ZHANG Hong-rui, LIU Chang-hong, ZHANG Jiu-kai, HAN Jian-xun, CHEN Ying, ZHENG Lei. Qualitative Identification and Quantitative Analysis of Maca Adulteration Based on Multispectral Imaging Technology[J]. Spectroscopy and Spectral Analysis, 2020, 40(1): 152

Search by keywords or author
- Spectroscopy and Spectral Analysis
- Vol. 40, Issue 1, 152 (2020)
Abstract
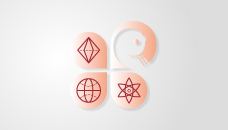
Set citation alerts for the article
Please enter your email address