Abstract
Histopathology relies upon the staining and sectioning of biological tissues, which can be laborious and may cause artifacts and distort tissues. We develop label-free volumetric imaging of thick-tissue slides, exploiting refractive index distributions as intrinsic imaging contrast. The present method systematically exploits label-free quantitative phase imaging techniques, volumetric reconstruction of intrinsic refractive index distributions in tissues, and numerical algorithms for the seamless stitching of multiple three-dimensional tomograms and for reducing scattering-induced image distortion. We demonstrated label-free volumetric imaging of thick tissues with the field of view of 2 mm × 1.75 mm × 0.2 mm with a spatial resolution of 170 nm × 170 nm × 1400 nm. The number of optical modes, calculated as the reconstructed volume divided by the size of the point spread function, was ~20 giga voxels. We have also demonstrated that different tumor types and a variety of precursor lesions and pathologies can be visualized with the present method.1 Introduction
Microscopic assessment of biopsied and resected tissues is central to understanding the underlying pathophysiology and clinical states of many patients. Because most microscopic specimens are translucent to visible light, conventional histological methods achieve image contrast using exogenous labels, such as haematoxylin and eosin (H&E) or immunohistochemical labeling.1–3 Although these procedures allow pathologists to easily identify cellular processes due to high specificity, they have several limitations, including heavy use of chemicals during sample preparation, which can induce artifacts and distort samples;4 long sample preparation time, which can delay pathological diagnosis; and labor-intensive histochemical processes.5 Importantly, the staining and visualization of thicker () tissues using traditional histology is limited by strong light absorption and scattering. Although recent advances in structured illumination microscopy6 and light-sheet microscopy7 have alleviated many of those disadvantages, label-free microscopy is still attractive because it might further reduce sample preparation.
A variety of diverse label-free microscopy methods have been employed to circumvent these challenges. Nonlinear microscopy methods are widely used8 because chemical specificities are retained without exogenous labels via characteristic vibrational modes9,10 or nonlinear susceptibilities11–14 of biomolecules. Nevertheless, the long dwell time to collect weak nonlinear signals impedes high-speed image scanning and rapid identification of pathological regions. Optical coherence tomography also shows promising results in histology,15 but imaging of subcellular features is limited due to speckle noise and spatial resolution. Quantitative phase imaging (QPI) is an alternative candidate for next-generation label-free histological methods, because it accelerates imaging speed by exploiting holographic imaging and using elastically scattering light information as an intrinsic contrast agent for biological specimens.16 Nevertheless, conventional QPI techniques provide only two-dimensional (2D) information of thin tissue samples.17,18
Optical diffraction tomography (ODT) is a three-dimensional (3D) QPI technique that achieves label-free volumetric histopathology. From transmitted-light holographic measurements at various angles, similar to x-ray computed tomography, ODT reconstructs the 3D refractive index (RI) distribution of biological cells.19,20 Because RI values depend on the number of intracellular biomolecules, including proteins and lipids, ODT allows label-free quantitative 3D morphological mapping of biological specimens and has been widely utilized to advance our understanding of the physiology of various live cells.21–25 However, several technical issues, such as a small holographic field retrieval field-of-view (FoV) and image degradation due to multiple light scattering, have hindered 3D RI-based histopathological analyses. When using this algorithm, tissue slices with thickness of up could be reconstructed with high contrast.
Sign up for Advanced Photonics TOC. Get the latest issue of Advanced Photonics delivered right to you!Sign up now
Here, we demonstrate multiscale label-free volumetric histopathology of thick tissue slides. We addressed the issues of limited FoV and image degradation by constructing a long-working-distance ODT optical setup and developing a robust multiscale ODT reconstruction and stitching algorithm, which consider optical aberration due to thick tissues. The experimental setup enabled mesoscopic imaging of various pathologic tissues over a millimeter-scale FoV with submicrometer resolution.
2 Results
2.1 Optical Setup
To image thick tissues, a custom ODT setup was built [Figs. 1(a) and 1(b)]. The system was based on a Mach–Zehnder interferometer, which was equipped with a long-working-distance objective lens for imaging thick tissues, an automated sample stage for raster-scanning, and a digital micromirror device (DMD; DLPLCR6500EVM, Texas Instrument) for illumination beam control. A blue continuous-wave laser (central , Cobolt Twist, Cobolt) was selected to avoid the H&E-staining absorption peak. A plane wave illuminated the samples with a specific illumination angle, which was systematically controlled by projecting time-multiplexed hologram patterns on the DMD.26 The intensity of the light projected on the sample by the condenser objective [LUCPLFLN40X, numerical aperture , Olympus] was of when using a camera exposure of 2 ms. The scattered field from the sample was collected by the objective lens (UPlanSAPO20X, , Olympus) and projected to the camera (LT425M-WOCG, Lumenera Inc.), where the scattered field from the sample interfered with a reference beam and generated a spatially modulated interferogram, from which both amplitude and phase images, as a function of illumination angles, were retrieved using Fourier analysis27 [Fig. 1(c)]. These multiple 2D field images were used to reconstruct a 3D RI tomogram of the sample using the Fourier diffraction theorem.28 The theoretical resolution of our imaging system, as defined by the maximum bandwidth of the optical transfer function, was 0.17 and in the lateral and axial directions, respectively.29
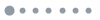
Figure 1.Design and mechanism of a custom optical diffraction tomography apparatus. (a) Close-up view of motorized stage; (b) schematic of the optical setup. BS, beam-splitter; L, lens; M, mirror; DMD, digital micromirror device; COND, condenser lens; OBJ, objective lens. (c) Optical diffraction tomography steps: first, a hologram is retrieved by the camera; the amplitude and phase of the field transmitted through the sample are retrieved from this hologram. Finally, the RI map of the sample is obtained. (d)–(g) Stitching algorithm steps: (d) overlapping regions of adjacent tiles are retrieved; (e) phase correlation algorithm output allows retrieval of the subpixel shift between the two overlapping regions; (f) mean square error between the overlapping regions after the subpixel shift correction; (g) small FoVs are raster scanned to form a big FoV; they are then stitched together using the relative position found in (e).
There are two main technical challenges in the reconstruction of wide and thick biological tissues: (1) alignment of multiple overlapping image tiles into a single stitched image and (2) improvement of image contrast against sample-induced aberrations. We addressed these issues computationally using correlation-based subpixel adjustments and digital field refocusing during RI reconstruction, respectively.
2.2 Evaluation of Optical Diffraction Tomography in Thick Samples
RI reconstruction using the Fourier diffraction theorem28 is based on a weak scattering approximation and, as such, does not hold well as samples become thicker. Recently, different methods mitigating multiple scattering effects on image reconstruction have been developed.30–32 However, these are computationally expansive and are still not suitable for a wide FoV imaging. However, it has also been shown that refocusing the field at every focal plane before reconstructing the RI can increase contrast in thick samples.33,34 This method is less efficient than the previous ones, but it is computationally less expansive and has been used in our algorithm.
Furthermore, we only refocused the field at a few focal planes and then reconstructed the RI around those virtual focal planes. This decreases the computational cost while conserving the quality improvement from the original refocusing method. To estimate the efficiency of our algorithm, the same data were reconstructed with and without digital refocusing. Then, the imaging quality was assessed by computing the RI contrast using its standard deviation. Indeed, sample-induced aberrations cause the fields to add up destructively, reducing both contrast and resolution. From Figs. 2(b) and 2(c), one can see that when digital refocusing was not used, the contrast rapidly decreases from the optical focal plane. However, when refocusing is used, contrast again increases at every virtual focal plane. The refocusing interval is chosen as a compromise between the computation speed and contrast lost between the virtual focal planes. In this experiment, a refocusing interval of was used. Even when using digital refocusing, due to multiple light scattering, the quality of the retrieved tomograms degraded as the tissue thickness was increased. Due to this, the tissue thickness is limited to when using the current setup and sample preparation. The maximum tissue thickness might be lower for fresh tissues, but it could be increased using longer wavelength light35 or ODT techniques using spatiotemporally incoherent illumination.36 The developments of the advanced reconstruction algorithm considering multiple light scattering would also further improve the imaging thickness.37,38
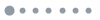
Figure 2.(a) Schematic of virtual focal planes and holographic refocusing; (b) RI standard deviation as a function of physical depth; (c) cross-sectional images of the reconstructed tomogram (left) with holographic refocusing and (right) without holographic refocusing. (d) Schematic of the stitching and focus finding steps. Scale bars are long.
A last limiting factor is that the illumination and detection size of single tiles is spatially limited. This limits the maximal axial FoV. Indeed, the volume that receives the detection at full numerical aperture and is illuminated by every illumination angle is limited to a conical region above and below the focal plane. Out of this region, the image will have both lower resolution and ghost images due to the circular symmetry of the discrete Fourier transform used in the reconstruction algorithm. It is important to overlap the tiles sufficiently so that these erroneous regions of the tomograms are removed when stitching. In all the experiments, an overlapping distance corresponding to 20% of the camera FoV was used.
2.3 Sample Preparation
It is crucial when imaging thick samples to use RI matching to limit multiple light scattering. Formalin-fixed paraffin-embedded tissue blocks from the normal small intestine, colon, and pancreas, and from the pancreatic neuroendocrine tumor and intraductal papillary neoplasm of the bile duct were selected. Tissue blocks were sliced with thickness, deparaffinized using three consecutive 10 min xylene bath, and finally mounted between two number zero coverslips using Permount mounting medium (Permount, , Fisher Chemical). Thin -thick sections were mounted on 1-mm-thick slide glass with Acrymount mounting medium.
2.4 Stitching Segmented Tomograms
Accurate image alignment between overlapping image tiles was achieved by correlation-based subpixel adjustment [Figs. 1(d)–1(g)]. During data acquisition, overlapping regions between adjacent 3D tiles were set to 20%, which guaranteed border artifact suppression during RI reconstruction [Fig. 1(d)]. The precise relative 3D position between overlapping regions was then determined with subpixel accuracy using a phase-correlation algorithm39 [Fig. 1(e)]. This position-correcting process enabled artifact reduction due to image misalignment, as evidenced by a mean squared errors analysis [Fig. 1(f)]. Next, the global positions of multiple image tiles were further adjusted using least square minimization.40 Minimization was weighted using the Pearson correlation coefficient of overlapping sections. This allowed quantifying similarity between overlapping regions and thus gave less importance to regions without tissue, where finding the relative position was more difficult. Weighting and the use of robust least square minimization avoided error propagation. Finally, images were placed at the obtained position and seamlessly blended together [Fig. 1(g)].
An important consideration when using stitching with ODT is that the illumination and detection size of single tiles is spatially limited. This limits the maximal axial FoV. Indeed, the volume that receives the detection at full numerical aperture and is illuminated by every illumination angle is limited to a conical region above and below the focal plane. Out of this region, the image will have both lower resolution and ghost images due to the circular symmetry of the discrete Fourier transform used in the reconstruction algorithm. It is, as such, important to overlap the tiles sufficiently so that these erroneous regions of the tomograms are removed when stitching. In all the experiments, an overlapping distance corresponding to 20% of the camera FoV was used.
2.5 Focus Finding in Tissues
The use of coherent light in ODT allows the focal plane to be adjusted numerically, avoiding blurring artifacts due to focus mismatching,41 which occurs in bright-field microscopy and other incoherent imaging methods. In our reconstruction algorithm, when imaging thin samples, the sample position is first automatically detected, and then an image containing only the most in-focus part of the tissue is generated. The optimal focus plane was found using the Tamura of the gradient focus criterion42 on the tomogram, which is a function that reaches its maximum when an image is well focused. The focal position was researched in this way every and interpolated in regions without samples. This method was also used in thick tissue to quickly find high contrast well-focused lateral cross-sections. Alternatively, the lateral shift between the fields taken from different illumination angles could also be used to find the focus position in a thin sample.43
2.6 Reconstruction of Refractive Index Distributions
Initially, reconstructed tomograms of thick tissues suffered from weak image contrast and distortion due to sample-induced aberrations. Considering that samples nearest the optical focal plane display sharper image contrast when reconstructed with the Rytov approximation, we exploited holographic refocusing to reconstruct 3D RI maps with higher image contrast33,34 [Figs. 2(a)–2(c)]. We digitally refocused the obtained light field images to virtual focal planes using a diffraction kernel based on Green’s function [Fig. 2(a)]. The refocusing interval is chosen as a compromise between the computation speed and contrast lost between the virtual focal planes. Here, we set the refocusing interval to , reconstructed volumetric RI tomograms thick with a voxel size of around each virtual focal plane, and combined the tomogram stack into a single tomogram. Qualitative comparison validated that the RI tomograms out of a focal plane could recover image contrast using refocusing-based RI reconstruction [Fig. 2(b)]. The RI distribution root-mean-squares also increased at the out-of-focus planes, quantitatively confirming that digital refocusing improved RI contrast of reconstructed thick tissue tomograms [Fig. 2(c)].
These algorithms were implemented on a graphical processing unit (GPU) to facilitate reconstruction speed. In particular, since phase unwrapping has to be performed at every refocusing step, phase unwrapping is a particularly computationally intensive part of the process. To improve performance, we implemented a custom version of Goldstein’s phase unwrapping algorithm,44 where the residue pairing was realized on the CPU, while residue detection, residue linking, rasterization, and phase unwrapping were performed on the GPU.
2.7 Demonstration in Thick and Thin Tissues
To validate the capacity of the present method for tissue slides volumetric histopathology, we first measured human small intestine tissue samples. For comparison purposes, we prepared two consecutive tissue slides, one using conventional H&E staining and the other unlabeled. The XY cross-sectional images of the unlabeled slide in the optical focal plane were consistent with the conventional in-focus bright-field images of the H&E-stained slide, demonstrating the high accuracy of this method [Figs. 3(a) and 3(b)]. Overall tissue anatomy and subcellular features were clearly seen in both the conventional H&E image and RI tomogram. To demonstrate applicability, various histopathology tissue slides were imaged [Figs. 3(c)–3(e)]. Unlabeled tissue samples from human organs (pancreas, small intestines, and large intestines) were cut at a thickness of and analyzed using the present method. The resulting FoVs were . The capability of volumetric histopathology can be seen in the high-resolution images of subparts at various axial foci [insets, Figs. 3(c)–3(e)]. Not only can subcellular features be accessed without labeling, but the 3D architecture of tissue structures can also be investigated.
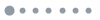
Figure 3.(a) Comparison of a normal colon tissue slice imaged with bright field and stained with H&E and (b) an unstained neighbor tissue slice imaged with ODT. In (a), the zoomed in image was taken with a 60× objective lens, while, in (b), the zoomed in image is a cropped version of the stitched image. (c)–(e) 3D wide field images of -thick tissue slices of (c) pancreas, (d) colon, and (e) small intestine. Zoomed in regions of interest are shown at different depths. Scale bars are long in (a), (b) and zoom in from (c)–(e). Scale bars are long in wide FoV images of (c)–(e).
2.8 Imaging Pathologic Tissue Slides
To validate further the present method for clinical applications, volumetric images of unlabeled thick pancreas tissues samples obtained from patients with pancreas neoplasms are shown in Fig. 4. The RI tomograms of tissues from patient #1 [Fig. 4(a)] show neoplastic epithelial cells with phenotypic neuroendocrine differentiation, characteristics of a pancreatic neuroendocrine tumor (PanNET). The back-to-back neoplastic cells with minimal stroma are easily appreciated, as is the uniformity in the shape of the round nuclei. All of these allow for the recognition of the diagnosis. For validation purposes, adjacent tissues were prepared and imaged using the conventional H&E staining method, which exhibit good agreement with the present method. The RI tomograms of the tissues from patient #2 show low-grade pancreatic intraepithelial neoplasia (PanIN) as well as normal pancreatic ducts, which show different cytoplasmic features between normal pancreatic ducts and low grade PanIN [Figs. 4(b) and 4(c)]. In the liver tissues from patient #3, the normal bile duct, intraductal papillary neoplasm of bile duct (IPNB), and cirrhotic nodule are imaged with the present method [Figs. 4(d)–4(f)]. Thus, different tumor types and a variety of precursor lesions and pathologies can be visualized.
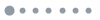
Figure 4.Volumetric histopathology of unlabeled -thick pancreas tissue samples from three individuals: (a) patient with a pancreatic neuroendocrine tumor; (b), (c) patient with pancreatic intraepithelial neoplasia (PanIN); and (d)–(f) patient with IPNB in the liver. The areas indicated with the boxes (i)–(vi) are shown at three different axial positions with magnification (from the second to the fourth rows). For comparison purposes, adjacent tissues were prepared in thin tissue slides with the conventional H&E staining method. (The fifth row, magnification.) Scale bars are long in wide FoV images and long in zoomed regions of interest.
3 Discussion and Conclusion
We present multiscale volumetric imaging of unlabeled tissue samples. We imaged millimeter-scale, unstained, -thick tissues at a subcellular 3D resolution, which enabled the visualization of individual cells and multicellular tissue architectures in a variety of tissues. By systematically stitching 3D RI tile data obtained with a long-working-distance ODT setup, we demonstrated an FoV of with a spatial resolution of , and, throughout this extended imaging volume, image degradation due to sample-induced aberration was significantly reduced using the holographic refocusing strategy. The present method can be understood as extremely high content imaging. The number of optical modes, calculated as the reconstructed volume divided by the size of the point spread function, was .
Considering the label-free volumetric imaging capability exhibited across a variety of tissue samples, this method could potentially be used for rapid cancer diagnosis during intraoperative pathology consultations or small biopsy samples. In addition, the present digital pathology approach has considerable advantages over conventional imaging methods, particularly in terms of measuring seamless multiscale volumetric images; conventional methods only generate one or a few focused plane images. In the current system, the time needed to measure sections () and produce volumetric reconstructions is 40 and 135 min, respectively. However, this can be further expedited by implementing a high-speed translational stage, a fast image sensor, and more powerful computing power.
To make the method more applicable, more research is needed on the imaging of fresh tissues and the histological interpretation of RI information. Because RI tomograms do not generate the same data as H&E staining, they would be utilized as complementary information. We envision that this generic approach could have far-reaching applications in histopathology and cytometry, possibly in conjunction with newly emerging machine learning methods for segmentation and cell-type classification.
References
[1] H. Suchannek. Beiträge zur feinern normalen Anatomie des menschlichen Geruchsorganes. Arch. mikroskopische Anat., 36, 375-403(1890).
[2] A. H. Coons, H. J. Creech, R. N. Jones. Immunological properties of an antibody containing a fluorescent group. Proc. Soc. Exp. Biol. Med., 47, 200-202(1941).
[3] M. Titford. The long history of hematoxylin. Biotech. Histochem., 80, 73-78(2005).
[4] J. I. Hamburger et al. Increasing the accuracy of fine-needle biopsy for thyroid nodules. Arch. Pathol. Lab. Med., 113, 1035-1041(1989).
[5] R. J. Buesa. Histology: a unique area of the medical laboratory. Ann. Diagn. Pathol., 11, 137-141(2007).
[6] M. Wang et al. Gigapixel surface imaging of radical prostatectomy specimens for comprehensive detection of cancer-positive surgical margins using structured illumination microscopy. Sci. Rep., 6, 27419(2016).
[7] A. K. Glaser et al. Light-sheet microscopy for slide-free non-destructive pathology of large clinical specimens. Nat. Biomed. Eng., 1, 0084(2017).
[8] H. Tu et al. Stain-free histopathology by programmable supercontinuum pulses. Nat. Photonics, 10, 534-540(2016).
[9] C. W. Freudiger et al. Label-free biomedical imaging with high sensitivity by stimulated Raman scattering microscopy. Science, 322, 1857-1861(2008).
[10] A. Zumbusch, G. R. Holtom, X. S. Xie. Three-dimensional vibrational imaging by coherent anti-Stokes Raman scattering. Phys. Rev. Lett., 82, 4142-4145(1999).
[11] D. Débarre et al. Imaging lipid bodies in cells and tissues using third-harmonic generation microscopy. Nat. Methods, 3, 47-53(2006).
[12] J. A. Squier et al. Third harmonic generation microscopy. Opt. Express, 3, 315-324(1998).
[13] D. Yelin, Y. Silberberg. Laser scanning third-harmonic-generation microscopy in biology. Opt. Express, 5, 169-175(1999).
[14] W. R. Zipfel et al. Live tissue intrinsic emission microscopy using multiphoton-excited native fluorescence and second harmonic generation. Proc. Natl. Acad. Sci. U. S. A., 100, 7075-7080(2003).
[15] O. Assayag et al. Large field, high resolution full-field optical coherence tomography: a pre-clinical study of human breast tissue and cancer assessment. Technol. Cancer Res. Treat., 13, 455-468(2014).
[16] Y. Park, C. Depeursinge, G. Popescu. Quantitative phase imaging in biomedicine. Nat. Photonics, 12, 578-589(2018).
[17] Z. Wang et al. Tissue refractive index as marker of disease. J. Biomed. Opt., 16, 116017(2011).
[18] M. Lee et al. Label-free optical quantification of structural alterations in Alzheimer’s disease. Sci. Rep., 6, 31034(2016).
[19] E. Wolf. Three-dimensional structure determination of semi-transparent objects from holographic data. Opt. Commun., 1, 153-156(1969).
[20] K. Kim et al. Optical diffraction tomography techniques for the study of cell pathophysiology. J. Biomed. Photonics Eng., 2, 020201(2016).
[21] Y. Kim et al. Profiling individual human red blood cells using common-path diffraction optical tomography. Sci. Rep., 4, 6659(2014).
[22] Y. Park et al. Refractive index maps and membrane dynamics of human red blood cells parasitized by Plasmodium falciparum. Proc. Natl. Acad. Sci. U.S.A., 105, 13730-13735(2008).
[23] S.-A. Yang et al. Measurements of morphological and biophysical alterations in individual neuron cells associated with early neurotoxic effects in Parkinson’s disease. Cytom. Part A, 91, 510-518(2017).
[24] G. Dardikman-Yoffe et al. High-resolution 4-D acquisition of freely swimming human sperm cells without staining. Sci. Adv., 6, eaay7619(2020).
[25] F. Merola et al. Tomographic flow cytometry by digital holography. Light Sci. Appl., 6, e16241(2017).
[26] K. Lee et al. Time-multiplexed structured illumination using a DMD for optical diffraction tomography. Opt. Lett., 42, 999-1002(2017).
[27] M. Takeda, H. Ina, S. Kobayashi. Fourier-transform method of fringe-pattern analysis for computer-based topography and interferometry. J. Opt. Soc. Am., 72, 156-160(1982).
[28] E. Wolf, M. Born. Scattering from inhomogeneous media. Principles of Optics: Electromagnetic Theory of Propagation, Interference and Diffraction of Light, 695-734(1999).
[29] C. Park, S. Shin, Y. Park. Generalized quantification of three-dimensional resolution in optical diffraction tomography using the projection of maximal spatial bandwidths. J. Opt. Soc. Am. A, 35, 1891-1898(2018).
[30] J. Lim et al. High-fidelity optical diffraction tomography of multiple scattering samples. Light Sci. Appl., 8, 82(2019).
[31] U. S. Kamilov et al. Learning approach to optical tomography. Optica, 2, 517-522(2015).
[32] S. Fan et al. Optical fiber refractive index profiling by iterative optical diffraction tomography. J. Lightwave Technol., 36, 5754-5763(2018).
[33] A. Goy et al. Imaging thick samples with optical tomography(2017).
[34] J. Kostencka et al. Holographic tomography with scanning of illumination: space-domain reconstruction for spatially invariant accuracy. Biomed. Opt. Express, 7, 4086-4101(2016).
[35] S. L. Jacques. Optical properties of biological tissues: a review. Phys. Med. Biol., 58, R37-R61(2013).
[36] T. H. Nguyen et al. Gradient light interference microscopy for 3D imaging of unlabeled specimens. Nat. Commun., 8, 210(2017).
[37] J. Lim et al. Beyond Born––Rytov limit for super-resolution optical diffraction tomography. Opt. Express, 25, 30445-30458(2017).
[38] D. Ryu et al. DeepRegularizer: rapid resolution enhancement of tomographic imaging using deep learning(2020).
[39] H. Foroosh, J. B. Zerubia, M. Berthod. Extension of phase correlation to subpixel registration. IEEE Trans. Image Process., 11, 188-200(2002).
[40] S. Preibisch, S. Saalfeld, P. Tomancak. Globally optimal stitching of tiled 3D microscopic image acquisitions. Bioinformatics, 25, 1463-1465(2009).
[41] P. Ferraro et al. Extended focused image in microscopy by digital holography. Opt. Express, 13, 6738-6749(2005).
[42] Y. Zhang et al. Edge sparsity criterion for robust holographic autofocusing. Opt. Lett., 42, 3824-3827(2017).
[43] L. Che et al. Exploiting a holographic polarization microscope for rapid autofocusing and 3D tracking. Biomed. Opt. Express, 11, 7150-7164(2020).
[44] D. C. Ghiglia, M. D. Pritt. Two-Dimensional Phase Unwrapping: Theory, Algorithms, and Software(1998).