Xingyu Wang, Tianyi Wu, Chen Dong, Haonan Zhu, Zhuodan Zhu, Shanghong Zhao, "Integrating deep learning to achieve phase compensation for free-space orbital-angular-momentum-encoded quantum key distribution under atmospheric turbulence," Photonics Res. 9, B9 (2021)

Search by keywords or author
- Photonics Research
- Vol. 9, Issue 2, B9 (2021)
![CNN structure is used to predict the phase distortion in atmospheric turbulence channel. BN, batch normalization; ReLU, rectified linear unit. We consider the encoder-decoder architecture of SegNet [48], since the prediction of the turbulent phase screen belongs to the pixel-by-pixel prediction tasks. The encoder part includes the convolutional layers, pooling layers, etc., where the three maxpool layers make the resolution of the feature map 8 times lower than that of the original image to perform nonlinear upsampling. In the decoder part, three deconvolutional layers are used for upsampling by a factor of 8, so as to keep the size of the original input image consistent. Note that the CNN can be changed according to the needs of specific prediction tasks.](/richHtml/prj/2021/9/2/020000B9/img_001.jpg)
Fig. 1. CNN structure is used to predict the phase distortion in atmospheric turbulence channel. BN, batch normalization; ReLU, rectified linear unit. We consider the encoder-decoder architecture of SegNet [48], since the prediction of the turbulent phase screen belongs to the pixel-by-pixel prediction tasks. The encoder part includes the convolutional layers, pooling layers, etc., where the three maxpool layers make the resolution of the feature map 8 times lower than that of the original image to perform nonlinear upsampling. In the decoder part, three deconvolutional layers are used for upsampling by a factor of 8, so as to keep the size of the original input image consistent. Note that the CNN can be changed according to the needs of specific prediction tasks.
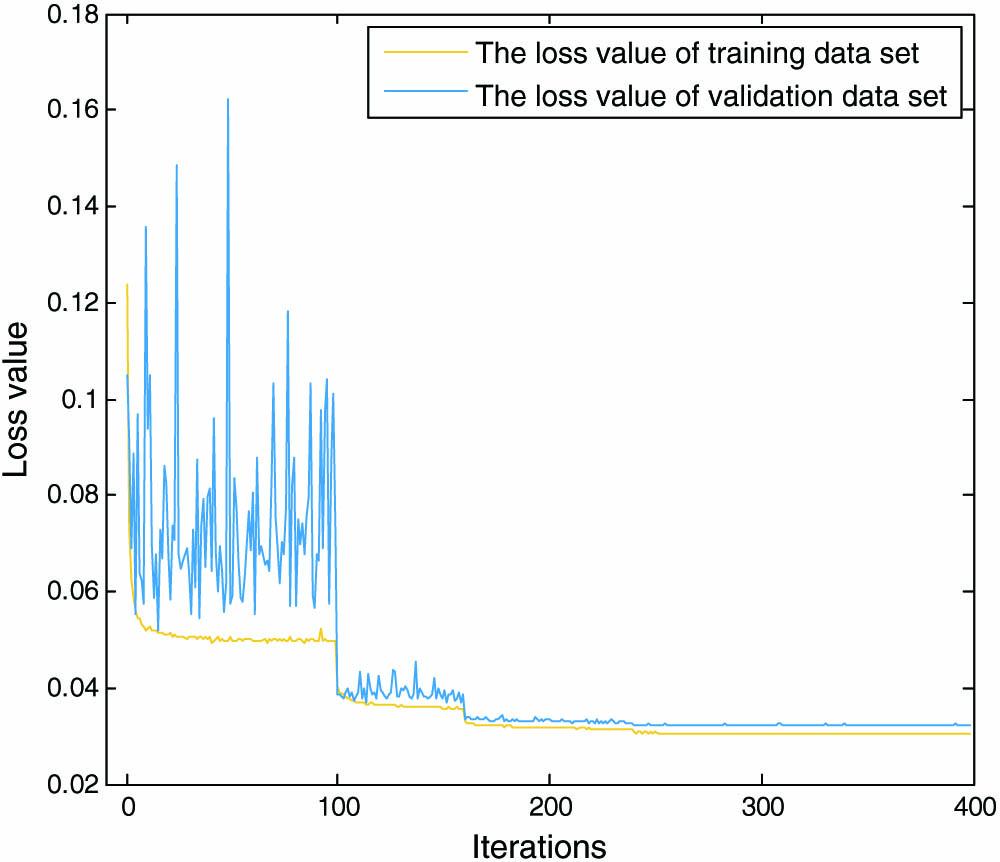
Fig. 2. Loss values of the validation data set and training data set against the iterations of the algorithm. Here we dynamically tune the learning rate at every 10 epochs. As the number of iterations increases, the learning rate is gradually reduced in the process of approaching the optimal solution, which shows drops of the loss value at 100 iterations.
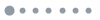
Fig. 3. Atmospheric turbulence phase graphs predicted by the CNN different training iterations. The five images from left to right represent the phase screens that are predicted by the validation data set at 10, 100, 200, 300, and 400 iterations, and the rightmost one is the one corresponding to the actual phase screen.
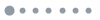
Fig. 4. Schematic diagram of an OAM-encoded QKD with decoy-state method. CW laser, continuous-wave laser; GPB, Gaussian probe beam; IM, intensity modulator; PBS, polarization beam splitter; SLM, spatial light modulator; ATT, attenuator; MR: mirror; CCD, charge-coupled device; SPD, single-photon detector. At Alice’s side, the signal pulses (a CW laser beam modulated by an IM) are separated to two parts by a PBS. The x -polarized GPB is modulated to produce a decoy state and encoded to load the spatially helical phase wavefront (or OAM) by an IM and SLM1, respectively. The y -polarized GPB is then combined with the encoded OAM signal pulses by another PBS and transmitted together through turbulence channel simulated by SLM2. Both the y -polarized GPB and the encoded OAM signal pulses will get phase distortion when transmitted through the simulated turbulence channel. The phase distortion of the y -polarized GPB is detected by a CCD, and the corresponding reverse phase screen will be produced by SLM3 to compensate for the phase distortion of the OAM-encoded signal pulses. After compensation, the OAM-encoded signal pulses will be classified by the OAM sorter and received by different SPDs.
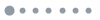
Fig. 5. Mode purity of the received OAM states with and without compensation.
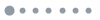
Fig. 6. Mode purity of the received OAM state in the different situations.
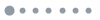
Fig. 7. Secret key rate performance of the OAM-encoded QKD versus propagation distance for different schemes. From top to bottom, the curves represent the secret key rates in the perfect phase compensation (red dotted line, χ = 1 ), the practical situation with the phase compensation feedback supported by the learned CNN (blue solid line, χ < 1 ), and the situation without phase compensation (green dashed line, χ = 0 ). Note that we fix the signal and decoy intensities to μ = 0.3 , v = 0.05 . Therefore, by scanning through the decoy-state intensities and probabilities, the key rate can be further optimized.
|
Table 1. List of Parameters Used in the Simulations
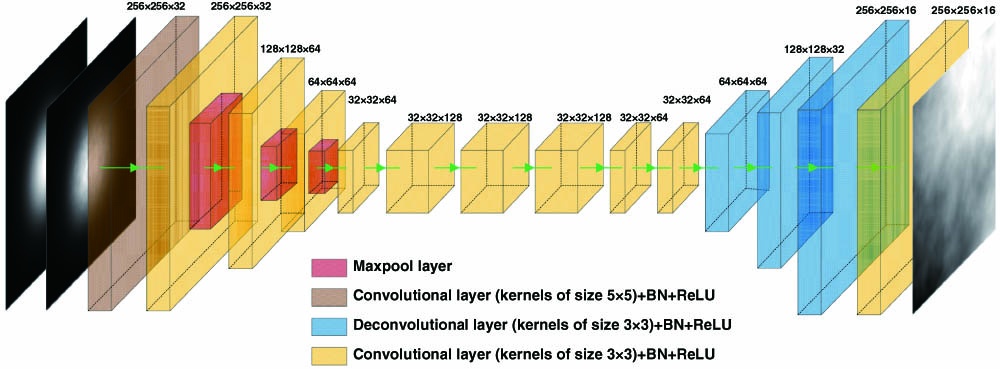
Set citation alerts for the article
Please enter your email address