Jie-hong CHENG, Zheng-guang CHEN, Shu-juan YI. Wavelength Selection Algorithm Based on Minimum Correlation Coefficient for Multivariate Calibration[J]. Spectroscopy and Spectral Analysis, 2022, 42(3): 719

Search by keywords or author
- Spectroscopy and Spectral Analysis
- Vol. 42, Issue 3, 719 (2022)
Abstract
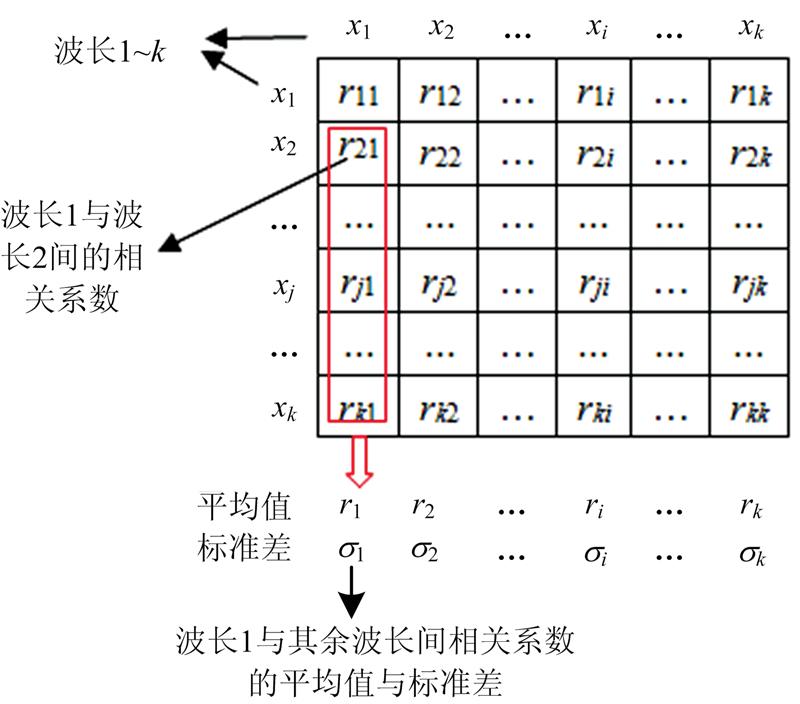
Set citation alerts for the article
Please enter your email address