Tao Li, Wei Jin, Randi Fu, Gang Li, Caoqian Yin. Nighttime sea fog recognition based on remote sensing satellite and deep neural decision tree[J]. Opto-Electronic Engineering, 2022, 49(9): 220007

Search by keywords or author
- Opto-Electronic Engineering
- Vol. 49, Issue 9, 220007 (2022)
Abstract
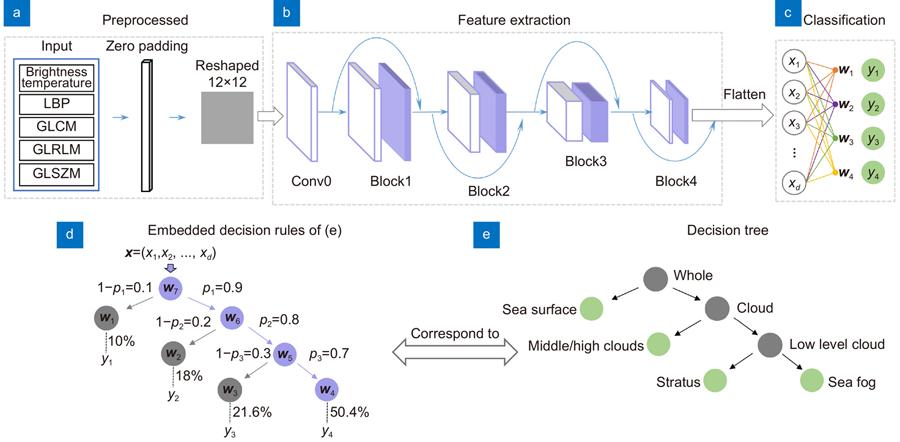
Set citation alerts for the article
Please enter your email address