Biomed Eng, 42, 1212(1995).
[7] W Pribyl, H Hadl. 32+32+8 channel data acquisition system for bio-signals in routine and research applications, 2685(1992).
[8] E Ratti, S Waninger, C Berka et al. Comparison of medical and consumer wireless EEG systems for use in clinical trials. Front Hum Neurosci, 11, 398(2017).
[9] J W Xu, S Mitra, A Matsumoto et al. A wearable 8-channel active-electrode EEG/ETI acquisition system for body area networks. IEEE J Solid-State Circuits, 49, 2005(2014).
[10] J W Xu, B Büsze, C Van Hoof et al. A 15-channel digital active electrode system for multi-parameter biopotential measurement. IEEE J Solid-State Circuits, 50, 2090(2015).
[11] K A Ng, Y P Xu. A low-power, high CMRR neural amplifier system employing CMOS inverter-based OTAs with CMFB through supply rails. IEEE J Solid-State Circuits, 51, 724(2016).
[12] S Y Park, J Cho, K Na et al. Modular 128-channel Δ-Δσ analog front-end architecture using spectrum equalization scheme for 1024-channel 3-D neural recording microsystems. IEEE J Solid-State Circuits, 53, 501(2018).
[13] A Karimi-Bidhendi, O Malekzadeh-Arasteh, M C Lee et al. CMOS ultralow power brain signal acquisition front-ends: Design and human testing. IEEE Trans Biomed Circuits Syst, 11, 1111(2017).
[14] H Wang, P P Mercier. A current-mode capacitively-coupled chopper instrumentation amplifier for biopotential recording with resistive or capacitive electrodes. IEEE Trans Circuits Syst II Express Briefs, 65, 699(2018).
[15] C Y Wu, C H Cheng, Z X Chen. A 16-channel CMOS chopper-stabilized analog front-end ECoG acquisition circuit for a closed-loop epileptic seizure control system. IEEE Trans Biomed Circuits Syst, 12, 543(2018).
[16] M Tohidi, J K Madsen, M J R Heck et al. A low-power analog front-end neural acquisition design for seizure detection, 1(2016).
[17] J W Zheng, W H Ki, L Y Hu et al. Chopper capacitively coupled instrumentation amplifier capable of handling large electrode offset for biopotential recordings. IEEE Trans Circuits Syst II Express Briefs, 64, 1392(2017).
[18] J W Zheng, W H Ki, C Y Tsui. A fully integrated analog front end for biopotential signal sensing. IEEE Trans Circuits Syst I Regul Pap, 65, 3800(2018).
[19] P Tallgren, S Vanhatalo, K Kaila et al. Evaluation of commercially available electrodes and gels for recording of slow EEG potentials. Clin Neurophysiol, 116, 799(2005).
[20] P Griss, P Enoksson, H K Tolvanen-Laakso et al. Micromachined electrodes for biopotential measurements. J Microelectromechanical Syst, 10, 10(2001).
[21] Y J Huang, C Y Wu, A M K Wong et al. Novel active comb-shaped dry electrode for EEG measurement in hairy site. IEEE Trans Biomed Eng, 62, 256(2015).
[22] L D Liao, I J Wang, S F Chen et al. Design, fabrication and experimental validation of a novel dry-contact sensor for measuring electroencephalography signals without skin preparation. Sensors, 11, 5819(2011).
[23] C J Harland, T D Clark, R J Prance. Remote detection of human electroencephalograms using ultrahigh input impedance electric potential sensors. Appl Phys Lett, 81, 3284(2002).
[24] T J Sullivan, S R Deiss, G Cauwenberghs. A low-noise, non-contact EEG/ECG sensor, 154(2008).
[25] B Renshaw, A Forbes, B R Morison. Activity of isocortex and hippocampus: Electrical studies with micro-electrodes. J Neurophysiol, 3, 74(1940).
[26] B L McNaughton, J O'Keefe, C A Barnes. The stereotrode: A new technique for simultaneous isolation of several single units in the central nervous system from multiple unit records. J Neurosci Methods, 8, 391(1983).
[27] K A Moxon, S C Leiser, G A Gerhardt et al. Ceramic-based multisite electrode arrays for chronic single-neuron recording. IEEE Trans Biomed Eng, 51, 647(2004).
[28] T Adrega, S P Lacour. Stretchable gold conductors embedded in PDMS and patterned by photolithography: Fabrication and electromechanical characterization. J Micromech Microeng, 20, 055025(2010).
[29] J G Webster, J W Clark. Medical instrumentation: application and design. John Wiley & Sons(2010).
[30] J C Huhta, J G Webster. 60-HZ interference in electrocardiography. IEEE Trans Biomed Eng, 20, 91(1973).
[31] E S Winokur, M K Delano, C G Sodini. A wearable cardiac monitor for long-term data acquisition and analysis. IEEE Trans Biomed Eng, 60, 189(2013).
[32] E M Spinelli, M A Mayosky. Two-electrode biopotential measurements: Power line interference analysis. IEEE Trans Biomed Eng, 52, 1436(2005).
[33] R E Serrano, M Gasulla, O Casas et al. Power line interference in ambulatory biopotential measurements. Proceedings of the 25th Annual International Conference of the IEEE Engineering in Medicine and Biology Society (IEEE Cat. No.03CH37439), Cancun, 3024(2003).
[34] Y Higashi, Y Yokota, Y Naruse. Signal correlation between wet and original dry electrodes in electroencephalogram according to the contact impedance of dry electrodes, 1062(2017).
[35] M Guermandi, E F Scarselli, R Guerrieri. A driving right leg circuit (DgRL) for improved common mode rejection in bio-potential acquisition systems. IEEE Trans Biomed Circuits Syst, 10, 507(2016).
[36] J A Costa, T C Pimenta. CMOS analog front-end IC for EEG applications with high powerline interference rejection, 1(2018).
[37] R Kaur, R Malhotra, S Deb. MAC based FIR filter: A novel approach for low-power real-time de-noising of ECG signals, 1(2015).
[38] Y Liu, F L An, X Lang et al. Remove motion artifacts from scalp single channel EEG based on noise assisted least square multivariate empirical mode decomposition, 568(2020).
[39] A Bakker, J Huijsing. High-accuracy CMOS smart temperature sensors. Springer(2000).
[40] A Jain, K Kandpal. Design of a high gain, temperature compensated biomedical instrumentation amplifier for EEG applications, 292(2017).
[41] G C Huang, T Yin, Q S Wu et al. A 1.3 μW 0.7 μRMS chopper current-reuse instrumentation amplifier for EEG applications, 2624(2015).
[42] Y T Dong, L H Tang, X L Yang et al. A 1.8 μW 32 nV/√Hz current-reuse capacitively-coupled instrumentation amplifier for EEG detection, 1(2017).
[43] C J Lee, J I Song. A chopper stabilized current-feedback instrumentation amplifier for EEG acquisition applications. IEEE Access, 7, 11565(2019).
[44] Z Hoseini, M Nazari, K S Lee et al. Current feedback instrumentation amplifier with built-In differential electrode offset cancellation loop for ECG/EEG sensing frontend. IEEE Trans Instrum Meas, 70, 1(2021).
[45] Y Z Zhou, M L Zhao, Y T Dong et al. A low-power low-noise biomedical instrumentation amplifier using novel ripple-reduction technique, 1(2018).
[46] R Chebli, M Sawan. Chopped logarithmic programmable gain amplifier intended to EEG acquisition interface, 1(2014).
[47] B Babušiak, Š Borik. Bio-Amplifier with programmable gain and adjustable leads, 616(2013).
[48] I Y AbuShawish, S A Mahmoud. Digitally programmable gain and tunable band-width DPOTA based bio-medical amplifier, 147(2021).
[49] N Marchon, G Naik. A novel linear phase FIR high pass filter for biomedical signals, 147(2019).
[50] I Winkler, S Debener, K R Müller et al. On the influence of high-pass filtering on ICA-based artifact reduction in EEG-ERP, 4101(2015).
[51] A Abdallah, M Diab, S Mahmoud. A micropower EEG detection system applicable for paralyzed hand artifical control, 411(2017).
[52] K N Wang, C H Chang, M Onabajo. A fully-differential CMOS low-pass Notch filter for biosignal measurement devices with high interference rejection, 1041(2014).
[53] A Kumar Sahu, A Kumar Sahu. A review on different filter design techniques and topologies for bio-potential signal acquisition systems, 934(2019).
[54] G K Soni, H Singh, H Arora et al. Ultra low power CMOS low pass filter for biomedical ECG/EEG application, 558(2020).
[55] X L Yang, Y Zhou, M L Zhao et al. A 12b 238kS/s SAR ADC with novel built-in digital calibration method for EEG acquisition applications, 1(2015).
[56] X L Yang, M L Zhao, Y T Dong et al. A 14.9μW analog front-end with capacitively-coupled instrumentation amplifier and 14-bit SAR ADC for epilepsy diagnosis system, 268(2017).
[57] H França, M Ataei, A Boegli et al. A 100nW 10-bit 400S/s SAR ADC for ultra low-power bio-sensing applications, 1(2017).
[58] W C Lai. SAR ADC with optical frontend for biosensor applications, 1(2021).
[59] R Arif, S K Wijaya et al. Design of EEG data acquisition system based on Raspberry Pi 3 for acute ischemic stroke identification, 271(2018).
[60] R Martins, S Selberherr, FA Vaz. A CMOS IC for portable EEG acquisition systems. IEEE Transactions on Instrumentation and Measurement, 47, 1191(1998).
[61] C L Qian, J Parramon, E Sanchez-Sinencio. A micropower low-noise neural recording front-end circuit for epileptic seizure detection. IEEE J Solid-State Circuits, 46, 1392(2011).
[62] S Robinet, P Audebert, G Regis et al. A low-power 0.7 μVrms 32-channel mixed-signal circuit for ECoG recordings. IEEE J Emerg Sel Topics Circuits Syst, 1, 451(2011).
[63] H Y Zhou, M Voelker, J Hauer. A mixed-signal front-end ASIC for EEG acquisition system, 649(2013).
[64] J Yoo, L Yan, D El-Damak et al. An 8-channel scalable EEG acquisition SoC with patient-specific seizure classification and recording processor. IEEE J Solid-State Circuits, 48, 214(2013).
[65] R Muller, H P Le, W Li et al. A minimally invasive 64-channel wireless μECoG implant. IEEE J Solid-State Circuits, 50, 344(2015).
[66] W A Smith, B J Mogen, E E Fetz et al. Exploiting electrocorticographic spectral characteristics for optimized signal chain design: A 1.08 W analog front end with reduced ADC resolution requirements. IEEE Trans Biomed Circuits Syst, 10, 1171(2016).
[67] M Tohidi, J Kargaard Madsen, F Moradi. Low-power high-input-impedance EEG signal acquisition SoC with fully integrated IA and signal-specific ADC for wearable applications. IEEE Trans Biomed Circuits Syst, 13, 1437(2019).
[68] T Tang, W L Goh, L Yao et al. A TDM-based 16-channel AFE ASIC with enhanced system-level CMRR for wearable EEG recording with dry electrodes. IEEE Trans Biomed Circuits Syst, 14, 516(2020).
[69] D Gao, L X Liu. A two-stage time-division multiplexing AFE with input impedance boosting DDA for EEG signal acquisition, 1(2021).
[70] C W Huang, J J Wang, C C Hung et al. Design of CMOS analog front-end electroencephalography (EEG) amplifier with ±1-V common-mode and ±10-mV differential-mode artifact removal, 714(2022).
[71] A L Goldberger, L A N Amaral, L Glass et al. PhysioBank, PhysioToolkit, and PhysioNet. Circulation, 101, e215(2000).
[72] A Burrello, L Cavigelli, K Schindler et al. Laelaps: an energy-efficient seizure detection algorithm from long-term human iEEG recordings without false alarms, 752(2019).
[73] R G Andrzejak, K Schindler, C Rummel. Nonrandomness, nonlinear dependence, and nonstationarity of electroencephalographic recordings from epilepsy patients. Phys Rev E, 86, 046206(2012).
[74] R San-Segundo, M Gil-Martín, L F D'Haro-Enríquez et al. Classification of epileptic EEG recordings using signal transforms and convolutional neural networks. Comput Biol Med, 109, 148(2019).
[76] A K Jaiswal, H Banka. Epileptic seizure detection in EEG signal with GModPCA and support vectormachine. Bio Med Mater Eng, 28, 141(2017).
[77] A K Jaiswal, H Banka. Epileptic seizure detection in EEG signal using machine learning techniques. Australas Phys Eng Sci Med, 41, 81(2018).
[78] U Acharya, S L Oh, Y Hagiwara et al. Deep convolutional neural network for the automated detection and diagnosis of seizure using EEG signals. Comput Biol Med, 100, 270(2018).
[79] A Sharmila, P Geethanjali. Effect of filtering with time domain features for the detection of epileptic seizure from EEG signals. J Med Eng Technol, 42, 217(2018).
[80] J Saini, M Dutta. Epilepsy classification using optimized artificial neural network. Neurol Res, 40, 982(2018).
[81] H Al-Hadeethi, S Abdulla, M Diykh et al. Adaptive boost LS-SVM classification approach for time-series signal classification in epileptic seizure diagnosis applications. Expert Syst Appl, 161, 113676(2020).
[82] A Eltrass, M Tayel, A F EL-qady. Automatic epileptic seizure detection approach based on multi-stage Quantized Kernel Least Mean Square filters. Biomed Signal Process Control, 70, 103031(2021).
[83] M Zeng, X N Zhang, C Y Zhao et al. GRP-DNet: A gray recurrence plot-based densely connected convolutional network for classification of epileptiform EEG. J Neurosci Methods, 347, 108953(2021).
[84] X M Hu, S S Yuan, F Z Xu et al. Scalp EEG classification using deep Bi-LSTM network for seizure detection. Comput Biol Med, 124, 103919(2020).
[85] A Quintero-Rincón, C D'Giano, H Batatia. A quadratic linear-parabolic model-based EEG classification to detect epileptic seizures. J Biomed Res, 34, 205(2020).
[86] Z Gao, G L Lu, P Yan et al. Automatic change detection for real-time monitoring of EEG signals. Front Physiol, 9, 325(2018).
[87] Y Li, X D Wang, M L Luo et al. Epileptic seizure classification of EEGs using time–frequency analysis based multiscale radial basis functions. IEEE J Biomed Health Inform, 22, 386(2018).
[88] H Choubey, A Pandey. A new feature extraction and classification mechanisms For EEG signal processing. Multidimens Syst Signal Process, 30, 1793(2019).
[89] V Gupta, R B Pachori. Epileptic seizure identification using entropy of FBSE based EEG rhythms. Biomed Signal Proces, 53, 101569(2019).
[90] J A de la O Serna, M R A Paternina, A Zamora-Méndez et al. EEG-rhythm specific Taylor–Fourier filter bank implemented with O-splines for the detection of epilepsy using EEG signals. IEEE Sens J, 20, 6542(2020).
[91] P Mathur, V K Chakka, S B Shah. Ramanujan periodic subspace based epileptic EEG signals classification. IEEE Sens Lett, 5, 1(2021).
[92] J Y Na, Z P Wang, S Q Lv et al. An extended K nearest neighbors-based classifier for epilepsy diagnosis. IEEE Access, 9, 73910(2021).
[93] H Pal, A Kumar. Stability analysis of multiscale bubble entropy and power metric based seizure detection technique with MLA. IETE J Res, 69, 3455(2023).
[94] Z P Wang, J Y Na, B Y Zheng. An improved kNN classifier for epilepsy diagnosis. IEEE Access, 8, 100022(2020).
[95] A Mansouri, S P Singh, K Sayood. Online EEG seizure detection and localization. Algorithms, 12, 176(2019).
[96] J Birjandtalab, M Baran Pouyan, D Cogan et al. Automated seizure detection using limited-channel EEG and non-linear dimension reduction. Comput Biol Med, 82, 49(2017).
[97] J Zhang, Z C Wei, J Z Zou et al. Automatic epileptic EEG classification based on differential entropy and attention model. Eng Appl Artif Intell, 96, 103975(2020).
[98] T Iešmantas, R Alzbutas. Convolutional neural network for detection and classification of seizures in clinical data. Med Biol Eng Comput, 58, 1919(2020).
[99] A Sharmila, P Mahalakshmi. Wavelet-based feature extraction for classification of epileptic seizure EEG signal. J Med Eng Technol, 41, 670(2017).
[100] Q Liu, X G Zhao, Z G Hou et al. Epileptic seizure detection based on the kernel extreme learning machine. Technol Health Care, 25, 399(2017).
[101] R R Sharma, P Varshney, R B Pachori et al. Automated system for epileptic EEG detection using iterative filtering. IEEE Sens Lett, 2, 1(2018).
[102] M G Tsipouras. Spectral information of EEG signals with respect to epilepsy classification. EURASIP J Adv Signal Process, 2019, 1(2019).
[103] C Mahjoub, R Le Bouquin Jeannès, T Lajnef et al. Epileptic seizure detection on EEG signals using machine learning techniques and advanced preprocessing methods. Biomedical Engineering / Biomedizinische Technik, 65, 1(2019).
[104] H S Chiang, M Y Chen, Y J Huang. Wavelet-based EEG processing for epilepsy detection using fuzzy entropy and associative petri net. IEEE Access, 7, 103255(2019).
[105] S N Chen, X Zhang, L L Chen et al. Automatic diagnosis of epileptic seizure in electroencephalography signals using nonlinear dynamics features. IEEE Access, 7, 61046(2019).
[106] I Aliyu, C G Lim. Selection of optimal wavelet features for epileptic EEG signal classification with LSTM. Neural Comput & Applic, 35, 1077(2023).
[107] A Anuragi, D Singh Sisodia, R B Pachori. Epileptic-seizure classification using phase-space representation of FBSE-EWT based EEG sub-band signals and ensemble learners. Biomed Signal Process Control, 71, 103138(2022).
[108] S R Ashokkumar, S Anupallavi, M Premkumar et al. Implementation of deep neural networks for classifying electroencephalogram signal using fractional S-transform for epileptic seizure detection. Int J Imaging Syst Tech, 31, 895(2021).
[109] S R Ashokkumar, G MohanBabu, S Anupallavi. A novel two-band equilateral wavelet filter bank method for an automated detection of seizure from EEG signals. Int J Imaging Syst Tech, 30, 978(2020).
[110] S Nasiri, G D Clifford. Generalizable seizure detection model using generating transferable adversarial features. IEEE Signal Process Lett, 28, 568(2021).
[111] K Zeng, G X Ouyang, H Chen et al. Characterizing dynamics of absence seizure EEG with spatial-temporal permutation entropy. Neurocomputing, 275, 577(2018).
[112] Q Y Zhan, W Hu. An epilepsy detection method using multiview clustering algorithm and deep features. Comput Math Methods Med, 2020, 1(2020).
[113] J W Mu, L Y Dai, J X Liu et al. Automatic detection for epileptic seizure using graph-regularized nonnegative matrix factorization and Bayesian linear discriminate analysis. Biocybern Biomed Eng, 41, 1258(2021).
[114] Z Mohammadpoory, M Nasrolahzadeh, J Haddadnia. Epileptic seizure detection in EEGs signals based on the weighted visibility graph entropy. Seizure, 50, 202(2017).
[115] P Li, C Karmakar, J Yearwood et al. Detection of epileptic seizure based on entropy analysis of short-term EEG. PLoS One, 13, e0193691(2018).
[116] S Lahmiri, A Shmuel. Accurate classification of seizure and seizure-free intervals of intracranial EEG signals from epileptic patients. IEEE Trans Instrum Meas, 68, 791(2019).
[117] A Attia, A Moussaoui, Y Chahir. Epileptic seizures identification with autoregressive model and firefly optimization based classification. Evol Syst, 12, 827(2021).
[118] Z Brari, S Belghith. A novel machine learning model for the detection of epilepsy and epileptic seizures using electroencephalographic signals based on chaos and fractal theories. Math Probl Eng, 2021, 1(2021).
[119] X Z Gao, X Y Yan, P Gao et al. Automatic detection of epileptic seizure based on approximate entropy, recurrence quantification analysis and convolutional neural networks. Artificial intelligence in medicine, 102, 101711(2019).
[120] A Goshvarpour, A Goshvarpour. Diagnosis of epileptic EEG using a lagged Poincare plot in combination with the autocorrelation. Signal Image and Video Processing, 14, 1309(2020).
[121] D Priyasad, T Fernando, S Denman et al. Interpretable seizure classification using unprocessed EEG with multi-channel attentive feature fusion. IEEE Sens J, 21, 19186(2021).
[122] N Mahmoodian, A Boese, M Friebe et al. Epileptic seizure detection using cross-bispectrum of electroencephalogram signal. Seizure, 66, 4(2019).
[123] X Lu, J Zhang, S Huang et al. Detection and classification of epileptic EEG signals by the methods of nonlinear dynamics. Chaos Solitons & Fractals, 151, 111032(2021).
[124] M S J Solaija, S Saleem, K Khurshid et al. Dynamic mode decomposition based epileptic seizure detection from scalp EEG. IEEE Access, 6, 38683(2018).
[125] O Faust, U R Acharya, H Adeli et al. Wavelet-based EEG processing for computer-aided seizure detection and epilepsy diagnosis. Seizure, 26, 56(2015).
[126] Y L Zhang, W D Zhou, S S Yuan et al. Seizure detection method based on fractal dimension and gradient boosting. Epilepsy Behav, 43, 30(2015).
[127] T Alexandros, G Markos, G Dimitrios et al. Automated epileptic seizure detection methods: A review study. Epilepsy–Histological, Electroencephalographic and Psychological Aspects, 2027(2012).
[128] M T Salam, M Sawan, D K Nguyen. Epileptic seizure onset detection prior to clinical manifestation, 6210(2010).
[129] J Gotman, L Y Wang. State-dependent spike detection: Concepts and preliminary results. Electroencephalogr Clin Neurophysiol, 79, 11(1991).
[130] T Zhang, W Z Chen, M Y Li. Automatic seizure detection of electroencephalogram signals based on frequency slice wavelet transform and SVM. Acta Phys Sin, 65, 038703(2016).
[131] A H Shoeb. Application of machine learning to epileptic seizure onset detection and treatment. Massachusetts Institute of Technology, 1, 1(2009).
[132] A K Tiwari, R B Pachori, V Kanhangad et al. Automated diagnosis of epilepsy using key-point-based local binary pattern of EEG signals. IEEE J Biomed Health Inform, 21, 888(2017).
[133] J Vicnesh, Y Hagiwara. Accurate detection of seizure using nonlinear parameters extracted from eeg signals. J Mech Med Biol, 19, 1940004(2019).
[134] Y F Wang, Z C Li, L C Feng et al. Automatic detection of epilepsy and seizure using multiclass sparse extreme learning machine classification. Comput Math Methods Med, 2017, 1(2017).
[135] M Sharma, R B Pachori, U Rajendra Acharya. A new approach to characterize epileptic seizures using analytic time-frequency flexible wavelet transform and fractal dimension. Pattern Recognit Lett, 94, 172(2017).
[136] D Chen, S R Wan, J Xiang et al. A high-performance seizure detection algorithm based on Discrete Wavelet Transform (DWT) and EEG. PLoS One, 12, e0173138(2017).
[137] R S Selvakumari, M Mahalakshmi, P Prashalee. Patient-specific seizure detection method using hybrid classifier with optimized electrodes. J Med Syst, 43, 121(2019).
[138] M Z Parvez, M Paul. Epileptic seizure detection by analyzing EEG signals using different transformation techniques. Neurocomputing, 145, 190(2014).
[139] L Guo, D Rivero, J Dorado et al. Automatic epileptic seizure detection in EEGs based on line length feature and artificial neural networks. J Neurosci Methods, 191, 101(2010).
[140] Q Yuan, W D Zhou, L R Zhang et al. Epileptic seizure detection based on imbalanced classification and wavelet packet transform. Seizure, 50, 99(2017).
[141] S Raghu, N Sriraam. Classification of focal and non-focal EEG signals using neighborhood component analysis and machine learning algorithms. Expert Syst Appl, 113, 18(2018).
[142] O K Fasil, R Rajesh. Time-domain exponential energy for epileptic EEG signal classification. Neurosci Lett, 694, 1(2019).
[143] E Alickovic, J Kevric, A Subasi. Performance evaluation of empirical mode decomposition, discrete wavelet transform, and wavelet packed decomposition for automated epileptic seizure detection and prediction. Biomed Signal Process Control, 39, 94(2018).
[144] K D Tzimourta, A T Tzallas, N Giannakeas et al. A robust methodology for classification of epileptic seizures in EEG signals. Health Technol, 9, 135(2019).
[145] J Birjandtalab, V N Jarmale, M Nourani et al. Imbalance learning using neural networks for seizure detection, 1(2018).
[146] X S Wang, G H Gong, N Li et al. Detection analysis of epileptic EEG using a novel random forest model combined with grid search optimization. Front Hum Neurosci, 13, 52(2019).
[147] A Y Yan, W D Zhou, Q Yuan et al. Automatic seizure detection using Stockwell transform and boosting algorithm for long-term EEG. Epilepsy Behav, 45, 8(2015).
[148] M Mursalin, S S Islam, M K Noman et al. Epileptic seizure classification using statistical sampling and a novel feature selection algorithm(2019).
[149] M K Siddiqui, M Z Islam, M A Kabir. Analyzing performance of classification techniques in detecting epileptic seizure. International Conference on Advanced Data Mining and Applications. ADMA 2017. Lecture Notes in Computer Science. Springer, Cham, 10604, 386(2017).
[150] M Mursalin, Y Zhang, Y H Chen et al. Automated epileptic seizure detection using improved correlation-based feature selection with random forest classifier. Neurocomputing, 241, 204(2017).
[151] H Rajaguru, S K Prabhakar. Multilayer autoencoders and EM-PCA with genetic algorithm for epilepsy classification from EEG, 353(2018).
[152] S W Ji, W Xu, M Yang et al. 3D convolutional neural networks for human action recognition. IEEE Trans Pattern Anal Mach Intell, 35, 221(2013).
[153] G C Jana, R Sharma, A Agrawal. A 1D-CNN-spectrogram based approach for seizure detection from EEG signal. Procedia Comput Sci, 167, 403(2020).
[154] M T Avcu, Z Zhang, D W Shih Chan. Seizure detection using least eeg channels by deep convolutional neural network. ICASSP 2019 - 2019 IEEE International Conference on Acoustics, Speech and Signal Processing (ICASSP), 1120(2019).
[155] P Bizopoulos, G I Lambrou, D Koutsouris. Signal2Image modules in deep neural networks for EEG classification, 702(2019).
[156] D K Thara, B G PremaSudha, F Xiong. Epileptic seizure detection and prediction using stacked bidirectional long short term memory. Pattern Recognit Lett, 128, 529(2019).
[157] S Roy, I Kiral-Kornek, S Harrer. ChronoNet: A deep recurrent neural network for abnormal EEG identification. Conference on Artificial Intelligence in Medicine in Europe. Springer, 47(2019).
[158] K M Tsiouris, V C Pezoulas, M Zervakis et al. A Long Short-Term Memory deep learning network for the prediction of epileptic seizures using EEG signals. Comput Biol Med, 99, 24(2018).
[159] R Akut. Wavelet based deep learning approach for epilepsy detection. Health Inf Sci Syst, 7, 1(2019).
[160] R H Gabr, A I Shahin, A A Sharawi et al. A deep learning identification system for different epileptic seizure disease stages. J Eng Appl Sci, 67, 925(2020).
[161] B C Zhang, W N Wang, Y T Xiao et al. Cross-subject seizure detection in EEGs using deep transfer learning. Comput Math Methods Med, 2020, 1(2020).
[162] B Akbarian, A Erfanian. A framework for seizure detection using effective connectivity, graph theory, and multi-level modular network. Biomed Signal Process Control, 59, 101878(2020).
[163] S Hadiyoso, I Wijayanto, A Humairani. Signal dynamics analysis for epileptic seizure classification on EEG signals. Traitement Du Signal, 38, 73(2021).
[164] Y N Zhao, C X Dong, G B Zhang et al. EEG-Based Seizure detection using linear graph convolution network with focal loss. Comput Methods Programs Biomed, 208, 106277(2021).
[165] S W Jang, S H Lee. Detection of epileptic seizures using wavelet transform, peak extraction and PSR from EEG signals. Symmetry, 12, 1239(2020).
[166] M N Ma, Y L Cheng, X H Wei et al. Research on epileptic EEG recognition based on improved residual networks of 1-D CNN and indRNN. BMC Med Informat Decis Making, 21, 1(2021).
[167] H S Nogay, H Adeli. Detection of epileptic seizure using pretrained deep convolutional neural network and transfer learning. Eur Neurol, 83, 602(2021).
[168] A Yildiz, H S Zan, S Said. Classification and analysis of epileptic EEG recordings using convolutional neural network and class activation mapping. Biomed Signal Process Control, 68, 102720(2021).
[169] L F Sui, X Y Zhao, Q B Zhao et al. Localization of epileptic foci by using convolutional neural network based on iEEG. Artificial Intelligence Applications and Innovations, Hersonissos, Crete(2019).
[170] M A Bin Altaf, C Zhang, J Yoo. A 16-channel patient-specific seizure onset and termination detection SoC with impedance-adaptive transcranial electrical stimulator. IEEE J Solid-State Circuits, 50, 2728(2015).
[171] M A Bin Altaf, J Yoo. A 1.83-J/classification, 8-channel, patient-specific epileptic seizure classification SoC using a non-linear support vector machine. IEEE Trans Biomed Circuits Syst, 10, 49(2016).
[172] S K Lin, L C Istiqomah et al. An ultra-low power smart headband for real-time epileptic seizure detection. IEEE J Transl Eng Heath Med, 6, 1(2018).
[173] S A Huang, K C Chang, H H Liou et al. A 1.9-mW SVM processor with on-chip active learning for epileptic seizure control. IEEE J Solid-State Circuits, 55, 452(2020).
[174] J H Liu, Z Zhu, Y Zhou et al. 4.5 BioAIP: A reconfigurable biomedical AI processor with adaptive learning for versatile intelligent health monitoring, 62(2021).
[175] M L Zhang, L Zhang, C W Tsai et al. A patient-specific closed-loop epilepsy management SoC with one-shot learning and online tuning. IEEE J Solid-State Circuits, 57, 1049(2022).
[176] L S Zhu, D S Liu, X H Li et al. An Efficient Hardware Architecture for Epileptic Seizure Detection using EEG Signals based on 1D-CNN, 1(2021).
[177] A Chua, M I Jordan, R Muller. SOUL: An energy-efficient unsupervised online learning seizure detection classifier. IEEE J Solid-State Circuits, 57, 2532(2022).
[178] C Q Li, C Lammie, X N Dong et al. Seizure detection and prediction by parallel memristive convolutional neural networks. IEEE Trans Biomed Circuits Syst, 16, 609(2022).
[179] D M Goldenholz, A Kuhn, A Austermuehle et al. Long-term monitoring of cardiorespiratory patterns in drug-resistant epilepsy. Epilepsia, 58, 77(2017).