Chang Qiao, Haoyu Chen, Run Wang, Tao Jiang, Yuwang Wang, Dong Li, "Deep learning-based optical aberration estimation enables offline digital adaptive optics and super-resolution imaging," Photonics Res. 12, 474 (2024)

Search by keywords or author
- Photonics Research
- Vol. 12, Issue 3, 474 (2024)
Abstract
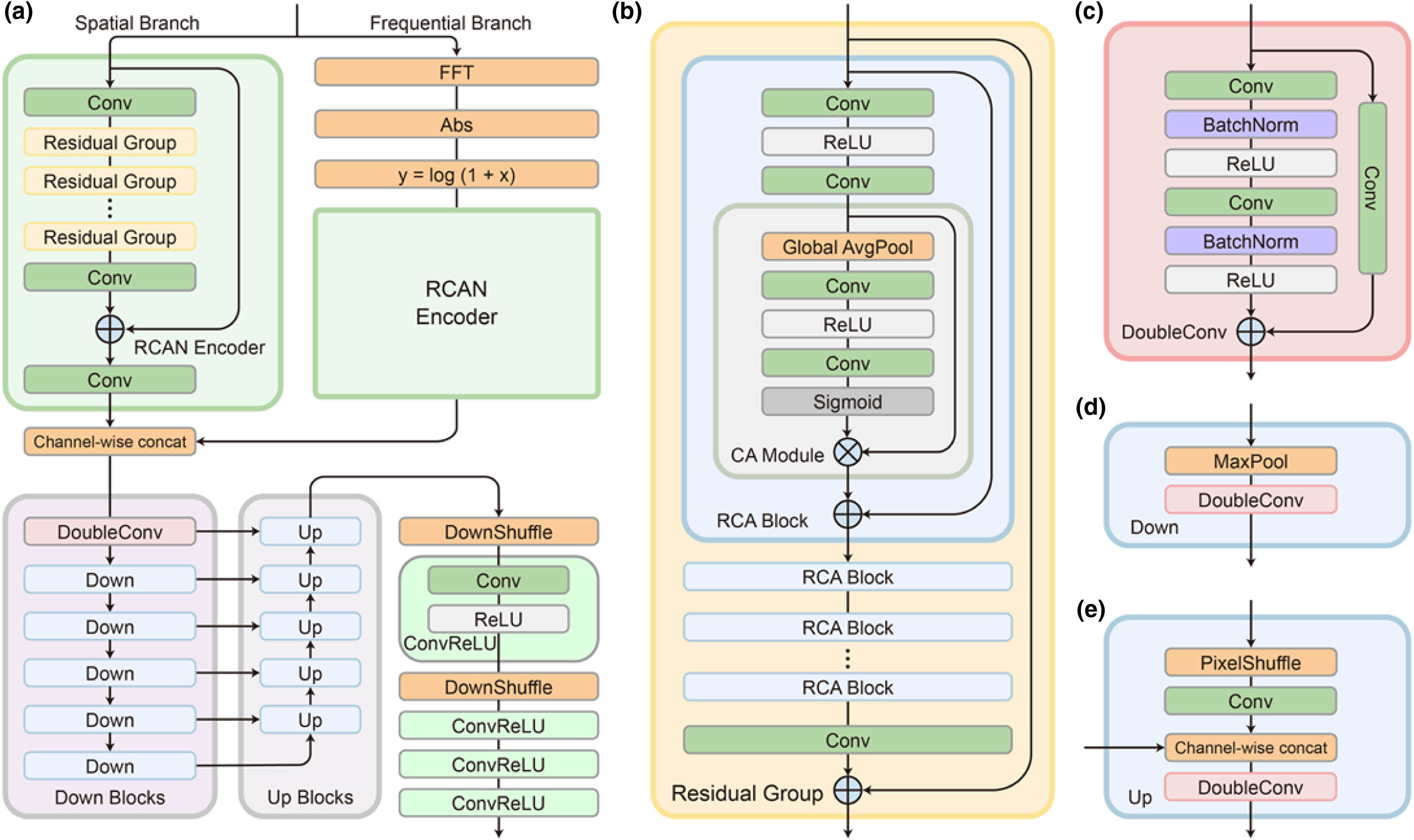
Set citation alerts for the article
Please enter your email address