Cheng Luo, Man-Kit Sit, Hongxiang Fan, Shuanglong Liu, Wayne Luk, Ce Guo. Towards efficient deep neural network training by FPGA-based batch-level parallelism[J]. Journal of Semiconductors, 2020, 41(2): 022403

Search by keywords or author
- Journal of Semiconductors
- Vol. 41, Issue 2, 022403 (2020)
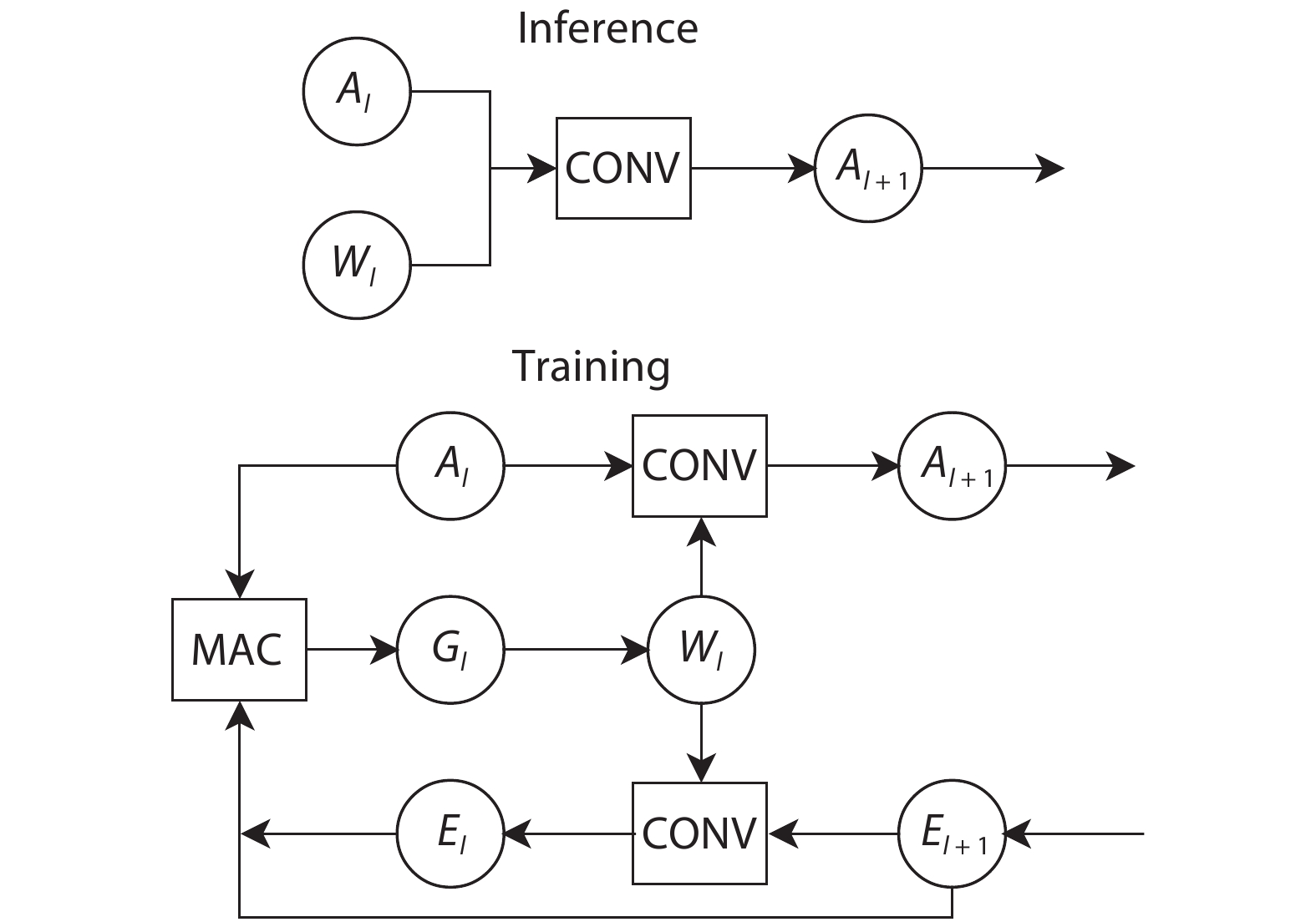
Fig. 1. A overview of inference and training processes on the convolutional layer.
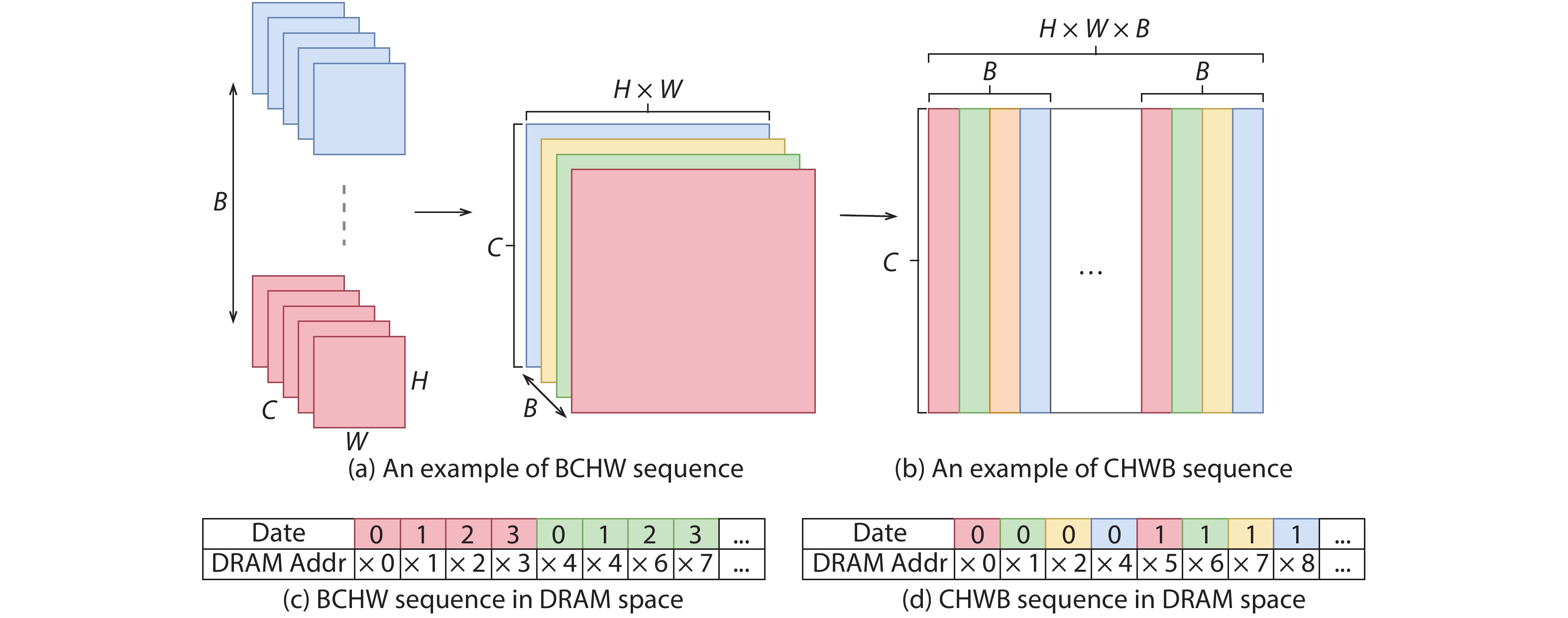
Fig. 2. (Color online) Comparison of BCHW and CHWB patterns.
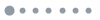
Fig. 3. (Color online) The tiling flow for convolution.
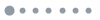
Fig. 4. (Color online) System overview.
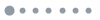
Fig. 5. (Color online) Hardware architecture of GEMM kernel.
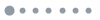
Fig. 6. (Color online) Input double buffer supporting matrix transposition.
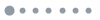
Fig. 7. (Color online) The DarkFPGA framework.
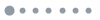
Fig. 8. (Color online) Performance and resource consumption experiments under different design space using int8 weights. (a) Computational time. (b) Performance evaluation. (c) Resource consumption.
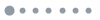
Fig. 9. (Color online) Performance comparisons between homogeneous system and heterogeneous system.
|
Table 1. Parameters for FPGA training.
|
Table 2. The network architecture in experiment.
|
Table 3. Performance comparison among FPGA, CPU and GPU.
|
Table 4. Performance comparison of different FPGA-based training accelerators.
|
Table 5. [in Chinese]
|
Table 6. [in Chinese]
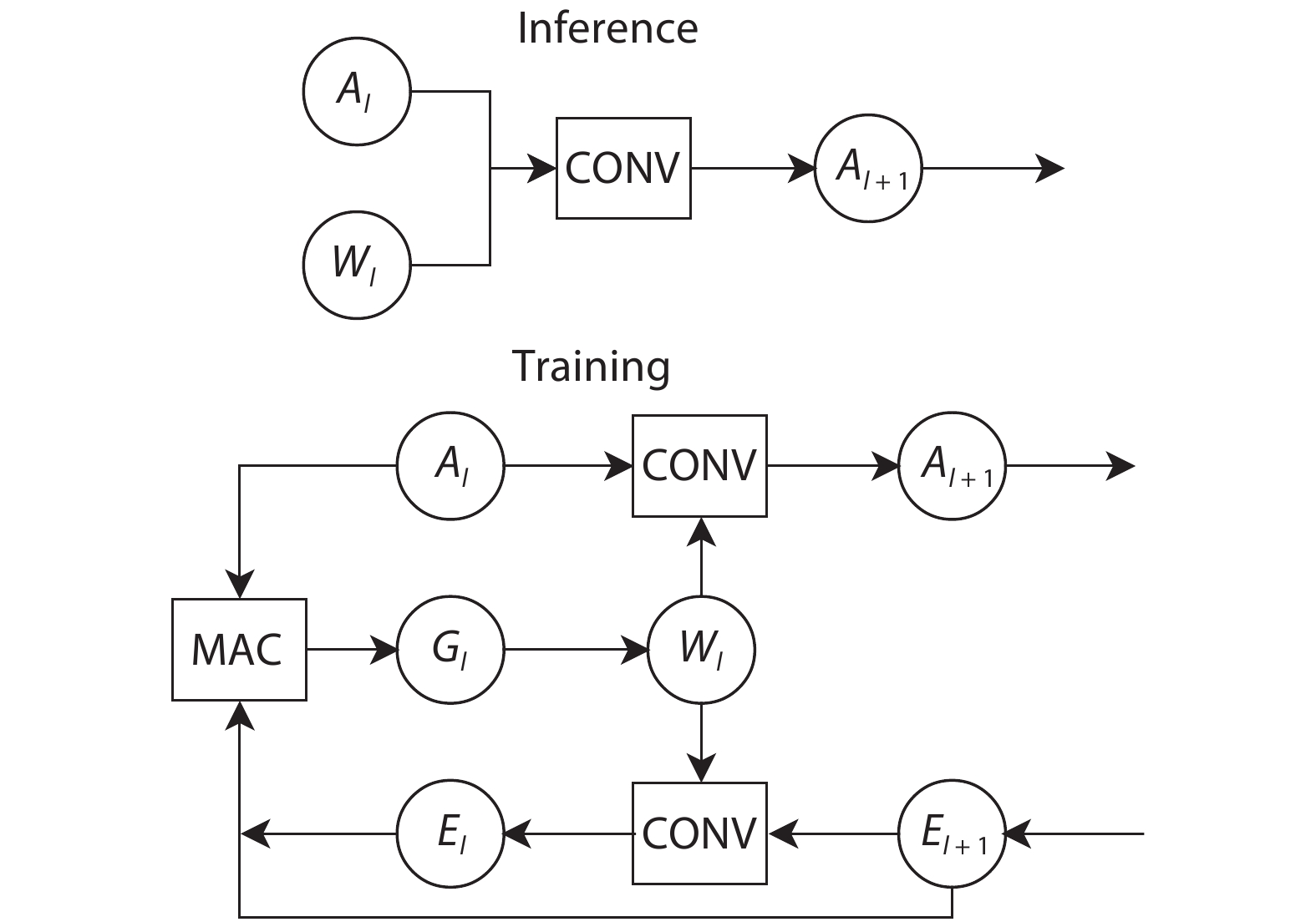
Set citation alerts for the article
Please enter your email address