Yinan Ji, Haifeng Li, Xu Liu. Image segmentation learning method for large field single lens computational imaging system[J]. Opto-Electronic Engineering, 2022, 49(5): 210371

Search by keywords or author
- Opto-Electronic Engineering
- Vol. 49, Issue 5, 210371 (2022)
Abstract
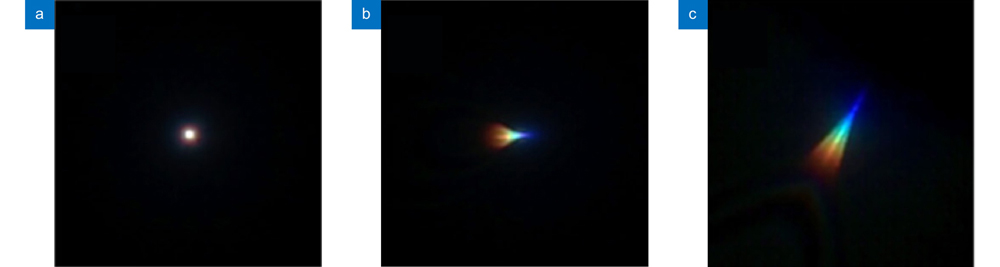
Set citation alerts for the article
Please enter your email address