Ruichao Zhu, Jiafu Wang, Tianshuo Qiu, Dingkang Yang, Bo Feng, Zuntian Chu, Tonghao Liu, Yajuan Han, Hongya Chen, Shaobo Qu. Direct field-to-pattern monolithic design of holographic metasurface via residual encoder-decoder convolutional neural network[J]. Opto-Electronic Advances, 2023, 6(8): 220148

Search by keywords or author
- Opto-Electronic Advances
- Vol. 6, Issue 8, 220148 (2023)
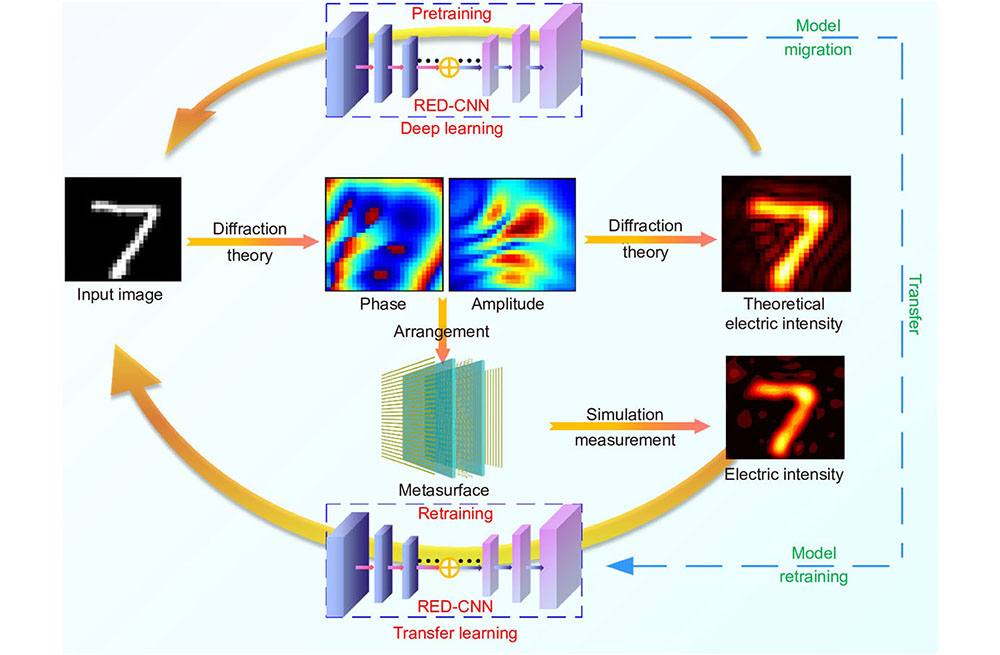
Fig. 1. Schematic diagram of CAHM monolithic design via REDCNN model
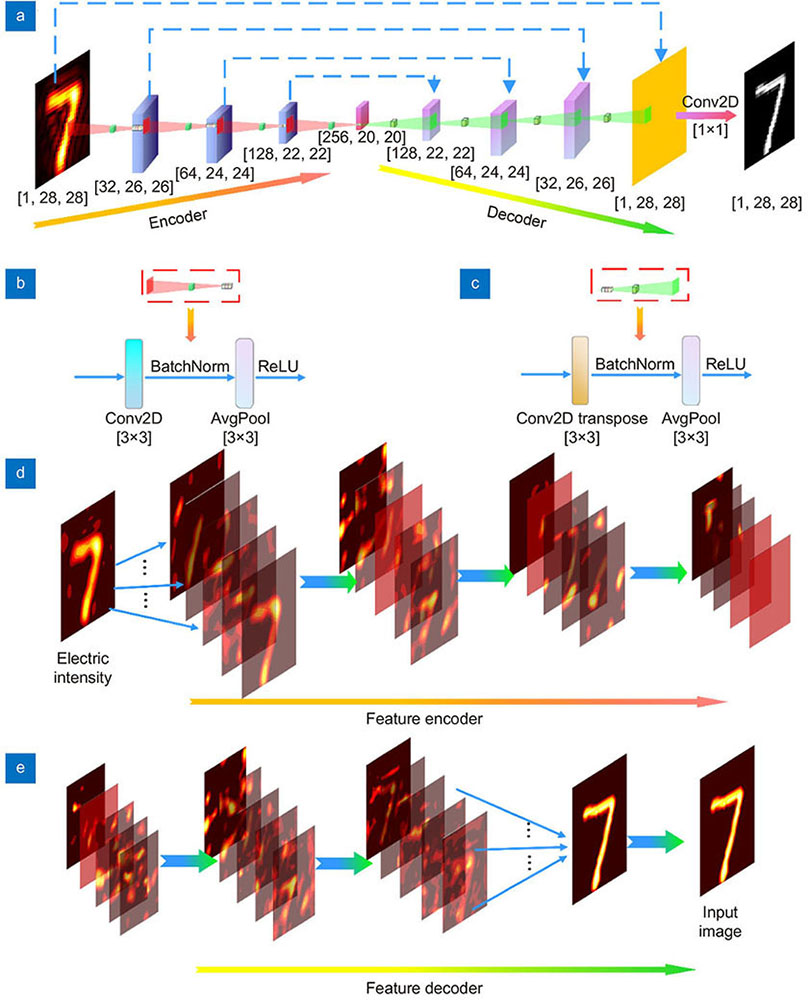
Fig. 2. REDCNN model design and feature extraction. (a ) The architecture and dimension of REDCNN model. (b ) The downsampling process of feature encoder. (c ) The upsampling process of feature decoder. (d ) Feature transfer of different channels in encoding process. (e ) Feature transfer of different channels in decoding process
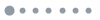
Fig. 3. Training and test of the REDCNN model. (a ) The variation of MAE loss value in deep learning process. (b ) The variation of MAE loss value in transfer learning process. (c ) The error histogram of deep learning in training set. (d ) The error histogram of deep learning in test set. (e ) The error histogram of transfer learning in training set. (f ) The error histogram of transfer learning in test set.
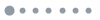
Fig. 4. The comparison of predicted metasurface and real metasurface with error distributions. (a ) Input images. (b ) Phase profiles of metasurface. (c ) Amplitude profiles of metasurface. (d ) Theoretical electric field distributions calculated by diffraction theory. (e ) Simulated electric field distributions.
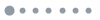
Fig. 5. Measurement verification and comparation of the metasurfaces. (a ) Photograph of fabricated metasurface prototype. (b , c ) Photograph of orthogonal metal gratings. (d ) Photograph of real metasurface pattern. (e ) Photograph of predicted metasurface pattern. (f ) Electric-field measurement environment in microwave anechoic chamber. (g ) Measured electric field distribution of real metasurface. (h ) Measured electric field distribution of predicted metasurface. (i ) The error of measured electric field distribution between the real and predicted metasurfaces
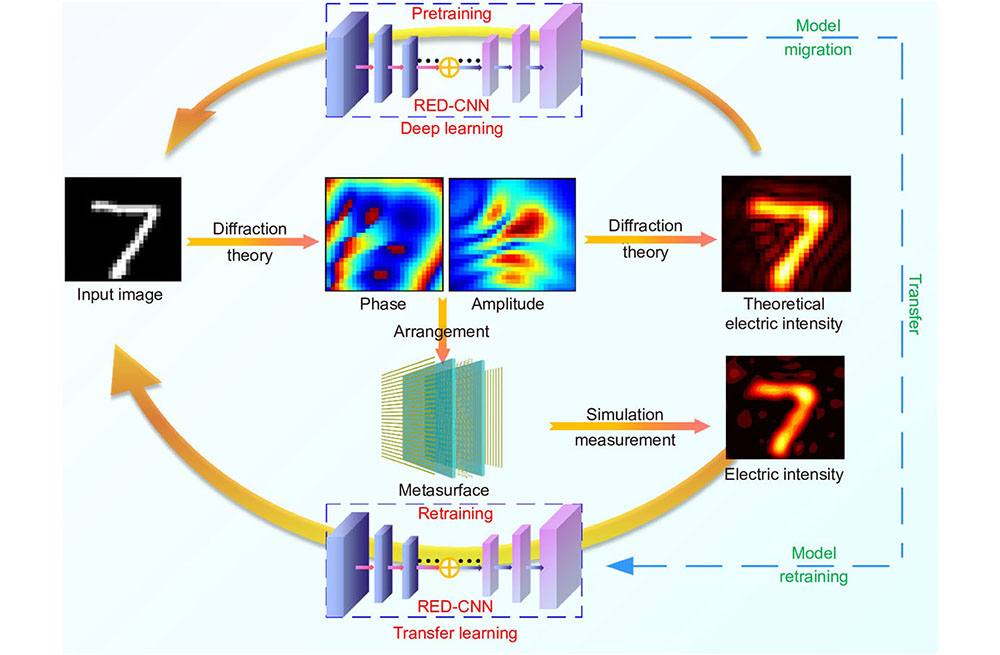
Set citation alerts for the article
Please enter your email address