Imaging through scattering media is one of the hotspots in the optical field, and impressive results have been demonstrated via deep learning (DL). However, most of the DL approaches are solely data-driven methods and lack the related physics prior, which results in a limited generalization capability. In this paper, through the effective combination of the speckle-correlation theory and the DL method, we demonstrate a physics-informed learning method in scalable imaging through an unknown thin scattering media, which can achieve high reconstruction fidelity for the sparse objects by training with only one diffuser. The method can solve the inverse problem with more general applicability, which promotes that the objects with different complexity and sparsity can be reconstructed accurately through unknown scattering media, even if the diffusers have different statistical properties. This approach can also extend the field of view (FOV) of traditional speckle-correlation methods. This method gives impetus to the development of scattering imaging in practical scenes and provides an enlightening reference for using DL methods to solve optical problems.

- Photonics Research
- Vol. 9, Issue 5, B210 (2021)
Abstract
1. INTRODUCTION
Object information is seriously degraded after being modulated by complex media [1,2]. The scattering of light in the diffusion is an established problem considered to be a common phenomenon in our daily life (i.e., seeing through dense fog to obtain the license plate and driver’s facial information is crucial for a traffic monitor). Imaging with randomly scattered light is a challenging problem with an urgent requirement in different fields (e.g., astronomical observations through the turbulent atmosphere and biological analysis through active tissue) [3–7]. Conventional imaging methods based on geometric optics cannot work with the disordered light beam under scattering. Benefitting from the great progress of optoelectronic devices and computational techniques, many new imaging methods have been proposed for imaging through scattering media. The typical imaging techniques include the wavefront-shaping methods [8–11], reconstruction using the transmission matrix [12,13], single-pixel imaging methods [14–16], or techniques based on the point spread function (PSF) [17–19]. The methods listed here have made great progress in object reconstruction with invasive prior, and the scattering scenes are relatively stable. Speckle correlation based on the optical memory effect (OME) is an extraordinary method for noninvasive imaging through opaque layers [20] with only one frame of speckle pattern [21,22]. Object recovery based on speckle correlation methods uses phase retrieval algorithms such as hybrid input-output (HIO) [23], those based on the alternating direction method of multipliers (ADMM) [24], and phase retrieval based on generalized approximate message passing (prGAMP) [25]. The field of view (FOV) of speckle-correlation methods is limited by OME, and the recovery performance is also influenced by the recovery capability of phase retrieval algorithms.
Recently, with the advent of digital technology, big data, and advanced optoelectronic technology, deep learning (DL) has shown great potential in optics and photonics [26,27]. With powerful data mining and mapping capabilities, the data-informed DL methods can extract the key features and build a reliable model in many fields [28]. To date, the DL approach has been successfully applied in digital holography imaging [29–32], Fourier ptychographic imaging [33–36], computational ghost imaging [37,38], superresolution microscopic imaging [39–42], optical tomography imaging [43–45], photon-limited imaging [46,47], three-dimensional (3D) measurements with fringe pattern analysis [48–51], and imaging through scattering media [52–60]. Compared to traditional computational imaging (CI) technology, the learning-heuristic methods can not only solve complex imaging problems, but also can significantly improve the core performance indicators (i.e., spatial resolution, temporal resolution, and sensitivity). The great progress by DL is indicated by the rapidly increasing number of DL-related publications in photonics journal in the last several years [61]. However, the methods using DL are meeting several challenging problems, such as the choice of the DL framework tending to be empirical and the limited generalization capability.
Based on the nonlinear characteristics of deep neural networks (DNNs), DL methods have good performance in highly ill-posed problems, especially in imaging through random media [52–54,57]. IDiffNet is the first proposed method to reconstruct an object through scattering media via a densely connected DNN. The performance with a different type of training dataset and the loss function are systematically discussed [52]. A hybrid neural network is constructed to see through a thick scattering medium and achieves object restoration exceeding the FOV of OME [53]. The speckle patterns of single-mode fiber and multimode fibers are reconstructed and recognized successfully [54]. PDSNet is built to reconstruct complex objects through scattering medium and expands the FOV up to 40 times of the memory effect (ME) [55]. The methods above are mainly focused on a specified diffuser that has a limitation in complex and variable scattering conditions. Therefore, some DL methods have the potential to reconstruct objects through unstable media that mainly use different DNN structures, such as one-to-all with dense blocks, interpretable DL method, generative adversarial network (GAN), or a two-stage framework [57–60]. Li
Sign up for Photonics Research TOC. Get the latest issue of Photonics Research delivered right to you!Sign up now
In this paper, with the physics prior of scattering and the support of DL, a physics-informed learning method is proposed for imaging through unknown diffusers. By pre-processing, the data model based on the physics prior can solve the generalization problems in different scattering scenes, which can reduce the data dependence of the DL model and robustly improve the feature extraction efficiency. The efficient physics prior can provide an optimized direction for DNN to find the optimal reconstruction solution in different scattering scenes. The DL method based on physics prior can help to learn and extract the statistical invariants from different scattering scenes. Instead of training with captured patterns directly, using the DL framework with speckle correlation prior to imaging through different diffusers is technologically reasonable. Employing the physics-informed learning method, scalable imaging through unknown diffusers can be achieved with high reconstruction quality. The scattering degradation of the sparse objects can even be modeled with one ground glass, and imaging through unknown ground glasses even with different statistical characteristics can be achieved. More complex objects (e.g., human faces) can be reconstructed accurately by slightly increasing the number of training diffusers. Meanwhile, it is hard to restore the objects efficiently exceeding the FOV of OME by the traditional speckle-correlation method. Based on the powerful capability in data mining and processing of DNN, the physics-informed learning method can also break through the FOV limitation for scalable imaging. Finally, we demonstrate the physics-informed learning scheme with an experimental dataset and present the quantitative evaluation results with multiple indicators. The results with the statistical average indicator show the accuracy and robustness of our scheme, and reflect the great potential of combining physical knowledge and DL.
2. METHODS
A. Physical Basic
The proposed model must be established to have general applicability for scalable imaging through unknown diffusers, and it is also one of the indispensable conditions to apply this method to practical complex scenes. The wave propagating through an inhomogeneous medium with multiple scattering will generate a fluctuating intensity pattern, and the universal physical law exists in different transmitted modes. The speckle correlation and memory effect in optical wave transmission through disordered media are proposed to observe and analyze the shift-invariant characteristic of speckle patterns [62,63]. The speckle patterns of scattered light through diffusive media are invariant to small tilts or shifts in the incident wavefront of light, and the outgoing light field still retains the information carried by the incoming beam within the range of ME [64]. Therefore, within the scope of ME, the scattering system can be considered as an imaging system with shift-invariant point spread function. The speckle pattern captured by the camera is given by the convolution of the object intensity pattern
Figure 1.Speckle statistical characteristics analysis of the same object corresponding to different testing diffusers. (a) First row and second row are the speckle autocorrelation of the object within or exceeding the OME range, the third row is the cross-correlation with D1, respectively. (b)–(d) Intensity values of the white dash lines in the first, second, and third rows of (a), respectively. The color bar represents the normalized intensity. Scale bars: 875.52 µm.
With the speckle-correlation prior, the statistical invariants of the object through different scattering media can be effectively extracted, which informs the DNN to obtain useful information and reconstruct the object in different scattering scenes. Imaging through different scattering media with speckle-correlation prior can be used as a reference and a heuristic approach to design the DL methods in different optical problems.
B. Framework of Physics-Informed Learning
Figure 2.Schematic of the physics-informed learning method for scalable scattering imaging.
After the speckle-correlation pre-processing step, the captured speckle pattern is adjusted and refactored, and the next step is post-processing by DNN to reconstruct the hidden object. By adding the speckle-correlation theory, the imaging model can make full use of the advantages of the neural network. The DL model is a simple convolutional neural network (CNN) of the U-Net type [65]. Comparing a specially designed DNN structure, the physics-informed learning method can achieve better imaging results with a simple U-Net without any other tricks.
In our experiments, multiple objects datasets with different levels of complexity are used to reconstruct through different diffusers, such as the modified National Institute of Standards and Technology (MINIST) dataset [66] and FEI face dataset [67]. An equilibrium constraint loss function is important for the training process, and we design a combination loss that includes negative Pearson correlation coefficient (NPCC) loss and mean square error (MSE). The Pearson correlation coefficient is an index used to evaluate the similarity between two variables, and the calculated value is distributed from
3. EXPERIMENTS AND RESULTS
A. Experimental Arrangement and Data Acquisition
Figure 3.Experimental setup for the scalable imaging. Different diffusers are employed to obtain speckle patterns with different scattering scenes. The OME range of this system is also measured by calculating the cross-correlation coefficient [21]. See Appendix
To obtain the speckle patterns in different scattering scenes, nine different ground glasses are used as the diffusers in the experiments, including six 220 grit diffusers (D1–D6), one 120 grit diffuser (D7), one 600 grit diffuser (D8) produced by Thorlabs, and one 220 grit diffuser (D9) produced by Edmund, like the configuration in Section 2.B. We choose one ground glass (D1) or the first three pieces of ground glasses (D1, D2, and D3) as the training diffusers, and the remaining ground glasses as the test diffusers. The objects are mainly selected from the MINIST database and FEI face databases. The character objects are selected randomly from the MINIST dataset to form the different complexity of single-character and double-character objects. For collecting the experimental data, 600 single characters, 600 double characters, and 400 human faces are used as objects hidden behind each diffuser. The first 500 characters are used as the seen objects and the remaining characters are used as the unseen objects. Similarly, the first 360 human faces are used as seen objects and the remaining faces are used as unseen objects. The autocorrelation pre-processing for speckle patterns is the first step for our method. As for the processing of the speckle patterns, we take the
According to different training data and testing data, different groups are used to characterize the generalization capability of the physics-informed DL method, respectively. All of the testing data are captured from unknown diffusers for emphasizing the generalization. The data can be roughly divided into four groups.
B. Scalable Imaging with Different Diffusers
Figure 4.Testing results for generalization reconstruction of Group 1. Scale bars: 264.24 µm.
Figure 5.Testing results for generalization reconstruction of Group 2. Scale bars: 264.24 µm.
Figure 6.Testing results for generalization reconstruction of Group 3. Scale bars: 264.24 µm.
Figure 7.Testing results for generalization reconstruction of Group 4. Scale bars: 820.8 µm.
Figure 8.Generalization results for a single-character object with different scales and the scale of FOV is defined as the FOV/OME times. (a), (b) Results with different amounts of training diffusers, which are trained with one diffuser and three diffusers, respectively. (c) Reconstruction results with different scales and corresponding ground truth (GT).
4. ANALYSIS
A. Comparison to Traditional DL Strategy
Figure 9.Comparison results without or with this pre-processing step for imaging through an unknown diffuser. Three ground glasses are selected as the training diffusers and another diffuser for testing.
B. Performance with Different Number of Speckles
Figure 10.Results with different number of speckles via the physics-informed learning method. Three ground glasses are selected as the training diffusers and another diffuser for testing.
C. Performance in Exceeding FOV
Figure 11.Generalization results of imaging exceeding OME range with different complexity objects.
5. DISCUSSION
According to the experimental results shown in Section 3, we have three key points.
6. CONCLUSION
In this paper, a physics-informed learning method is introduced to imaging through diffusers. Specifically, an explicit framework is established to efficiently solve the generalization problems in different scattering scenes by combining the physics theories and DL methods. This is a new approach to solve scalable imaging with deep learning, which can reconstruct complex objects through different scattering media, and provide an expanded FOV for the real imaging scenes. In the future, more complex scenes and objects can be considered, which can be applied to volumetric multiple scattering, such as biological imaging and astronomical imaging.
Acknowledgment
Acknowledgment. The authors thank Qianying Cui, Yingjie Shi, Chenyin Zhou, Kaixuan Bai, and Mengzhang Liu for technical support and experimental discussions.
APPENDIX A: THE FORMULA DERIVATION TO EXCEED THE OME RANGE
When the object size exceeds the range of OME, the object can be divided into multiple objects
APPENDIX B: OME RANGE CALIBRATION DETAILS
To calibrate the range of the shift-invariant, the distance from the object to the diffuser is changed to 15?cm and the image distance is maintained as 8?cm. A ground glass (DG100X100-220-N-BK7, Thorlabs) is used as the diffuser and placed between the object and the CMOS. A series of speckle patterns are collected while the horizontal displacement of the point object on the object surface is achieved. The cross-correlation coefficient between the speckle patterns and the PSF of the system is calculated. A threshold value of 0.5 is chosen as the cross-correlation coefficient to determine the range of OME [
References
[1] J. W. Goodman. Speckle Phenomena in Optics: Theory and Applications(2007).
[2] M. C. Roggemann, B. M. Welsh, B. R. Hunt. Imaging Through Turbulence(1996).
[3] R. K. Tyson. Principles of Adaptive Optics(2015).
[4] E. J. McCartney. Optics of the Atmosphere: Scattering by Molecules and Particles(1976).
[5] V. Ntziachristos. Going deeper than microscopy: the optical imaging frontier in biology. Nat. Methods, 7, 603-614(2010).
[6] S. Yoon, M. Kim, M. Jang, Y. Choi, W. Choi, S. Kang, W. Choi. Deep optical imaging within complex scattering media. Nat. Rev. Phys., 2, 141-158(2020).
[7] L. V. Wang, H.-I. Wu. Biomedical Optics: Principles and Imaging(2012).
[8] A. P. Mosk, A. Lagendijk, G. Lerosey, M. Fink. Controlling waves in space and time for imaging and focusing in complex media. Nat. Photonics, 6, 283-292(2012).
[9] I. M. Vellekoop, A. Mosk. Focusing coherent light through opaque strongly scattering media. Opt. Lett., 32, 2309-2311(2007).
[10] S. Rotter, S. Gigan. Light fields in complex media: mesoscopic scattering meets wave control. Rev. Mod. Phys., 89, 015005(2017).
[11] K. Wang, W. Sun, C. T. Richie, B. K. Harvey, E. Betzig, N. Ji. Direct wavefront sensing for high-resolution
[12] S. Popoff, G. Lerosey, R. Carminati, M. Fink, A. Boccara, S. Gigan. Measuring the transmission matrix in optics: an approach to the study and control of light propagation in disordered media. Phys. Rev. Lett., 104, 100601(2010).
[13] A. Drémeau, A. Liutkus, D. Martina, O. Katz, C. Schülke, F. Krzakala, S. Gigan, L. Daudet. Reference-less measurement of the transmission matrix of a highly scattering material using a DMD and phase retrieval techniques. Opt. Express, 23, 11898-11911(2015).
[14] E. Tajahuerce, V. Durán, P. Clemente, E. Irles, F. Soldevila, P. Andrés, J. Lancis. Image transmission through dynamic scattering media by single-pixel photodetection. Opt. Express, 22, 16945-16955(2014).
[15] Y.-K. Xu, W.-T. Liu, E.-F. Zhang, Q. Li, H.-Y. Dai, P.-X. Chen. Is ghost imaging intrinsically more powerful against scattering?. Opt. Express, 23, 32993-33000(2015).
[16] Q. Fu, Y. Bai, X. Huang, S. Nan, P. Xie, X. Fu. Positive influence of the scattering medium on reflective ghost imaging. Photon. Res., 7, 1468-1472(2019).
[17] D. Lu, M. Liao, W. He, Z. Cai, X. Peng. Imaging dynamic objects hidden behind scattering medium by retrieving the point spread function. Proc. SPIE, 10834, 1083428(2018).
[18] H. He, X. Xie, Y. Liu, H. Liang, J. Zhou. Exploiting the point spread function for optical imaging through a scattering medium based on deconvolution method. J. Innov. Opt. Health Sci., 12, 1930005(2019).
[19] X. Xu, X. Xie, A. Thendiyammal, H. Zhuang, J. Xie, Y. Liu, J. Zhou, A. P. Mosk. Imaging of objects through a thin scattering layer using a spectrally and spatially separated reference. Opt. Express, 26, 15073-15083(2018).
[20] J. Bertolotti, E. G. Van Putten, C. Blum, A. Lagendijk, W. L. Vos, A. P. Mosk. Non-invasive imaging through opaque scattering layers. Nature, 491, 232-234(2012).
[21] O. Katz, P. Heidmann, M. Fink, S. Gigan. Non-invasive single-shot imaging through scattering layers and around corners via speckle correlations. Nat. Photonics, 8, 784-790(2014).
[22] A. Porat, E. R. Andresen, H. Rigneault, D. Oron, S. Gigan, O. Katz. Widefield lensless imaging through a fiber bundle via speckle correlations. Opt. Express, 24, 16835-16855(2016).
[23] J. R. Fienup. Phase retrieval algorithms: a comparison. Appl. Opt., 21, 2758-2769(1982).
[24] J. Chang, G. Wetzstein. Single-shot speckle correlation fluorescence microscopy in thick scattering tissue with image reconstruction priors. J. Biophoton., 11, e201700224(2018).
[25] P. Schniter, S. Rangan. Compressive phase retrieval via generalized approximate message passing. IEEE Trans. Signal Process., 63, 1043-1055(2014).
[26] Y. LeCun, Y. Bengio, G. Hinton. Deep learning. Nature, 521, 436-444(2015).
[27] I. Goodfellow, Y. Bengio, A. Courville, Y. Bengio. Deep Learning, 1(2016).
[28] G. Barbastathis, A. Ozcan, G. Situ. On the use of deep learning for computational imaging. Optica, 6, 921-943(2019).
[29] Y. Rivenson, Y. Zhang, H. Günaydın, D. Teng, A. Ozcan. Phase recovery and holographic image reconstruction using deep learning in neural networks. Light Sci. Appl., 7, 17141(2018).
[30] Y. Wu, Y. Rivenson, Y. Zhang, Z. Wei, H. Günaydin, X. Lin, A. Ozcan. Extended depth-of-field in holographic imaging using deep-learning-based autofocusing and phase recovery. Optica, 5, 704-710(2018).
[31] Z. Ren, Z. Xu, E. Y. Lam. Learning-based nonparametric autofocusing for digital holography. Optica, 5, 337-344(2018).
[32] Z. Ren, Z. Xu, E. Y. Lam. End-to-end deep learning framework for digital holographic reconstruction. Adv. Photon., 1, 016004(2019).
[33] T. Nguyen, Y. Xue, Y. Li, L. Tian, G. Nehmetallah. Deep learning approach for Fourier ptychography microscopy. Opt. Express, 26, 26470-26484(2018).
[34] A. Kappeler, S. Ghosh, J. Holloway, O. Cossairt, A. Katsaggelos. Ptychnet: CNN based Fourier ptychography. IEEE International Conference on Image Processing (ICIP), 1712-1716(2017).
[35] S. Jiang, K. Guo, J. Liao, G. Zheng. Solving Fourier ptychographic imaging problems via neural network modeling and TensorFlow. Biomed. Opt. Express, 9, 3306-3319(2018).
[36] Y. F. Cheng, M. Strachan, Z. Weiss, M. Deb, D. Carone, V. Ganapati. Illumination pattern design with deep learning for single-shot Fourier ptychographic microscopy. Opt. Express, 27, 644-656(2019).
[37] M. Lyu, W. Wang, H. Wang, H. Wang, G. Li, N. Chen, G. Situ. Deep-learning-based ghost imaging. Sci. Rep., 7, 17865(2017).
[38] Y. He, G. Wang, G. Dong, S. Zhu, H. Chen, A. Zhang, Z. Xu. Ghost imaging based on deep learning. Sci. Rep., 8, 6469(2018).
[39] H. Wang, Y. Rivenson, Y. Jin, Z. Wei, R. Gao, H. Günaydın, L. A. Bentolila, C. Kural, A. Ozcan. Deep learning enables cross-modality super-resolution in fluorescence microscopy. Nat. Methods, 16, 103-110(2019).
[40] E. Nehme, L. E. Weiss, T. Michaeli, Y. Shechtman. Deep-storm: super-resolution single-molecule microscopy by deep learning. Optica, 5, 458-464(2018).
[41] W. Ouyang, A. Aristov, M. Lelek, X. Hao, C. Zimmer. Deep learning massively accelerates super-resolution localization microscopy. Nat. Biotechnol., 36, 460-468(2018).
[42] C. Ling, C. Zhang, M. Wang, F. Meng, L. Du, X. Yuan. Fast structured illumination microscopy via deep learning. Photon. Res., 8, 1350-1359(2020).
[43] L. Fang, D. Cunefare, C. Wang, R. H. Guymer, S. Li, S. Farsiu. Automatic segmentation of nine retinal layer boundaries in OCT images of non-exudative AMD patients using deep learning and graph search. Biomed. Opt. Express, 8, 2732-2744(2017).
[44] L. Waller, L. Tian. Computational imaging: Machine learning for 3D microscopy. Nature, 523, 416-417(2015).
[45] T. C. Nguyen, V. Bui, G. Nehmetallah. Computational optical tomography using 3-D deep convolutional neural networks. Opt. Eng., 57, 041406(2018).
[46] A. Goy, K. Arthur, S. Li, G. Barbastathis. Low photon count phase retrieval using deep learning. Phys. Rev. Lett., 121, 243902(2018).
[47] C. Chen, Q. Chen, J. Xu, V. Koltun. Learning to see in the dark. IEEE Conference on Computer Vision and Pattern Recognition, 3291-3300(2018).
[48] S. Feng, Q. Chen, G. Gu, T. Tao, L. Zhang, Y. Hu, W. Yin, C. Zuo. Fringe pattern analysis using deep learning. Adv. Photon., 1, 025001(2019).
[49] K. Wang, Y. Li, Q. Kemao, J. Di, J. Zhao. One-step robust deep learning phase unwrapping. Opt. Express, 27, 15100-15115(2019).
[50] H. Yu, X. Chen, Z. Zhang, C. Zuo, Y. Zhang, D. Zheng, J. Han. Dynamic 3-D measurement based on fringe-to-fringe transformation using deep learning. Opt. Express, 28, 9405-9418(2020).
[51] H. Yu, D. Zheng, J. Fu, Y. Zhang, C. Zuo, J. Han. Deep learning-based fringe modulation-enhancing method for accurate fringe projection profilometry. Opt. Express, 28, 21692-21703(2020).
[52] S. Li, M. Deng, J. Lee, A. Sinha, G. Barbastathis. Imaging through glass diffusers using densely connected convolutional networks. Optica, 5, 803-813(2018).
[53] M. Lyu, H. Wang, G. Li, S. Zheng, G. Situ. Learning-based lensless imaging through optically thick scattering media. Adv. Photon., 1, 036002(2019).
[54] N. Borhani, E. Kakkava, C. Moser, D. Psaltis. Learning to see through multimode fibers. Optica, 5, 960-966(2018).
[55] E. Guo, S. Zhu, Y. Sun, L. Bai, C. Zuo, J. Han. Learning-based method to reconstruct complex targets through scattering medium beyond the memory effect. Opt. Express, 28, 2433-2446(2020).
[56] E. Guo, Y. Sun, S. Zhu, D. Zheng, C. Zuo, L. Bai, J. Han. Single-shot color object reconstruction through scattering medium based on neural network. Opt. Lasers Eng., 136, 106310(2020).
[57] Y. Li, Y. Xue, L. Tian. Deep speckle correlation: a deep learning approach toward scalable imaging through scattering media. Optica, 5, 1181-1190(2018).
[58] Y. Sun, J. Shi, L. Sun, J. Fan, G. Zeng. Image reconstruction through dynamic scattering media based on deep learning. Opt. Express, 27, 16032-16046(2019).
[59] M. Liao, S. Zheng, D. Lu, G. Situ, X. Peng. Real-time imaging through moving scattering layers via a two-step deep learning strategy. Proc. SPIE, 11351, 113510V(2020).
[60] Y. Li, S. Cheng, Y. Xue, L. Tian. Displacement-agnostic coherent imaging through scatter with an interpretable deep neural network. Opt. Express, 29, 2244-2257(2020).
[61] K. Goda, B. Jalali, C. Lei, G. Situ, P. Westbrook. AI boosts photonics and vice versa. APL Photon., 5, 070401(2020).
[62] S. Feng, C. Kane, P. A. Lee, A. D. Stone. Correlations and fluctuations of coherent wave transmission through disordered media. Phys. Rev. Lett., 61, 834-837(1988).
[63] I. Freund, M. Rosenbluh, S. Feng. Memory effects in propagation of optical waves through disordered media. Phys. Rev. Lett., 61, 2328-2331(1988).
[64] H. Liu, Z. Liu, M. Chen, S. Han, L. V. Wang. Physical picture of the optical memory effect. Photon. Res., 7, 1323-1330(2019).
[65] O. Ronneberger, P. Fischer, T. Brox. U-Net: convolutional networks for biomedical image segmentation. International Conference on Medical Image Computing and Computer-Assisted Intervention, 234-241(2015).
[66] Y. LeCun, C. Cortes, C. J. C. Burges. The MNIST database of handwritten digits.
[67] C. E. Thomaz. FEI face database.
[68] C. Guo, J. Liu, W. Li, T. Wu, L. Zhu, J. Wang, G. Wang, X. Shao. Imaging through scattering layers exceeding memory effect range by exploiting prior information. Opt. Commun., 434, 203-208(2019).
[69] D. Tang, S. K. Sahoo, V. Tran, C. Dang. Single-shot large field of view imaging with scattering media by spatial demultiplexing. Appl. Opt., 57, 7533-7538(2018).
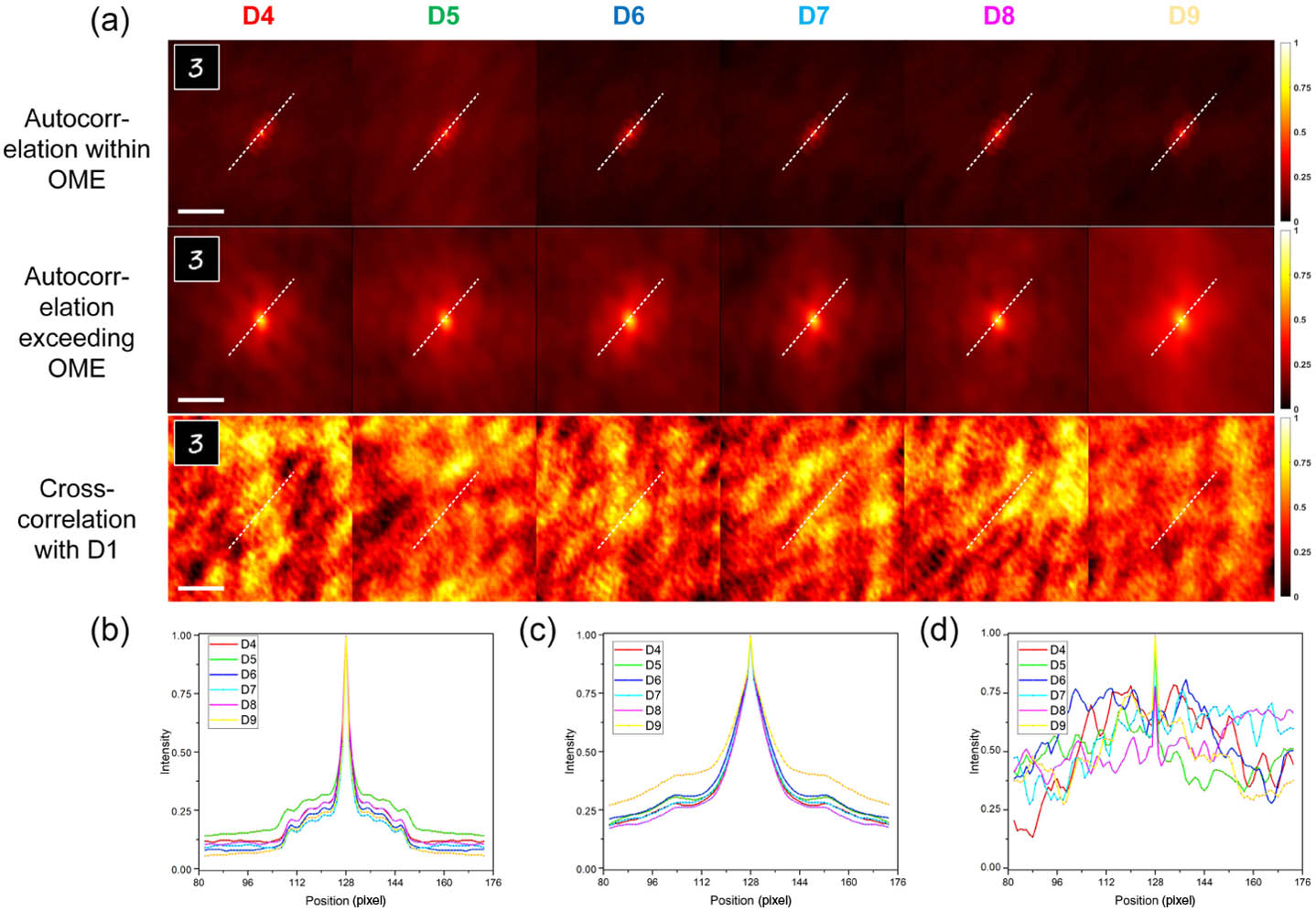
Set citation alerts for the article
Please enter your email address