Jia Sun, Dabo Guo, Tiantian Yang, Shitu Ma. Real-Time Object Detection Based on Improved YOLOv3 Network[J]. Laser & Optoelectronics Progress, 2020, 57(22): 221505

Search by keywords or author
- Laser & Optoelectronics Progress
- Vol. 57, Issue 22, 221505 (2020)
Abstract
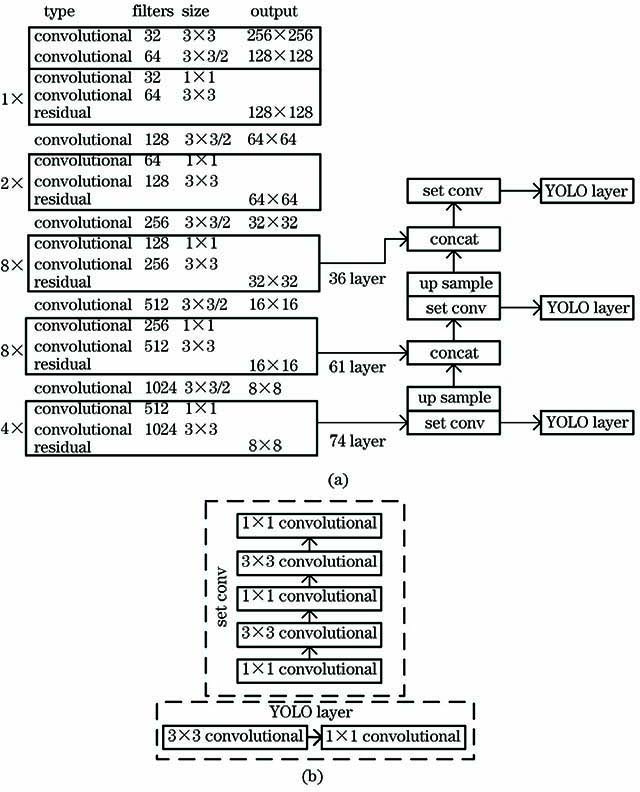
Set citation alerts for the article
Please enter your email address