
- Chinese Optics Letters
- Vol. 20, Issue 1, 011101 (2022)
Abstract
Keywords
1. Introduction
Ghost imaging (GI) is a non-local imaging technique that uses the second-order correlation function of light to obtain information about objects[
Creating on-chip GI algorithms is a key step in the practical application of GI because it reduces the cost and allows high-volume use of GI. GI requires a large number of measurements, which creates three challenges for on-chip GI algorithms to overcome: (i) there are many measurements, so data acquisition is time-consuming; (ii) required memory storage is very large, which is difficult to ensure in on-chip implementation; (iii) the image reconstruction algorithm takes a long time to form the image of the object. In 2020, we proposed an instant GI (IGI) algorithm that overcame challenges (ii) and (iii). The use of IGI greatly reduces the storage space required for GI, and the imaging time is almost zero. This study represents for the first time, to the best of our knowledge, in the field of GI that GI has been implemented on a chip, freeing GI from some of the limitations imposed by the use of conventional computers[
However, the IGI algorithm requires the same number of measurements as the standard GI algorithm to function at the same level. So, an algorithm is urgently needed that can both reduce the number of measurements and be suitable for on-chip implementation. In this study, we propose a new GI technique, derivative GI, to reduce the number of measurements and decrease the time taken for data acquisition. We make innovative use of the derivatives of the intensities of the test light and the reference light for imaging, and we have experimentally verified the effectiveness of this novel technique.
Sign up for Chinese Optics Letters TOC. Get the latest issue of Chinese Optics Letters delivered right to you!Sign up now
Our experimental results show that by combining derivative GI with the standard GI algorithm, multiple independent signals can be obtained in a single measurement. This approach greatly reduces the number of measurements required for imaging and improves image quality. Furthermore, derivative GI intrinsically does not have the storage-consuming background term of GI. Therefore, the derivative GI is suitable to apply to on-chip GI. Derivative GI overcomes a significant obstacle in the engineering application of GI and is therefore of major importance in the practical application of GI.
2. Methods
2.1. Theory
In essence, GI depends on the Hanbury Brown–Twiss (HBT) effect for any two spatial points. We propose and experimentally demonstrate derivative HBT. We show that combining derivative HBT with standard HBT can reduce the number of measurements required for correlation.
Derivative HBT is described by
In particular, when
It should be emphasized that all derivatives in this paper are derivates with respect to time
For GI, derivative GI is given by
In particular, when
2.2. Experimental setup
The experimental configuration is shown in Fig. 1. The 532 nm laser is projected onto the light-modulation glass disk to produce pseudo-thermal light. The pseudo-thermal light is split into two beams by the beam splitter. The test beam is directed onto the object, and light that passes through the object is received by the bucket detector; the reference beam is received directly by the array detector. The detector was a Hamamatsu S13620 array detector with
Figure 1.Experimental schematic. (a) The pseudo-thermal light is generated by a 532 nm laser illuminating a rotating light-modulating glass disk. The HBT experiment used a small hole in the object plane, and the GI experiment used the letter T as the object. (b) The bucket detector signal S and the reference light signal I(x) are received by the photodetectors to produce a photocurrent. The photocurrent is sampled by the analog-to-digital converter (A/D) to obtain S and I(x), and is transmitted to the field programmable gate array (FPGA). Simultaneously, the photocurrents enter the differentiators to produce the derivative signals, which then undergo A/D sampling and are transmitted to the FPGA for calculation of the experimental results. In the HBT experiment, the signal flow is similar to (b), with I(x1) and I(x2) replacing S and I(x). (c) The differentiators for S and I(x) are implemented by differentiating the operational amplitude circuits.
The distance from the glass to the object was equal to the distance from the glass to the detector array. The HBT effect was obtained using a small square hole in the object plane that allowed only light collected by one pixel to pass. The GI experiment used the letter T in the object plane.
We developed a configurable hardware system that sampled both the intensity and its first-order derivative [shown as the circuit board in Fig. 1(a)]. In the standard GI experiment, the signal was immediately sampled by the A/D and then transmitted to the field programmable gate array (FPGA). In the derivative GI experiment, the signal was initially sent to the differentiator to produce the derivative signal, which was then sampled by the A/D and transmitted to the FPGA for calculation of the correlations, as shown in Fig. 1(b). The HBT experiment used an identical configuration, but
All measured data of the derivative HBT and derivative GI were processed online immediately by the FPGA. When measurement ended, the correlation results were immediately available.
3. Experimental Results
Figure 2(a) shows the result of the first-order derivative HBT experiment, and Fig. 2(b) shows the result of the standard HBT experiment. The number of measurements was
Figure 2.Experimental results of (a) the first-order derivative HBT and (b) standard HBT. The number of measurements M in both cases was 512.
Figure 3(a) shows the object letter T; transmission through the white part was recorded as 1, and transmission through the black part was recorded as 0. The pixel size of the transparent part of the object was
Figure 3.(a) Object of the GI experiment, and the experimental results of (b) the first-order derivative GI and (c) standard GI. The number of measurements M in each case was 1024.
The signal-to-noise ratio (SNR) is
We want to emphasize that the derivative GI does not produce the background term (
In order to compare the derivative GI and standard GI easily, we subtracted the background term (
We now demonstrate that the first-order derivative HBT can be combined with standard HBT to reduce the number of experimental samples taken and show that data with different degrees of freedom can be obtained from one measurement. Figure 4(a) shows the first-order derivative HBT result (
Figure 4.Experimental results of (a) the first-order derivative HBT, (b) standard HBT (the number of measurements M in each case was 256), and (c) combination of (a) and (b) by simple addition after rescaling. The experimental results of (d) first-order derivative GI, (e) standard GI (the number of measurements M in each case was 512), and (f) the combination of (d) and (e).
Similarly, Figs. 4(d)–4(f) show that derivative GI [Fig. 4(d)] can be combined with standard GI [Fig. 4(f)] to reduce the number of image sample measurements and significantly improve image quality. A comparison of Figs. 4(f) and 3(c) shows that the combined image quality, from 512 measurements, is as good as the image quality of the standard GI algorithm from 1024 measurements.
The combination result is obtained by
Figure 5 shows the SNR results of the standard GI, the first-order derivative GI, and the combination of the two with different numbers of measurements. Each point with an error bar is calculated by 10 repeated experimental results. It can be seen from Fig. 5 that the image quality of standard GI and derivative GI is at the same level. For the same measurement number, the SNR of the combination is obviously better than that of any GI alone. Moreover, with increasing
Figure 5.Experimental results of the standard GI, the first-order derivative GI, and the combination of the two within different sample numbers M.
To validate the independence of the light intensity with its derivative signal, the experimental results of the correlation
Figure 6.Experimental results of 〈I (x0)I′(x)〉 − 〈I (x0)〉〈I′ (x)〉. x0 is chosen as (a) row 3 and column 3 and (b) row 5 and column 5, respectively.
4. Discussion
Because the signal strength
GI can be regarded as the achievement of
Some algorithms such as DGI, normalized GI, and higher-order GI can also improve the image quality of GI. There are two reasons why the derivative GI is more suitable for the on-chip implementation of GI. First, the derivative GI does not require one matrix to store the
In 1956, Hanbury Brown and Twiss used the second-order correlation of light intensity to measure the angular diameter of Sirius. The second-order correlation of intensity is known as the HBT effect[
5. Conclusions
In summary, we proposed the innovative derivative GI model and obtained image quality the same as standard GI. The importance of derivative GI is seen when the correlation results are combined with those of standard GI; signals with multiple degrees of freedom can be obtained in one measurement. Combining the intensity with its derivative reduces the number of measurements required and thus data-acquisition time. Moreover, derivative GI can be applied to on-chip GI because it intrinsically does not produce the storage-consuming background term of GI and is suitable for on-chip implementation.
We used experiments to show the effectiveness of first-order derivative GI and first-order derivative HBT. The method of using first-order derivative GI and higher-order derivative GI that we demonstrated will promote greater practical application of GI in many areas.
References
[1] T. B. Pittman, Y. H. Shih, D. V. Strekalov, A. V. Sergienko. Optical imaging by means of two-photon quantum entanglement. Phys. Rev. A, 52, R3429(1995).
[2] R. S. Bennink, S. J. Bentley, R. W. Boyd. ‘Two-photon’ coincidence imaging with a classical source. Phys. Rev. Lett., 89, 113601(2002).
[3] A. Gatti, E. Brambilla, M. Bache, L. A. Lugiato. Ghost imaging with thermal light: comparing entanglement and classical correlation. Phys. Rev. Lett., 93, 093602(2004).
[4] F. Ferri, D. Magatti, A. Gatti, M. Bache, E. A. Brambilla, L. A. Lugiato. High-resolution ghost image and ghost diffraction experiments with thermal light. Phys. Rev. Lett., 94, 183602(2005).
[5] D. Z. Cao, J. Xiong, K. Wang. Geometrical optics in correlated imaging systems. Phys. Rev. A, 71, 013801(2005).
[6] J. H. Shapiro. Computational ghost imaging. Phys. Rev. A, 78, 061802(2008).
[7] O. Katz, B. Yaron, Y. Silberberg. Compressive ghost imaging. Appl. Phys. Lett., 95, 131110(2009).
[8] G. M. Gibson, S. D. Johnson, M. Padgett. Single-pixel imaging 12 years on: a review. Opt. Express, 28, 28190(2020).
[9] Z. Yang, O. S. Magana-Loaiza, M. Mirhosseini, Y. Zhou, B. Gao, L. Gao, S. M. H. Rafsanjani, G. L. Long, R. W. Boyd. Digital spiral object identification using random light. Light: Sci. Appl., 6, e17013(2017).
[10] L. Wang, S. Zhao. Super resolution ghost imaging based on Fourier spectrum acquisition. Opt. Laser. Eng., 139, 106473(2021).
[11] X. Zhang, Y. H. He, L. A. Wu, L. M. Chen, B. B. Wang. Tabletop x-ray ghost imaging with ultra-low radiation. Optica, 5, 374(2018).
[12] N. Radwell, K. J. Mitchell, G. M. Gibson, M. P. Edgar, R. Bowman, M. J. Padgett. Single-pixel infrared and visible microscope. Optica, 1, 285(2014).
[13] J. Zhao, E. Yiwen, K. Williams, X. C. Zhang, R. Boyd. Spatial sampling of terahertz fields with sub-wavelength accuracy via probe beam encoding. Light: Sci. Appl., 8, 55(2019).
[14] G. Wang, H. Zheng, Z. Tang, Y. He, Y. Zhou, H. Chen, J. Liu, Y. Yuan, F. Li, Z. Xu. Naked-eye ghost imaging via photoelectric feedback. Chin. Opt. Lett., 18, 091101(2020).
[15] J. Gu, S. Sun, Y. Xu, H. Lin, W. Liu. Feedback ghost imaging by gradually distinguishing and concentrating onto the edge area. Chin. Opt. Lett., 19, 041102(2021).
[16] L. Basano, P. Ottonello. Experiment in lensless ghost imaging with thermal light. Appl. Phys. Lett., 89, 091109(2006).
[17] Z. Yang, L. Zhao, X. Zhao, W. Qin, J. Li. Lensless ghost imaging through the strongly scattering medium. Chin. Phys. B, 25, 024202(2016).
[18] M. J. Sun, M. P. Edgar, G. M. Gibson, B. Sun, N. Radwell, R. Lamb, M. J. Padgett. Single-pixel three-dimensional imaging with time-based depth resolution. Nat. Commun., 7, 12010(2016).
[19] W. Gong, C. Zhao, H. Yu, M. Chen, W. Xu, S. Han. Three-dimensional ghost imaging lidar via sparsity constraint. Sci. Rep., 6, 26133(2016).
[20] Y. Wang, H. Chen, W. Jiang, X. Li, X. Chen, X. Meng, P. Tian, B. Sun. Optical encryption for visible light communication based on temporal ghost imaging with a micro-LED. Opt. Laser. Eng., 134, 106290(2020).
[21] F. Ferri, D. Magatti, L. A. Lugiato, A. Gatti. Differential ghost imaging. Phys. Rev. Lett., 104, 253603(2010).
[22] X. R. Yao, W. K. Yu, X. F. Liu, L. Z. Li, M. F. Li, L. A. Wu, G. J. Zhai. Iterative denoising of ghost imaging. Opt. Express, 22, 24268(2014).
[23] S. S. Hodgman, W. Bu, S. B. Mann, R. I. Khakimov, A. G. Truscott. Higher-order quantum ghost imaging with ultracold atoms. Phys. Rev. Lett., 122, 233601(2019).
[24] C. M. Watts, D. Shrekenhamer, J. Montoya, G. Lipworth, J. Hunt, T. Sleasman, S. Krishna, D. R. Smith, W. J. Padilla. Terahertz compressive imaging with metamaterial spatial light modulators. Nat. Photon., 8, 605(2014).
[25] Z. Zhang, X. Ma, J. Zhong. Single-pixel imaging by means of Fourier spectrum acquisition. Nat. Commun., 6, 6225(2015).
[26] K. M. Czajkowski, A. Pastuszczak, R. Kotynski. Real-time single-pixel video imaging with Fourier domain regularization. Opt. Express, 26, 20009(2018).
[27] L. Wang, S. Zhao. Fast reconstructed and high-quality ghost imaging with fast Walsh–Hadamard transform. Photon. Res., 4, 240(2016).
[28] Z. H. Xu, W. Chen, J. Penuelas, M. J. Padgett, M. J. Sun. 1000 fps computational ghost imaging using LED-based structured illumination. Opt. Express, 26, 2427(2018).
[29] J. Liu, J. Zhu, C. Lu, S. Huang. High-quality quantum-imaging algorithm and experiment based on compressive sensing. Opt. Lett., 35, 1206(2010).
[30] J. Liu. On the recovery conditions for practical ghost imaging with AMP algorithm. Opt. Express, 26, 20519(2018).
[31] H. Wu, R. Wang, G. Zhao, H. Xiao, X. Zhang. Sub-Nyquist computational ghost imaging with deep learning. Opt. Express, 28, 3846(2020).
[32] F. Wang, H. Wang, H. Wang, G. Li, G. Situ. Learning from simulation: an end-to-end deep-learning approach for computational ghost imaging. Opt. Express, 27, 25560(2019).
[33] H. Wu, G. Zhao, M. Chen, L. Cheng, H. Xiao, L. Xu, D. Wang, J. Liang, Y. Xu. Hybrid neural network-based adaptive computational ghost imaging. Opt. Laser. Eng., 140, 106529(2021).
[34] H. Wu, R. Wang, G. Zhao, H. Xiao, J. Liang, D. Wang, X. Tian, L. Cheng, X. Zhang. Deep-learning denoising computational ghost imaging. Opt. Laser. Eng., 134, 106183(2020).
[35] T. Bian, Y. Yi, J. Hu, Y. Zhang, Y. Wang, L. Gao. A residual-based deep learning approach for ghost imaging. Sci. Rep., 10, 1(2020).
[36] Z. Yang, W. X. Zhang, Y. P. Liu, D. Ruan, J. L. Li. Instant ghost imaging: algorithm and on-chip implementation. Opt. Express, 28, 3607(2020).
[37] Z. Yang, W. X. Zhang, M. C. Zhang, D. Ruan, J. L. Li. Instant ghost imaging: improving robustness for ghost imaging on optical background noise. OSA Continuum, 3, 391(2020).
[38] M. J. Sun, H. Y. Wang, J. Y. Huang. Improving the performance of computational ghost imaging by using a quadrant detector and digital micro-scanning. Sci. Rep., 9, 4105(2019).
[39] R. H. Brown, R. Q. Twiss. Correlation between photons in two coherent beams of light. Nature, 177, 27(1956).
[40] R. H. Brown, R. Q. Twiss. A test of a new type of stellar interferometer on sirius. Nature, 178, 1046(1956).
[41] R. H. Miller. Measurement of stellar diameters. Science, 153, 581(1966).
[42] G. Baym. The physics of Hanbury Brown–Twiss intensity interferometry: from stars to nuclear collisions. Acta Phys. Pol. B, 29, 1839(1998).
[43] E. Frodermann, U. Heinz. Photon Hanbury Brown–Twiss interferometry for noncentral heavy-ion collisions. Phys. Rev. C, 80, 044903(2009).
[44] C. Plumberg, U. Heinz. Probing the properties of event-by-event distributions in Hanbury Brown–Twiss radii. Phys. Rev. C, 92, 044906(2015).
[45] M. Schellekens, R. Hoppeler, A. Perrin, J. V. Gomes, D. Boiron, A. Aspect, C. I. Westbrook. Hanbury Brown Twiss effect for ultracold quantum gases. Science, 310, 648(2005).
[46] H. Cayla, S. Butera, C. Carcy, A. Tenart, G. Hercé, M. Mancini, A. Aspect, I. Carusotto, D. Clément. Hanbury Brown and Twiss bunching of phonons and of the quantum depletion in an interacting Bose gas. Phys. Rev. Lett., 125, 165301(2020).
[47] Y. Bromberg, Y. Lahini, E. Small, Y. Silberberg. Hanbury Brown and Twiss interferometry with interacting photons. Nat. Photon., 4, 721(2010).
[48] J. Leach, B. Jack, J. Romero, A. K. Jha, A. M. Yao, S. Franke-Arnold, D. G. Ireland, R. W. Boyd, S. M. Barnett, M. J. Padgett. Quantum correlations in optical angle–orbital angular momentum variables. Science, 329, 662(2010).
[49] S. Hong, R. Riedinger, I. Marinković, A. Wallucks, S. G. Hofer, R. A. Norte, M. Aspelmeyer, S. Gröblacher. Hanbury Brown and Twiss interferometry of single phonons from an optomechanical resonator. Science, 358, 203(2017).
[50] T. Thomay, S. V. Polyakov, O. Gazzano, E. Goldschmidt, Z. D. Eldredge, T. Huber, V. Loo, G. S. Solomon. Simultaneous, full characterization of a single-photon state. Phys. Rev. X, 7, 041036(2017).
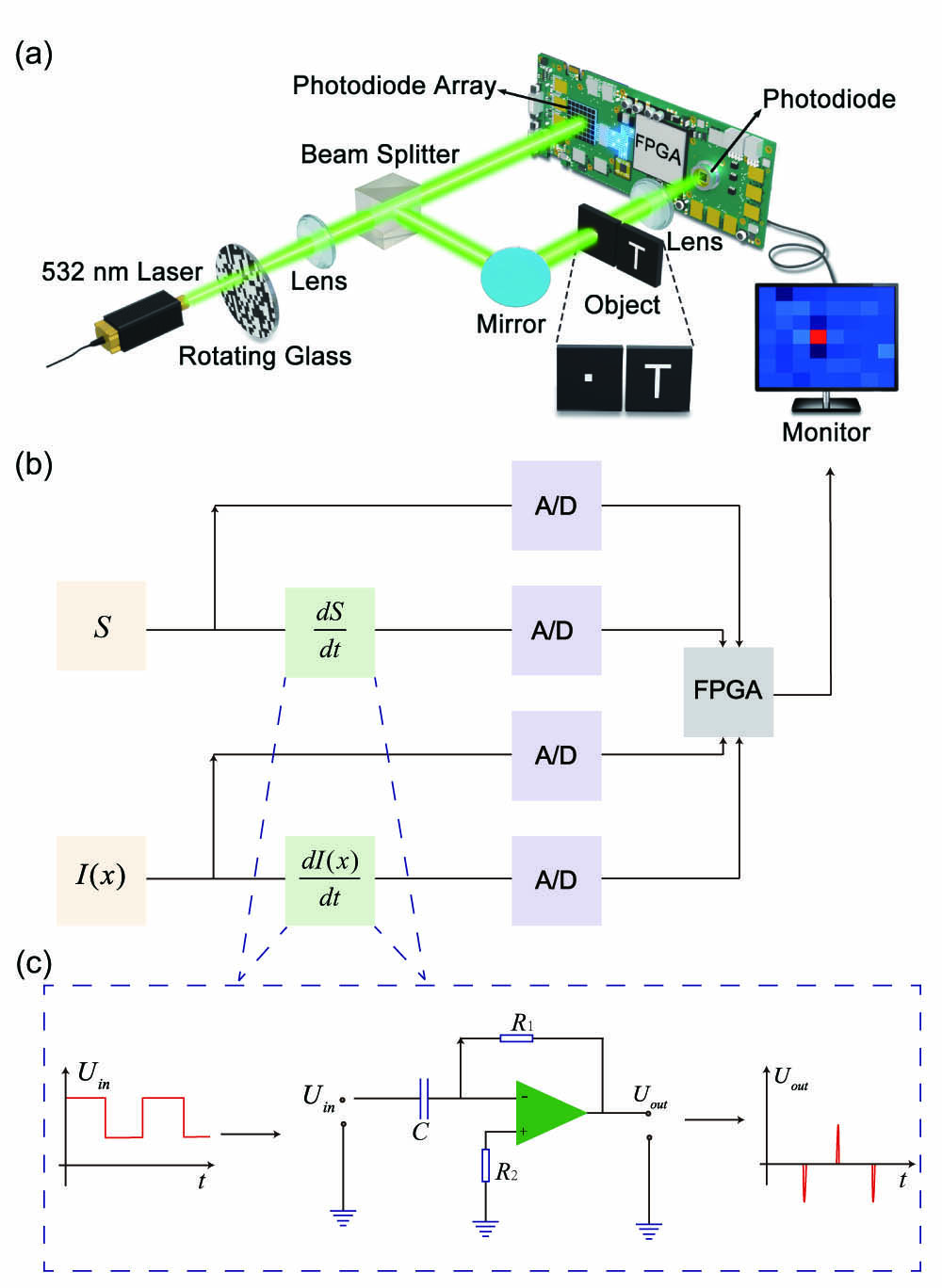
Set citation alerts for the article
Please enter your email address